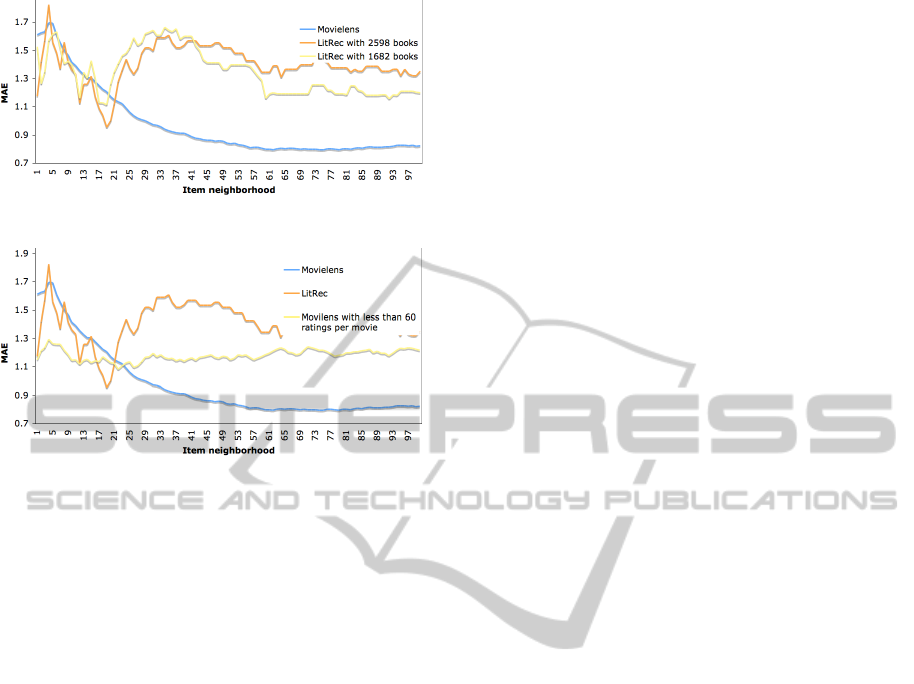
Figure 6: MAE using LitRec with the 1682 books.
Figure 7: MAE using Movielens including only movies
with fewer ratings.
5 CONCLUSIONS
Book recommendation is different from movie rec-
ommendation. Data sets are sparser making the CF
task harder. This may be due to the fact that books are
published in different languages while movies only
have the original. For example, a Portuguese person
who wants to rate the book “The Lord of the Rings”
will most likely be rating the Portuguese translations,
but if this person wants to rate the movie, he/she will
be rating only the original version of the movie.
In this paper we present a comparative study be-
tween Movielens and LitRec. Movielens has been
used in numerous studies and is considered by the re-
search community to be a well formed data set. Nev-
ertheless, book recommendation has specific recom-
mendation problems that are not present in Movie-
lens. Despite Movielens’ qualities as a data set, it
does not fit in all recommendation studies.
As was observed in the described experiments, al-
though a CF approach has acceptable performance
when using Movielens, even when the number of rat-
ings per item is reduced, the same does not happen
with LitRec due to rating distribution by user and
by item. This suggests that other approaches should
be tried to make book recommendation, e.g., using
hybrid set-ups (CF + content-based filtering) or us-
ing only content-based filtering. LitRec has the ad-
vantage of containing several features (book author,
genre, category, read, and rating date) and book con-
tent. Item content is always hard do get due to copy-
right restrictions.
Globally, LitRec performance is worse then
Movielens performance in a CF set-up, even when
items with the highest number of ratings were with-
drawn from the data set. This confirms the conclu-
sions achieved in previous works with LitRec that
other book features are important to improve book
recommendations accuracy (Vaz et al., 2012). Rec-
ommendation results, using LitRec, can take advan-
tage from hybrid recommendation set-ups.
Using Project Gutenberg documents can pose a
problem, because books are not recent. Despite the
fact that books like “Romeo and Juliet” and “Sense
and Sensibility” are always read, because they are
classics, results can be biased towards users with
given type of preferences. To generalize conclusions
further analysis of results must be conducted. Never-
theless, LitRec can be used to study the literary book
recommendation problem.
ACKNOWLEDGEMENTS
This work was supported by national funds through
FCT - Fundac¸
˜
ao para a Ci
ˆ
encia e a Tecnologia, under
project PEst-OE/EEI/LA0021/2011.
REFERENCES
Bellegarda, J. R. and Juang, B. H. (2006). Latent Seman-
tic Mapping: Principles And Applications (Synthesis
Lectures on Speech and Audio Processing). Morgan
& Claypool Publishers.
Blei, D. M., Ng, A. Y., and Jordan, M. I. (2003). La-
tent dirichlet allocation. J. Mach. Learn. Res., 3:993–
1022.
Celma, O. (2010). Music Recommendation and Discovery:
The Long Tail, Long Fail, and Long Play in the Digital
Music Space. Springer Publishing Company, Incorpo-
rated, 1st edition.
Goldberg, K., Roeder, T., Gupta, D., and Perkins, C. (2001).
Eigentaste: A constant time collaborative filtering al-
gorithm. Inf. Retr., 4(2):133–151.
Vaz, P. C., Martins de Matos, D., Martins, B., and Calado, P.
(2012). Improving a hybrid literary book recommen-
dation system through author ranking. In Proceedings
of the 12th ACM/IEEE-CS joint conference on Digital
Libraries, JCDL ’12, pages 387–388, New York, NY,
USA. ACM.
Ziegler, C.-N., McNee, S. M., Konstan, J. A., and Lausen,
G. (2005). Improving recommendation lists through
topic diversification. In Proceedings of the 14th inter-
national conference on World Wide Web, WWW ’05,
pages 22–32, New York, NY, USA. ACM.
LitRecvs.Movielens-AComparativeStudy
373