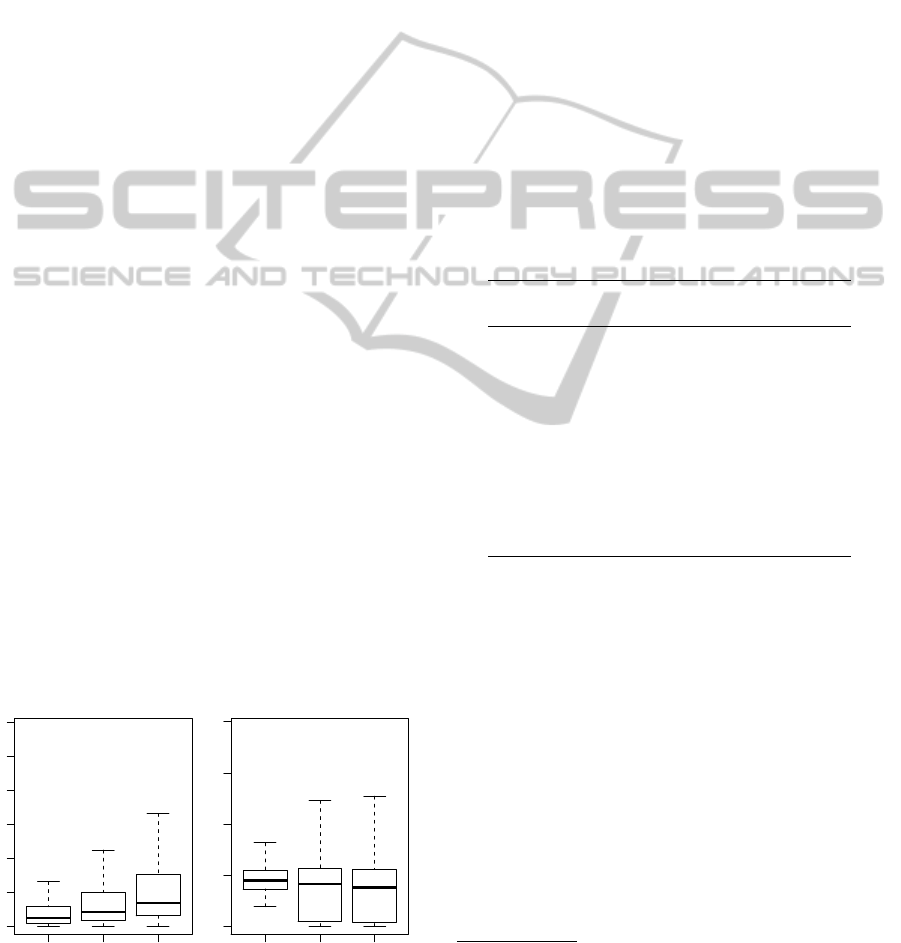
and we have pre-computed the neighborhood taking
only those ratings into account. Then, we evalu-
ate the algorithm considering all ratings up to Febru-
ary 1st, March 1st, and so on. For the evaluation,
we have considered two situations: one with neigh-
borhood pre-computation, where the previously com-
puted neighborhood (with ratings up to January 1st)
is used, and another where the neighborhood is com-
puted at recommendation time (and thus all ratings up
to the given month are considered). In either case, for
recommendation we consider all the ratings available
at that time. This way, we simulate an environment
where the neighborhood is computed once at the be-
ginning of the year, but the rating matrix is being up-
dated constantly. We have performed the evaluation
with 1, 000 randomly selected users.
First, we have studied how neighborhood pre-
computation can speed up recommendation time. For
our experiments, we have used a PC with a Intel Pen-
tium 4 CPU at 3.20 GHz and 256 MiB of RAM. Us-
ing an old machine is an approach commonly used for
efficiency evaluation in Information Retrieval (Badue
et al., 2007) when the dataset used is significantly
smaller than the amount of data in real applications.
Results are shown in Figure 1. As expected,
the usage of neighborhood pre-computation signif-
icantly reduces recommendation time. In average,
recommendation is computed two orders of magni-
tude faster, which is a very important achievement.
Moreover, with neighborhood pre-computation the
required time remains more or less constant among
months, even though the number of ratings increases.
On the other hand, with no pre-computation it signif-
icantly increases with the number of ratings. That is,
the neighborhood computation time is more affected
by the number of ratings than the final recommenda-
tion step, which makes sense because in that final step
only a few users (the neighbors) are actually consid-
ered.
●
●
●
●
●
●
●
●
●
●
●
●
●
●
●
●
●
●
●
●
●
●
●
●
●
●
●
●
●
●
●
●
●
●
●
●
●
●
●
●
●
●
●
●
●
●
●
●
●
●
●
●
●
●
●
●
●
●
●
●
●
●
●
●
●
●
●
●
●
●
●
●
●
●
●
●
●
●
●
●
●
●
●
●
●
●
●
●
●
●
●
●
●
●
●
●
●
●
●
●
●
●
●
●
●
●
●
●
●
●
●
●
●
●
●
●
●
●
●
●
Jany Jun Dec
0 20 40 60 80 100 120
●
●
●
●
●
●
●
●
●
●
●
●
●
●
●
●
●
●
●
●
●
●
●
●
●
●
●
●
●
Jan Jun Dec
0.0 0.1 0.2 0.3 0.4
With pre−computation
Recommendation time (s.)
Figure 1: Recommendation time (seconds) with and with-
out neighborhood pre-computation. Note the different scale
in each chart.
We have also evaluated the precision and recall of
the recommendations, in order to study the evolution
of the quality with the time elapsed after neighbor-
hood pre-computation. If the precision dropped very
fast, this technique would be not very useful, because
the pre-computation step would need to be done very
often. However, as seen in Table 1, this is not the
case. Both precision and recall remain similar with
and without pre-computation
1
, without statistical sig-
nificant differences between them. While updating
the rating matrix is very important (in order to recom-
mend new products, for example), dealing with an old
neighborhood seems to have almost no impact in rec-
ommendation quality. Of course, the actual threshold
where an outdated neighborhood begins to negatively
impact quality is domain-dependent. While a several
months old neighborhood is not a problem in the stud-
ied case, other domains might require a shorter neigh-
borhood update time.
Table 1: Precision@5 and Recall@5 with and without pre-
computation.
P@5 R@5
With Without With Without
Jan 1.28 1.28 0.13 0.13
Feb 0.99 0.90 0.12 0.08
Mar 1.16 1.39 0.13 0.15
Apr 0.98 1.21 0.12 0.17
May 0.72 0.76 0.07 0.08
Jun 0.75 0.81 0.09 0.12
Jul 1.03 0.65 0.46 0.12
Ago 0.12 0.39 0.03 0.05
Sep 0.26 0.32 0.08 0.27
Oct 0.22 0.21 0.14 0.12
3 CONCLUSIONS
In this paper we have evaluated the benefits of neigh-
borhood pre-computation. We have shown how this
technique can reduce the recommendation time of
k-Nearest Neighbors algorithms by two orders of
magnitude, without a significant impact in the rec-
ommendation list quality. These results show that
real applications can benefit from neighborhood pre-
computation techniques with no important drawback
in terms of precision. In the future, we plan to ex-
tend this research to further domains. We also plan to
study the impact on different metrics, and with differ-
ent update strategies.
1
Note that bad precision in the last months is related to
the evaluation methodology, as there are few relevant rat-
ings after that time. This is a well-known limitation of of-
fline evaluation (Cacheda et al., 2011).
KDIR2012-InternationalConferenceonKnowledgeDiscoveryandInformationRetrieval
334