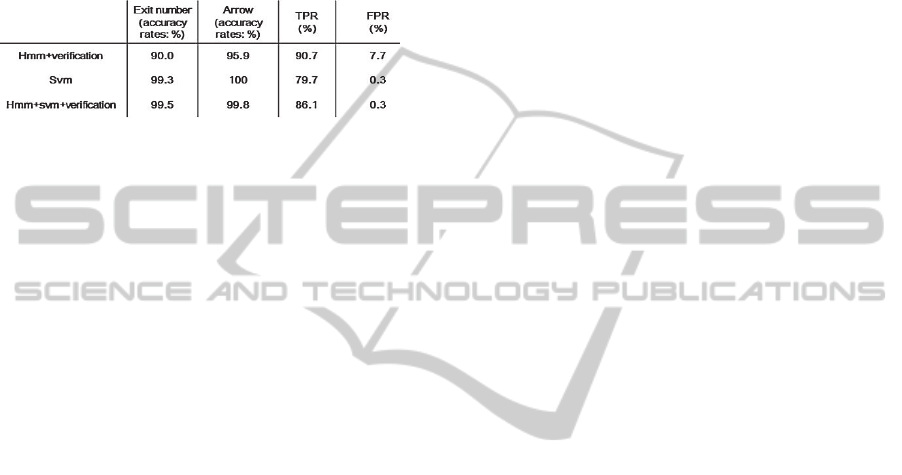
Finally, we compared three different
combinations: our proposal system and our novel
feature vector with HMM and other feature vector
with SVM. Also, we tested these combinations with
verification techniques as shown in table 2. As a
result, HMM+SVM with a verification technique is
better than the others.
Table 2: Comparison of the different combinations.
4 CONCLUSIONS
We have proposed a sign classification system,
focusing on 16 classes of exit number and arrow
signs. The main contribution of this paper is that we
suggest two methods: a novel feature extraction
algorithm and a verification technique. The main
advantage of our feature extraction algorithm is that it
is robust to various types and styles of signs. Also, a
false positive rate of 0.3% has demonstrated that
combining the verification technique is a reliable
method for discriminating sign regions from non-sign
regions.
However, some improvements remain as for the
system to be applied in the subway station. First, it is
necessary to develop a sign tracking algorithm to
verify the sign detection results. This sign tracking
algorithm would be a help improve TPR. Secondly,
the system must be operated in real time. Currently, in
our research, the processing time of our system is
about 5-15 fps dependent on how many signs are
detected. Thus, these improvements will be the future
work.
ACKNOWLEDGEMENTS
This research was supported by the Converging
Research Center Program funded by the Ministry of
Education, Science and Technology (No.
2011K000655).
REFERENCES
Ahmed, M., Ward, R., 2002. “A Rotation Invariant Rule-
Based Thinning Algorithm for Character Recognition,”
IEEE Transactions on Pattern Analysis and Machine
Intelligence, vol. 24, pp. 1672-1678.
Chang, C. C., Lin, C. J, 2001. “LIBSVM: a library for
support vector machines,” ACM Transactions on
Intelligent System and Technology, 2:27:1—27:27.
Chen, X., Yuille, A. L., 2004. “Detecting and reading text
in antural scenes,” Computer Vision and Pattern
Recognition (CVPR), vol. 2, pp. 366-373.
Froba, B., Ernst, A., 2004. “Face Detection with the
Modified Census Transform,” IEEE Conf. on
Automatic Face and Gesture Recognition, pp.91-96.
Kim, J. E., Yoon, H. S., 2011. “Graph Matching Method
for Character Recognition in Natural Scene Images,”
Conf. on Intelligent Engineering Systems (INES), pp.
347-350.
Liu, C. L., 2008. “Handwritten Chinese character
recognition: effects of shape normalization and feature
extraction,” Lecture Notes in Computer Science, vol.
4768/2008, pp.104-128.
Niblack, W., 1986. “An Introduction to Image
Processing,” Prentice-Hall, Englewood Cliffs, pp. 115-
116.
Otsu, N., 1979. “A threshoild selection method from gray-
level histogram,” IEEE Trans. Syst. Man Cybern., vol.
9, pp. 62-66.
Rabiner, L. R., 1989. “A tutorial on hidden Markov
models and selected applications in speech
recognition,” Proc. of the IEEE, vol.77, pp. 257-286.
Van, B. L., Garcia-Salicetti, S., Dorizzi, B., 2004. “Fusion
of HMM’s likelihood and Viterbi path for on-line
signature verification,” Proc. of Lecture Notes in
Computer Science, vol. 3072. Berlin, Germany:
Springer-Verlag, pp. 16-22.
Wu, T. F., Lin, C. J., Weng, R. C., 2004. “Probability
Estimates for Multi-class Classification by Pairwise
Coupling,” The Journal of Machine Learning
Research, vol. 5, pp. 975-1005.
Yoon, Y. W., Ban, K. D., Yoon, H. S., Kim, J. H., 2011.
“Blob Extraction based Character Segmentation
Method for Automatic License Plate Recognition
System,” Conf. on Systems, Man, and Cybernetics
(SMC), pp. 2192-2196.
IJCCI2012-InternationalJointConferenceonComputationalIntelligence
634