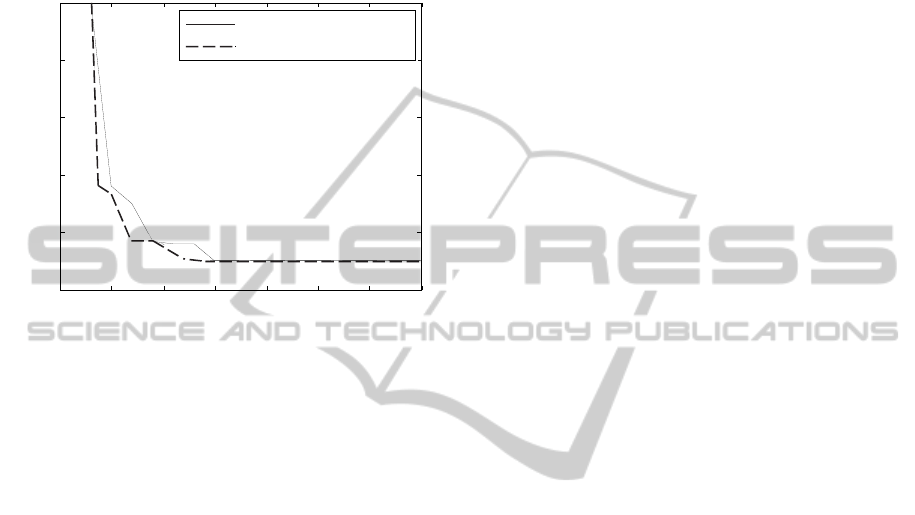
and accuracy at the equal error rate (EER) operating
point are used to evaluate the normalization effect.
Daugman’s normalization method resulted in
accuracy at the EER of 96.31 % while our proposed
normalization method reported a value of 97.24 %.
Figure 2 shows the ROC curves resulting for each of
the normalization methods.
Figure 2: ROC curves resulting from a parabolic
normalization compared to Daugman’s linear
normalization.
The ROC curves give best analyses of accuracy
because they present the achieved accuracy over a
range of operating points. As can be seen in figure 2,
the parabolic normalization improved accuracy at
most operating points, especially at low operating
points where significant accuracy improvements are
shown.
4 CONCLUSIONS
In this paper, we propose a novel iris normalization
method that normalizes the iris following a parabolic
function. Evaluation of the method is performed at
the matching stage using an optimized multilayer
perceptron neural network. Results compared to
Daugman’s normalization show better network
performance, more specifically, 62.5%, 20% and
30.62% lower train, validation and test error
respectively. In addition better accuracy at the EER
operating point and better ROC curves are reported
using parabolic normalization. These results show
that parabolic normalization is convenient to
represent the iris information and contribute in better
iris recognition performance.
REFERENCES
Boles, W., Boashash, B., 1998. A human identification
technique using images of the iris and wavelet
transform. IEEE Transactions on Signal Processing,
46(4).
Broussard, R., Kennell, L., Ives, R. and Rakvic, R., 2008.
An artificial neural network based matching metric for
iris identification. Proceedings of SPIE, 6812, 0-11.
CASIA-IrisV3, http://www.cbsr.ia.ac.cn/IrisDatabase.html
Chen, C., and Chu, C., 2009. High performance iris
recognition based on 1-D circular feature extraction
and PSO–PNN classifier. Expert Systems with
Applications, 36(7), 10351-10356.
Cho, S., and Kim, J., 2006. Iris recognition using LVQ
neural network. Third international conference on
Advances in Neural Networks, 3(2), 26-33.
Daugman, J., 1993. High Confidence Visual Recognition
of Persons by a Test of Statistical Independence. IEEE
Trans. in Pattern Anal. Mach. Intell, 15(11), 1148-
1161.
Daugman, J., 2007. New methods in iris recognition. IEEE
Trans. Syst., Man, Cybern. B, Cybern., 37(5), 1167-
1175.
Krichen, E., 2007. Reconnaissance des personnes par
l’iris en mode dégradé, Evry-Val Essonne University,
thesis.
Lim, S., Lee, K., Byeon, O., and Kim, T., 2001. Efficient
Iris recognition through improvement of feature vector
and classifier. ETRI Journal, 23(2), 1-2.
Ma, L., Wang, Y. and Tan, T., 2002. Iris Recognition
Using Circular Symetric Filters, 16th International
Conference on Pattern Recognition Proceedings, 2,
414- 417.
Murakami, M., Takano, H., Nakamura, K.. 2003. Real-
time Iris recognition by a rotation spreading neural
network. Annual SICE conference, 1, 283-289.
Park, C. H., Lee, J. J., Smith, M. J. T. and Park, K. H.,
2003. Iris-Based Personal Authentication Using a
Normalized Directional Energy Feature. Audio and
video based biometric person authentication
conference, 224-232.
Reaz, M. B. I., Sulaiman, M. S., Yasin, F. M., Leng, T.A.,
2004. Iris recognition using neural network based
onVHDL prototyping. International Conference on
Information and Communication Technologies: From
Theory to Applications, 463-464.
Wildes, R., 1997. Iris recognition: an emerging biometric
technology. Proceedings of the IEEE, 85(9).
Yuan, X. and Shi P., 2005. Advances in Biometric Person
Authentication. Lecture Notes in Computer Science,
3781, 135-141.
Zhu, Y., Tan, T. and Wang, Y., 2000. Biometric personal
identification based on iris patterns. 15th International
Conference on Pattern Recognition, Barcelona , Spain,
2, 801-804.
4.5 5 5.5 6 6.5 7 7.5
x 10
-3
0
0.005
0.01
0.015
0.02
.
False Match Rate
False Non-Match Rate
Daugman's normalization
Parabolic normalization
Daugman's normalization
Parabolic normalization
False Match Rate
-
False Non-Match Rate
4.5
5
5.5
6
6.5
7
7.
8
0.005
0.01
0.015
0.02
0.025
IJCCI2012-InternationalJointConferenceonComputationalIntelligence
646