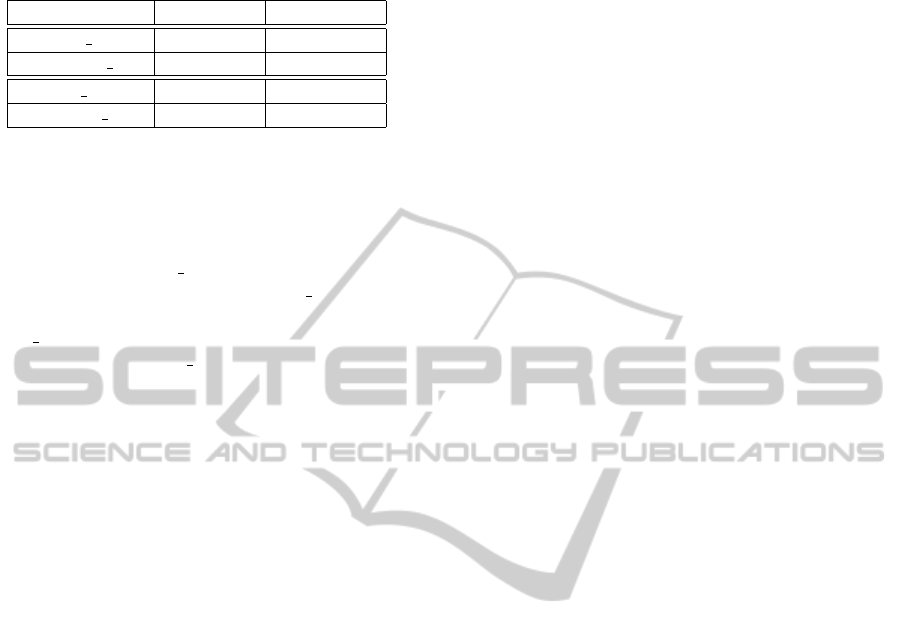
Table 1: Performance of DIKpEW compared to the baseline
mechanism.
NDCG@5 NDCG@10
Base Ita 0.484 0.437
DIKpEW Ita 0.558 0.614
Base Eng 0.485 0.576
DIKpEW Eng 0.523 0.686
returns only very relevant keyphrases then the NDCG
assumes the ideal value 1. Table 1 reports the 8 differ-
ent NDCG values computed for evaluating and com-
paring the accuracy of the top 5 and top 10 keyphrases
extracted by: (i) the baseline system from Web pages
written in Italian (Base Ita); (ii) our approach from
Web pages written in Italian (DIKpEW Ita); (iii) the
baseline system from Web pages written in English
(Base Eng); (iv) our approach from Web pages writ-
ten in English (DIKpEW Eng); .
According to the results showed in Table 1 our ap-
proach outperforms the baseline mechanism. More-
over, the accuracy of the results computed for the
Web pages in Italian are comparable to the accuracy
for the Web pages in English. This means that the
noise introduced by the translation in English does
not significantly lowers the accuracy of the results.
This can be justified in two ways: (i) the weight of
the keyphrase depends on a set of statistical features
which discard possible incorrect translation; (ii) the
Wikipedia feature allows us to throw out (or at least to
assign to lower positions) the bi-grams and tri-grams
which have not a clear meaning.
A final consideration concerns the NDCG met-
ric: it is important to emphasize that we exploited it
only for comparing our approach to a baseline refer-
ence. In fact, the choice of selecting only the top N
keyphrases (where N=5 or N=10) does not tackle the
possibility of working with pages with only 2 or 3
significant phrases. In this case, the NDCG@10 met-
ric, for example, would be much lower than 1. Future
work will also address this issue.
4 CONCLUSIONS
In this work we presented an approach which is aimed
at supporting the users of social tagging systems in
classifying Web pages. In particular,the presented ap-
proach identifies English n-grams from a Web docu-
ment for suggesting meaningful labels for the specific
resource. An experimental evaluation showed that
the proposed approach is plausible and future analysis
will investigate if the proposed approach can produce
better results for specific topics or specific set of Web
pages (blogs, newspapers, etc.).
The proposed approach can provide keyphrases
which appear already in the given document. Future
work will focus on overcoming this limitation by nav-
igating other knowledge sources such as Wikipedia
and Wordnet, producing in such a way meaningful
tags which are constituted by uni-grams, bi-grams, or
tri-grams not contained in the text, and that are the re-
sult of a domain reasoning activity. We also plan to
integrate our approach in collaborative and content-
based recommender systems following the ideas pro-
posed in (Ferrara and Tasso, 2011) and (Ferrara et al.,
2011).
REFERENCES
Barker, K. and Cornacchia, N. (2000). Using noun phrase
heads to extract document keyphrases. In Proceedings
of the 13th Biennial Conference of the Canadian So-
ciety on Computational Studies of Intelligence, pages
40–52, London, UK. Springer-Verlag.
Ferrara, F., Pudota, N., and Tasso, C. (2011). A keyphrase-
based paper recommender system. In Agosti, M., Es-
posito, F., Meghini, C., and Orio, N., editors, Digi-
tal Libraries and Archives, volume 249 of Communi-
cations in Computer and Information Science, pages
14–25. Springer Berlin Heidelberg.
Ferrara, F. and Tasso, C. (2011). Extracting and exploiting
topics of interests from social tagging systems. In Pro-
ceedings of the International Conference on Adaptive
and Intelligent Systems, ICAIS’11, pages 285–296,
Berlin, Heidelberg. Springer-Verlag.
Hulth, A. (2003). Improved automatic keyword extraction
given more linguistic knowledge. In Proceedings of
the 2003 conference on Empirical methods in natu-
ral language processing, pages 216–223, Morristown,
NJ, USA. Association for Computational Linguistics.
Hulth, A. and Megyesi, B. B. (2006). A study on automat-
ically extracted keywords in text categorization. In
ACL-44: Proc. of the 21st Int. Conf. on Computational
Linguistics and the 44th annual meeting of the Associ-
ation for Computational Linguistics, pages 537–544,
Morristown, NJ, USA. ACL.
Porter, M. F. (1997). An algorithm for suffix stripping.
Readings in information retrieval, pages 313–316.
Pudota, N., Dattolo, A., Baruzzo, A., Ferrara, F., and Tasso,
C. (2010). Automatic keyphrase extraction and on-
tology mining for content-based tag recommendation.
International Journal of Intelligent Systems, Special
Issue: New Trends for Ontology-Based Knowledge
Discovery, 25:1158–1186.
Turney, P. (1999). Learning to extract keyphrases from
text. Technical Report ERB-1057, National Research
Council, Institute for Information Technology.
Turney, P. D. (2000). Learning algorithms for keyphrase
extraction. Information Retrieval, 2(4):303–336.
AKeyphraseExtractionApproachforSocialTaggingSystems
365