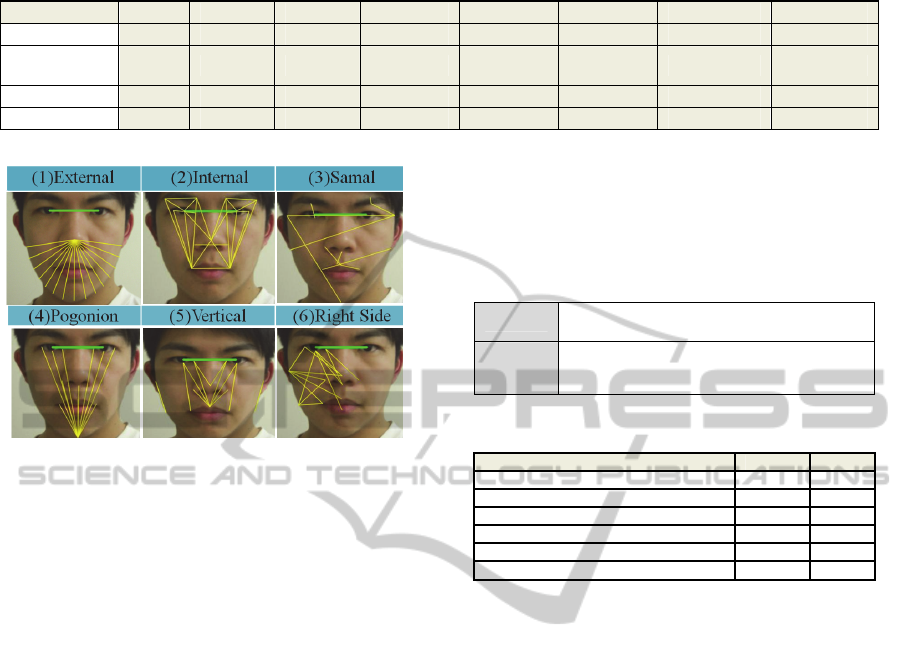
Table 1: Comparison of individual feature and classification.
Samal Internal External Pogonion Vertical Right Side Hair texture Hair color
SVM 67.85% 65.05% 66.70% 65.85% 62.85% 65.40% 80.10% 72.65%
Modest
AdaBoost
65.30% 62.10% 61.35% 65.15% 62.00% 64.65% 79.25% 70.05%
LogitBoost 64.60% 60.70% 60.65% 64.75% 61.75% 64.30% 78.30% 66.35%
Real AdaBoost 64.65% 60.30% 59.55% 65.00% 61.45% 64.10% 78.55% 66.85%
Figure 9: Geometrical feature extraction (thick line for
normalization).
3 EXPERIMENTAL RESULTS
3.1 Database
Related to face recognition scope, FERET database
is an image database extensively used. There are
14,126 pictures in the database, including 1,199
persons and about 2,722 images. We choose 2,000
images (male and female have 1,000 images,
respectively) to test colorful face images.
3.2 Experimental Results
At first, we compare all the classifiers and individual
features as shown in Table 1. For all listed
classifiers, SVM has the best performance than the
others. And if focusing on individual feature, the
result shows that hair features get higher correct
classification rate than geometry features, we can
consider it as strong features. But for individual
feature, there is still betterment.
Moreover, For the purpose of getting higher
correct classification rate, we had tested many sets
of feature combination. I just list some well
performed results as shown in Table 2. For facial
geometry gender classification, combined with
Samal and external feature it has the best
performance. From this, we can understand that it is
not always the case that the more geometry features
selected, the better performance it will be. Still
more, if it can combine strong feature of texture
detection, the total correct recognition rate can be
higher up to 87.15%.
Table 2: Performance of feature combination.
Texture
(1) complex background, (2) wrinkle and pores
(3) susceptibility to light.
Color
(1) darkness background, (2) region selected
(3) the same color as skin, (4) susceptibility to
light.
Table 3: Performance of combined features.
Feature Combination Features Rate
Internal + External
39 69.00%
Samal+ External
25 70.10%
Pogonion + External
29 69.85%
Samal + External + Hair color
29 79.55%
Samal + External + Hair texture
29 87.15%
Samal + External + Hair texture and color
33 86.55%
3.3 Discussion
There are more changing factors of color features
than the texture features, so it is easy to know the
poor results that should be. The normal color and
texture influence factor are shown as Table 3. As
you can see, even system can achieve the best
performance from simulation results, texture
features is not always better than color features for
long hair detection, even though texture features can
get the best recognition rate in experiments. The
reason is that FERET has many pictures in simple
background, and just changes the influence of light.
In practice, we should choose the features
combination methods that rely on the changes of
back ground or to eliminate background.
4 CONCLUSIONS
In this paper, we construct a fast and low complex
gender classification system. Our experimental
results show the importance of hair texture and the
most appropriate geometry characteristics of
matching for gender classification. We can still find
the importance of texture features, because of color
Component-basedGenderClassificationbasedonHairandFacialGeometryFeatures
629