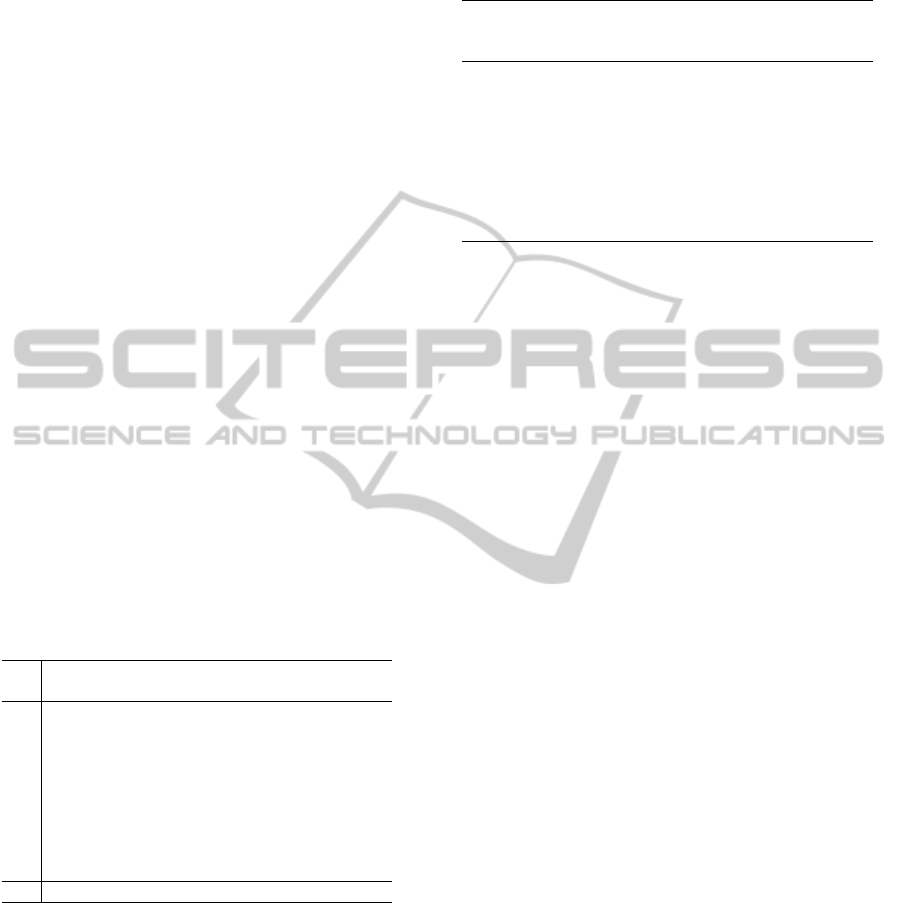
3 PROVOKING PREFERENCES
In order to make an ANN learn the PA the first step
is to find a suitable encoding for the specified rela-
tions and an architecture of the ANN for the consid-
ered kind of problem. Since the problems considered
here are 3-term problems, an architecture with two
nodes in the input layer were used for classifying pos-
sible relations. For the encoding of the premises, −1
was used for the relation <, 0 for =, and 1 for >. For
this kind of problems it is possible, that all three rela-
tions hold between two points. Therefor, three nodes
within the output layer were used. Respectively one
for each possible relation. If a relation holds for given
premises, the corresponding output node returns 1,
else it returns 0. For example, the premises [−1, 0]
with the target output [1, 0, 0] is a suitable pattern for
training the ANN. It represents the three-term-series
problem with the premises a<b, b=c and the solution
a<c. Furthermore, a hidden layer was used and the
number of nodes iteratively increased to find a suc-
cessful architecture for the given problem. For train-
ing the ANN, backpropagation was used as learning
algorithm with 1000 training iterations, a learning rate
of .3 and a momentum factor of .1. The tangens hy-
perbolicus was used as sigmoid activation function.
As depicted in Table 3, a suitable architecture requires
six nodes within the hidden layer. Since the ANN
Table 3: Rounded results on training different ANN archi-
tectures for PA. (hn = number of hidden nodes).
Prem 1 hn 2 hn 3 hn 4 hn 5 hn 6 hn 7 hn
p
1
p
2
<, =, ><, =, ><, =, ><, =, ><, =, ><, =, ><, =, >
-1 -1 1,0,1 1,0,0 1,-1,-1 1,0,0 1,1,0 1,0,0 1,0,0
-1 0 1,0,1 1,0,0 1,1,0 1,0,0 1,1,0 1,0,0 1,0,0
-1 1 1,0,1 1,0,1 1,1,1 1,1,1 1,1,1 1,1,1 1,1,1
0 -1 1,0,1 1,0,0 1,0,0 1,0,0 1,1,0 1,0,0 1,0,0
0 0 1,0,1 1,0,1 1,1,0 1,1,1 0,1,0 0,1,0 0,1,0
0 1 0,0,1 0,0,1 0,0,1 0,0,1 0,0,1 0,0,1 0,0,1
1 -1 1,0,1 1,0,1 1,1,1 1,1,1 1,1,1 1,1,1 1,1,1
1 0 0,0,1 0,0,1 0,0,1 0,0,1 0,0,1 0,0,1 0,0,1
1 1 0,0,0 0,0,1 0,0,1 0,0,1 0,0,1 0,0,1 0,0,1
Errors 3.685 1.980 1.260 0.766 1.503 0.020 0.086
was trained on the complete set of correct patterns of
three-term-series problems of PA, it is some kind of
over-fitted. However, in this case this does not matter,
because the ANN is only used to provide real-valued
outcomes in the complete process to determine possi-
ble sources for preferences. For this purpose, the tar-
get values will be varied from integers to real-values
to reproduce preferences in PA reasoning. The re-
sult shows that PA is learnable by a quite small ANN
with little effort and suggests a good architecture of
an ANN for this kind of task. Human reasoning per-
Table 4: Mapping of C D relations to ANN input. Re-
sults on training the ANN with the varied patterns [[-1,
1],[0.9,1,0.9]] and [[ 1,-1],[0.9,1,0.9]] of PA.
C D PA ANN Learned Target
Rel. Dimension Input Values
x y x y
SW < < -1 -1 0.990 -0.360 0.107
W < = -1 0 0.999 -0.410 0.092
NW < > -1 1 0.902 0.970 0.897
S = < 0 -1 0.987 -0.327 0.040
EQ = = 0 0 -0.003 0.998 0.004
N = > 0 1 0.000 -0.003 1.000
NE > < 1 -1 0.902 0.996 0.884
E > = 1 0 0.000 0.000 1.000
SE > > 1 1 0.000 0.000 1.000
formance is known to be error prone and in cases of
various solutions preferences for particular relations
can be found (Rauh et al., 2005). In the previous sub-
section it was shown that an ANN is basically able to
learn PA . But what happens if the level of believe,
i.e., the target values in the patterns, change? For the
perfect fitting of the previous described ANN the fol-
lowing patterns were used:
[[-1,-1],[1,0,0]],
[[-1, 0],[1,0,0]],
[[-1, 1],[1,1,1]], (1)
[[ 0,-1],[1,0,0]],
[[ 0, 0],[0,1,0]],
[[ 0, 1],[0,0,1]],
[[ 1,-1],[1,1,1]], (2)
[[ 1, 0],[0,0,1]],
and [[ 1, 1],[0,0,1]]. And a variation of some target
values, i.e.
[[-1, 1],[0.9,1,0.9]], (1)
[[ 1,-1],[0.9,1,0.9]], (2)
used in the latter for Cardinal Directions problems,
changes the rounded results shown in Table 3 in the
way the reported preferences of humans suggest. Ta-
ble 4 depicts the outcoming results for both of this
changes by reasoning with PA with the previous de-
scribed ANN with six nodes within the hidden layer.
With a mapping for a two–dimensional C D relation
to two one–dimensional PA relations, the previous re-
sults could be used to pass preferences from one cal-
culus to the other. Considering 3ts-problems in PA
the previously described ANN is used to compute the
possible relation for a given problem. Now, for 3ts-
problems in C D the relations must be split by their x–
and y–dimensions and computed separately. To com-
pute the PA outcome only one ANN is used. Given
z
1
q
1,2
(x, y)z
2
:= z
1
r
1,2
(x)z
2
∧z
1
r
1,2
(y)z
2
, with q
1,2
∈
C D and r
1,2
∈ PA , and z
2
q
2,3
(x, y)z
3
:= z
2
r
2,3
(x)z
3
∧
z
2
r
2,3
(y)z
3
, with q
2,3
∈ C D and r
2,3
∈ PA the in-
puts for the ANN are r
1,2
(x) and r
2,3
(x) for the x–
dimensional information specified in the problem,
and r
1,2
(y) and r
2,3
(y) for the y–dimensional infor-
mation. The mapping of the C D relation is given
in Table 4. The result of the ANN concerning
DeductiveReasoning-UsingArtificialNeuralNetworkstoSimulatePreferentialReasoning
637