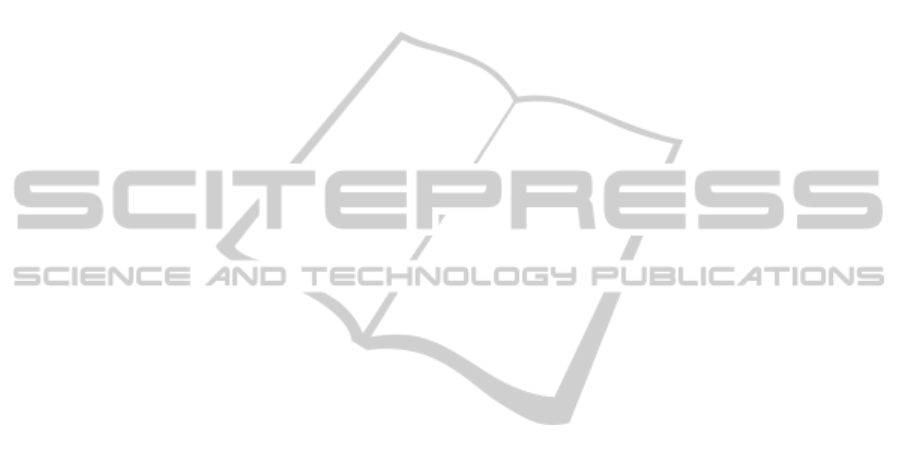
point temperature, short-wave solar radiation, air
pressure, and precipitation), riparian habitat
characteristics (stream bearing, gradients, depth,
substrate, wetted widths, and canopy cover), and
basins landscape characteristics (topographic and
vegetative), acquired by using a geographic
information system (GIS). Their results showed
RMSEs ranging between 0.05°C and 0.59°C and
with R
2
ranging from 0.88 to 0.99.
Comparison of seasonal performance showed
that the ANN model performed best in summer or
autumn, which is consistent with other temperature
models (Caissie et al., 1998); (Caissie et al., 2005);
(Chenard and Caissie, 2008). It could suggest the
potential role of discharge in the modeling
performance, as low water levels are usually
observed in autumn and mid-summer, resulting in
more effective thermal exchange and giving better
performances. The poorer but still good performance
in spring could be explained by the higher discharge
caused by snowmelt, resulting in a poorer air to
water temperature relationship (Caissie et al., 1998).
The performance of the model was closely linked to
water levels, meaning that the performance was
better when water levels were low. At LSWM, the
ANN model performed best in autumn for all the
years, whereas at CatBk, some years had their best
performance during summer. These results suggest
that the thermal exchange is more efficient for less
sheltered river under low flow (autumn at LSWM).
CatBk is more sheltered and could potentially be
influenced by other factors (ex., groundwater)
reducing the efficiency of the thermal exchange. For
example, Hébert, Caissie, Satish, and El-Jabi (2011)
showed that the impact of groundwater on hourly
water temperatures was more significant on smaller
streams, like CatBk.
The training period showed better results than
the validation period, which is consistent in
modeling. Daily water levels used in the modeling
were estimated using power functions (Caissie,
2004). Using hourly water levels instead of daily
water levels could potentially improve the modeling,
especially during days that discharge varied
significantly. However, hourly water levels were not
available for the present study.
ANN models have major advantages over more
commonly used water temperature models, as they
do not need many input data. In this case, only air
temperature and water level time series were used to
achieve good predictions. For instance, deterministic
model needs many hydrological and meteorological
parameters that are not always readily available
(e.g., solar radiation). Another major advantage of
ANN is that they are easy to use and very simple in
their application. However, ANN models cannot
give any physical explanation of the relationship
between the input and output data. These models
should therefore be used with caution, especially
when using input data that are outside the range of
the calibration period (Risley et al., 2003).
5 CONCLUSIONS
This study showed that artificial neural network
(ANN) could be an effective tool for the prediction
of hourly stream temperatures. ANN models
achieved comparable or better performances to other
water temperature models reported in the literature,
with RMSE of 0.94°C at Cat Bk and 1.23°C at
LSWM. ANN models showed a good generalization
capability by modeling well water temperature time-
series. ANN was effectively applied on two
thermally different streams and provides similar
results and performances. As such, ANN models can
be considered as effective modeling tool in water
resources and fisheries management.
REFERENCES
Bélanger, M., El-Jabi, N., Caissie, D., Ashkar, F., Ribi,
J.M. (2005). Estimation de la température de l’eau en
rivière en utilisant les réseaux de neurones et la
régression multiple. Revue des Sciences de l’Eau,
18(3), 403-421.
Caissie, D. (2004). Stream temperature modeling in forest
catchments. PhD Thesis, Dalhousie University,
Halifax, NS, 207p.
Caissie, D. (2006). The thermal regime of rivers: a review.
Freshwater Biology, 51, 1389-1406.
Caissie, D., El-Jabi, N., St-Hilaire, A. (1998). Stochastic
modelling of water temperatures in a small stream
using air to water relations. Canadian Journal of Civil
Engineering, 25, 250-260.
Caissie, D., Satish, M. G., El-Jabi, N. (2005). Predicting
river water temperatures using the equilibrium
temperature concept method with applications on
Miramichi River Catchments (New Brunswick,
Canada). Hydrological Processes, 19, 2137-2159.
Caissie, D., Satish, M. G., El-Jabi, N. (2007). Predicting
water temperatures using a deterministic model:
Application on Miramichi River catchments (New
Brunswick, Canada). Journal of Hydrology, 336(3-4),
303-315.
Chenard, J., Caissie, D. (2008). Stream temperature
modelling using artificial neural networks: application
on Catamaran Brook, New Brunswick, Canada.
Hydrological Processes, 22(17), 3361-3372.
ModelingofRiverWaterTemperaturesusingFeed-forwardArtificialNeuralNetworks
561