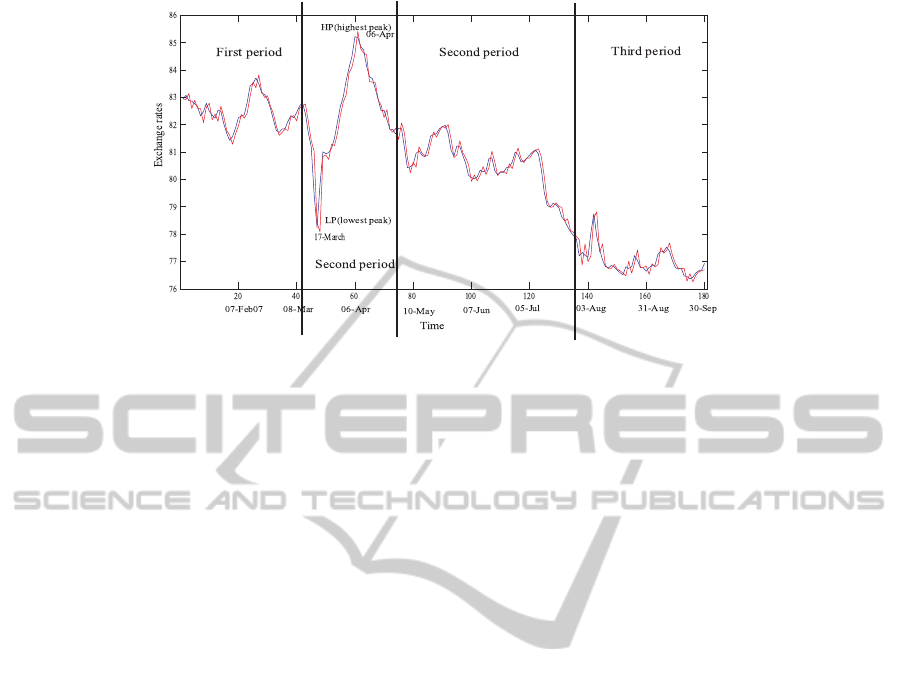
Figure 2: Training data of dollar-yen exchange rates during 2011.
tion coefficient by the contradiction was higher than
that by the conventional two methods.
Then, we computed quantization and topographic
errors for the training data. The quantization errors
decreased gradually and reached the final point of
0.437 when the spread parameter β was increased
from one to 20. By the conventional SOM, the quan-
tizatoin error was 0.505. The topographic error in-
creased from zero ((β = 1) to the maximum value
of 0.206 (β = 10). Then, the topographic error de-
creased gradually and reached the lowest point of
0.039. The topographic error by the SOM was 0.211.
Finally, mutual information between input patterns
and competitive units increased gradually when the
parameter β was increased. We could see that the
correlation coefficient between information and MSE
was -0.947. When information increased, the MSE
between outputs and targets for the testing data de-
creased. The correlation coefficient between infor-
mation and the coefficient coefficient between outputs
and targets was 0.874. When mutual information be-
tween input patterns and competitive units increased,
the correlation coefficient between outputs and targets
increased. We could also see that the correlation co-
efficient between information and quantization errors
was -0.992, meaning that quantization errors decease
when mutual information or organization increased.
This means that mutual information increase or in-
crease in organization in networks is closely related
to prediction and visualization performance except to-
pographic errors.
3.1.2 Visual Performance
Figure 3 shows the U-matrices by SOM and our
method. Figure 3 (a) shows the U-matrix by the con-
ventional SOM where we could not see clear class
boundaries. On the other hand, when the parame-
ter β was three in Figure 3(b), one straight bound-
ary in brown could be detected in the middle of
the U-matrix. When the parameter was increased to
five in Figure 3(c), the straight boundary deteriorated
slightly on the right hand side of the line. When the
parameter was further increase from 10 in Figure 3(d)
to 20 in Figure 3(f), class boundaries in warmer colors
became more complicated. Figures 4(a) and (b) show
the U-matrices and the corresponding labels by the
conventional SOM (b) and by the contradiction reso-
lution (a) when the network size was 10 by 5 and the
parameter β was ten. We can infer from these figures
that the entire period was divided into three periods,
namely, first, second and third period. In addition, the
highest and lowest peaks were separately treated as
shown in Figure 2(a). On the other hand, by the con-
ventional SOM in Figure 2(b), class boundaries on the
U-matrix and the labels were weaker.
3.2 Discussion
We here discuss visual and prediction performance
with some remarks on the possibility of our method.
First, our method could be applied to the self-
organizing maps to improve visualization perfor-
mance. In self-organizing maps, neurons are treated
equally, having no individual characteristics. The
main objective is to make neurons as similar as possi-
ble to each other. The self-organizing maps’ property
of cooperation has the effect to weaken class bound-
aries. Thus, many methods on the visualization of
SOM knowledge have been accumulated (Sammon,
1969), (Ultsch and Siemon, 1990), (Ultsch, 2003),
(Vesanto, 1999), (Kaski et al., 1998), (Yin, 2002), (Su
and Chang, 2001), (Xu et al., 2010), to cite a few. Our
contradiction resolution, as shown in the experimen-
IJCCI2012-InternationalJointConferenceonComputationalIntelligence
532