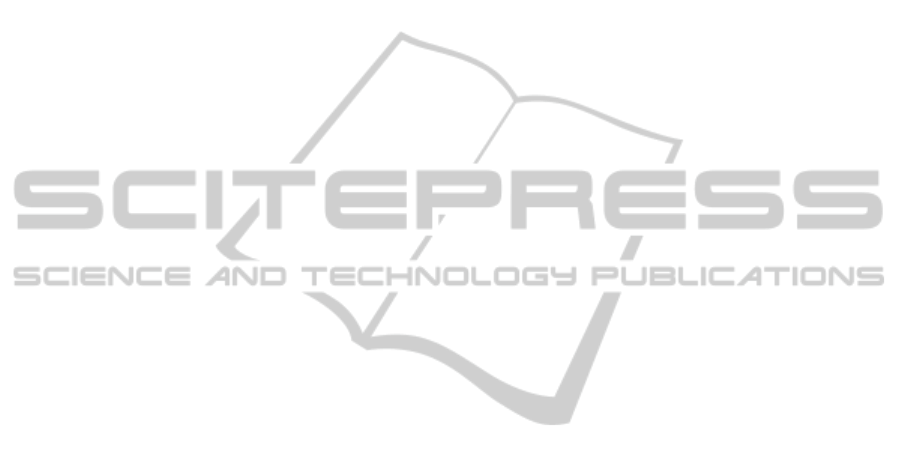
possible to improve the results in the future. Duo to
the frequency of admissions in the ICU, a more
comprehensive study may require at least one year
of continuous data acquisition.
7 CONCLUSIONS
The process of development of an IDSS which can
be autonomous and able to operate in real-time is a
big challenge. In this work, the most difficult task
has been the transformation of the data process, and
the process to obtain critical events and their ratios.
The results proved that it is possible to
implement an IDSS in critical health environments
minimizing the human intervention.
The greatest contribution of this work is the
automation of the entire KDD process, in particular,
the data transformation and the data mining
processes. INTCare become an autonomous system
and is able to, in a automatically and in real-time
way, predict the organ failure and outcome for the
next 24 hours for the patients admitted in ICU. The
DM engine operates autonomously. This engine runs
the models and makes the probability results
available through the INTCare system.
Experimental work has been conducted in order
to compare the sensibility and accuracy values
attained using online DM models with those
obtained in offline.
In the work has been considered data from a
limited number of patients. The obtained results
were very good and, in general, similar to the values
obtained in the past, using a semi-autonomous
process. The doctors can now access to patient data
anywhere and anytime, through the electronic
nursing record, and they can consult the probability
of organ failure and the outcome in an intuitive,
quick and easy way. A questionnaire has been
prepared in order to understand the level of
acceptance of the system. The answers provided by
the doctors and by the nurses were very satisfactory
and motivating.
Further work will include more data to optimize
the DM models. Complementarily, it will be
considered the development of an ensemble.
ACKNOWLEDGEMENTS
The authors would like to thank FCT (Foundation of
Science and Technology, Portugal) for the financial
support through the contract PTDC/EIA/72819/
2006. The work of Filipe Portela was supported by
the grant SFRH/BD/70156/2010 from FCT.
REFERENCES
Bellazzi, R., & Zupan, B., (2008). Predictive data mining
in clinical medicine: current issues and guidelines.
International journal of medical informatics, 77(2),
81-97.
Concepts, O. D. M., (2005). 11g Release 1 (11.1). Oracle
Corp, 2007.
Fayyad, U. M., Piatetsky-Shapiro, G., & Smyth, P. (1996).
From data mining to knowledge discovery: an
overview.
Gago, P., Santos, M. F., Silva, Á., Cortez, P., Neves, J., &
Gomes, L., (2006). INTCare: a knowledge discovery
based intelligent decision support system for intensive
care medicine. Journal of Decision Systems.
Han, J., & Kamber, M., (2006). Data mining: concepts
and techniques: Morgan Kaufmann.
Lyerla, F., LeRouge, C., Cooke, D. A., Turpin, D., &
Wilson, L., (2010). A Nursing Clinical Decision
Support System and potential predictors of Head-of-
Bed position for patients receiving Mechanical
Ventilation. American Journal of Critical Care, 19(1),
39-47.
Orwat, C., Graefe, A., & Faulwasser, T., (2008). Towards
pervasive computing in health care - A literature
review. [10.1186/1472-6947-8-26]. BMC Medical
Informatics and Decision Making, 8(1), 26.
Portela, F., Santos, M., Vilas-Boas, M., Rua, F., Silva, Á.,
& Neves, J., (2010). Real-time Intelligent decision
support in intensive medicine. Paper presented at the
KMIS 2010- International Conference on Knowledge
Management and Information Sharing.
Portela, F., Santos, M. F., Gago, P., Silva, Á., Rua, F.,
Abelha, A., et al., (2011). Enabling real-time
intelligent decision support in intensive care. Paper
presented at the 25th European Simulation and
Modelling Conference- ESM'2011, Guimarães,
Portugal.
Portela, F., Santos, M. F., Silva, Á., Machado, J., &
Abelha, A., (2011). Enabling a Pervasive approach
for Intelligent Decision Support in Critical Health
Care. Paper presented at the HCist 2011 –
International Workshop on Health and Social Care
Information Systems and Technologies.
Portela, F., Santos, M. F., & Vilas-Boas, M., (2012). A
Pervasive Approach to a Real-Time Intelligent
Decision Support System in Intensive Medicine. In
Springer (Ed.), Communications in Computer and
Information Science (Vol. 0272, pp. 14).
Santos, M. F., Portela, F., Vilas-Boas, M., Machado, J.,
Abelha, A., & Neves, J., (2011). INTCARE - Multi-
agent approach for real-time Intelligent Decision
Support in Intensive Medicine. Paper presented at the
3rd International Conference on Agents and Artificial
Intelligence (ICAART), Rome, Italy.
DataMiningPredictiveModelsforPervasiveIntelligentDecisionSupportinIntensiveCareMedicine
87