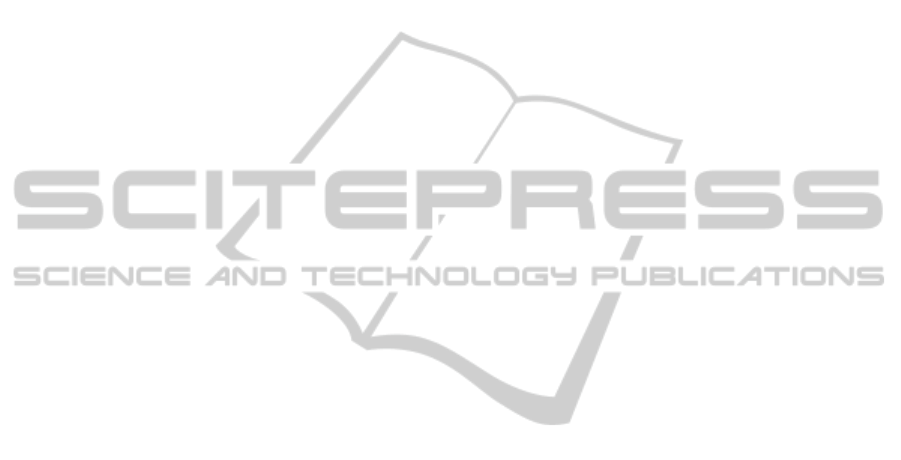
an experimental setup, in order to increase our
understanding on automatically attaining cognitive
styles based on specific Web interaction data of
users. Specific data metrics of CAPTCHA
mechanisms have been proposed and utilised by an
Artificial Neural Network (ANN), with the aim to
predict the users’ cognitive style.
The experimental process of the ANN yielded
very promising results for the sample examined. In
particular, the results obtained with ANNs for
predicting the cognitive styles ratio of individuals
were particularly successful in respect to their real
cognitive style ratio value. This indicates that
techniques such as ANNs are suitable for predicting
users’ cognitive typology using their interaction data
with CAPTCHA-related challenges.
The practicality and significance of this work is
that the suggested ways of capturing intrinsic
characteristics of users, like cognitive styles, and
their analysis through intelligent techniques may be
more effective and less time and effort consuming
than traditional instruments since they might
optimise the user modelling process. However, in
order to build a cohesive user model psychometric
tests are not yet to be replaced since this study is still
in its very early stages.
The meaning of the relation between cognitive
styles and interaction data needs to be further
examined to reach to a more cohesive user model
and effectively guide adaptation in interactive
systems. Future work includes further
experimentation for investigating these relations and
further employing fuzzy algorithms or other
Artificial Intelligence (AI) methods to determine the
degree of adaptation based on user profiles and the
correlations obtained by ANN models such as the
ones used in this work.
ACKNOWLEDGEMENTS
The work is co-funded by the EU project Co-
LIVING (60-61700-98-009), smarTag (University of
Cyprus) and PersonaWeb (Cyprus Research
Promotion Foundation).
REFERENCES
Antoniou, A., Lepouras, G., 2010. Modelling Visitors'
Profiles: A Study to Investigate Adaptation Aspects
for Museum Learning Technologies. J. Computing
Cultural Heritage. 3(2), 1-19.
Belk, M., Papatheocharous, E., Germanakos, P., Samaras,
G., 2012. Investigating User Models through
Cognitive Styles and Navigation Behavior in Web 2.0
Environments using Clustering Techniques. J. Web 2.0
engineering: new practices and emerging challenges,
Elsevier (submitted).
Brusilovsky, P., Kobsa, A., Nejdl, W., 2007. The Adaptive
Web: Methods and Strategies of Web Personalization.
Springer, Heidelberg, Berlin.
Chou, P., Li, P., Chen, K., Wu, M., 2010. Integrating web
mining and neural network for personalized e-
commerce automatic service. Expert Systems with
Applications, Elsevier. 37(4), 2898-2910.
Elson, J., Douceur, J., Howell, J., Saul, J., 2007. Asirra: A
CAPTCHA that Exploits Interest-Aligned Manual
Image Categorization. In CCS’07, International
Conference on Computer and Communications
Security, ACM Press. 366-374.
Felder, R., Silverman, L., 1988. Learning and Teaching
Styles in Engineering Education. Engineering
Education 78(7), 674-681.
Frias-Martinez, E., Chen, S. Y., Macredie R. D., Liu, X.,
2007. The Role of Human Factors in Stereotyping
Behavior and Perception of Digital Library Users: A
Robust Clustering Approach. J. User Modelling and
User-Adapted Interaction, Springer, 17(3), 305-337.
Frias-Martinez, E., Magoulas, G., Chen, S., Macredie, R.,
2005. Modelling Human Behavior in User-Adaptive
Systems: Recent Advances Using Soft Computing
Technique. Expert Systems with Applications, 29(2),
320-329.
Golle, P., 2008. Machine Learning Attacks Against the
Asirra CAPTCHA. In CCS’08, International
Conference on Computer and Communications
Security, ACM Press. 535-542.
Haykin, S., 1999. Neural Networks: A Comprehensive
Foundation, Prentice Hall. Upper Saddle River, NJ.
2
nd
edition.
Kim, M., Kim, E., Ryu J., 2004. A Collaborative
Recommendation Based on Neural Networks. In Lee,
Y., Li, J., Whang, K., Lee, D. (Eds.), Database
Systems for Advanced Applications. LNCS, Springer,
2973, 425-430.
Magoulas, G. D., Papanikolaou, K. A., Grigoriadou, M.,
2001. Neuro-fuzzy synergism for planning the content
in a web-based course. Informatica, 25(1), 39-48.
Perkowitz, M., Etzioni, O., 2000. Adaptive Web Sites.
Communications of the ACM, ACM Press. 43(8), 152-
158.
Riding, R., 2001. Cognitive Style Analysis - Research
Administration. Learning and Training Technology.
Riding, R., Cheema, I., 1991. Cognitive styles - An
Overview and Integration. Educational Psychology.
11, 3/4, pp. 193-215.
Rumelhart, D. E., Hinton, G. E., Williams, R. J., 1986.
Learning Internal Representations by Error
Propagation. In Parallel Distributed Processing:
Explorations in the Microstructure of Cognition, MIT
Press. 1, 318-362.
Securimage v.3.0., 2012. http://www.phpcaptcha.org.
Von Ahn, L., Blum, M., Langford, J., 2004. Telling
IJCCI2012-InternationalJointConferenceonComputationalIntelligence
568