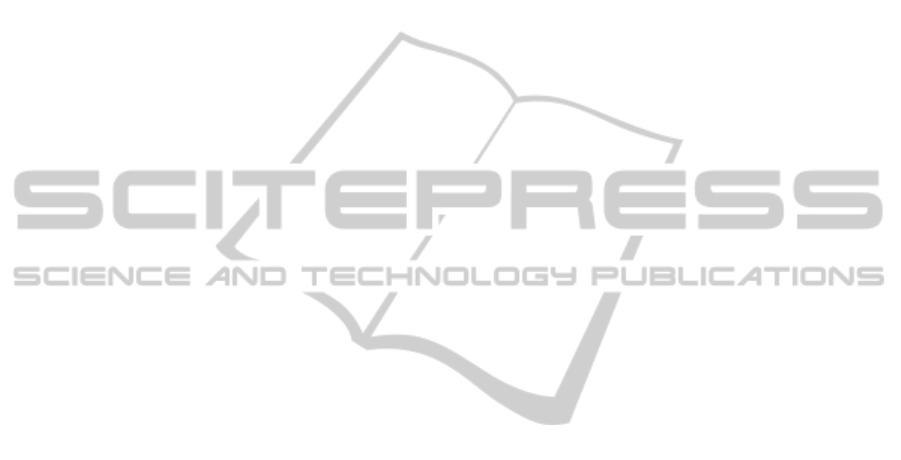
sidering 5 different experiments. The force-velocity
diagram shows that the MR
2
damper can be mod-
eled with high accuracy by the proposed ANN struc-
ture since this shock absorber has an on/off actuation
and does not have hysteresis; while the MR
1
damper
presents a more complex dynamics at high frequen-
cies with high displacements and the MR damper
model based on the proposed 1-hidden layer structure
can not represent this hysteretic behavior with only
one sensor. However, this displacement pattern is out
of the automotive operational zone of the damper, i.e.
it does not occur at normal driving conditions.
By comparing the modeling performance of the
proposed MR damper model based on ANN with an-
other MR damper models presented in the literature,
it is considerable to assume an optimal modeling per-
formance. In the proposed ANN model, the obtained
modeling error of 7.25% based on the RMS is equiv-
alent to 4.7% of Error to Signal-Ratio (ESR), this
means that the error in the proposed ANN model
is: 1) lower than the ESR average (14.5%) obtained
by the Bingham model and reported in (Savaresi
et al., 2005); 2) lower than the ESR average (8.7%)
obtained by a phenomenological model reported in
(Ruiz-Cabrera et al., 2010); lower than the ESR av-
erage (38.7%) obtained by a semi-phenomenological
model reported in (Ruiz-Cabrera et al., 2010); but
greater than the ESR average of (0.9%) and (2%) ob-
tained by an ANN model reported in (Savaresi et al.,
2005) and (Ruiz-Cabrera et al., 2010) respectively.
Although these latter ANN structures have regressors,
use the output feedback and demand the displacement
and velocity sensor.
Due to reliability of the proposed MR damper
model and simplicity on the ANN structure, the model
can be used to test semiactive suspension control sys-
tems. A control technique free of model has been
used to control the semiactive suspension of a quar-
ter of vehicle system; the performance of the passive
suspension was used as benchmark. Simulation re-
sults show that passengers comfort and road holding
can be increased at least 7.4% and 40.4% respectively,
when an MR semiactive suspension is used.
ACKNOWLEDGEMENTS
Authors thank to CONACYT (Program of Postgradu-
ate Cooperation - 2010) for the financial supports of
this research.
REFERENCES
Ahn, K., Islam, M., and Truong, D. (2008). Hysteresis
modeling of magneto-rheological (mr) fluid damper
by self tuning fuzzy control. In ICCAS 2008, Seoul
Korea, pages 2628–2633.
Atray, V. and Roschke, P. (2003). Design, fabrica-
tion, testing and fuzzy modeling of a large magneto-
rheological damper for vibration control in a railcar.
In IEEE/ASME Joint Rail Conf., pages 223–229.
Boada, M., Calvo, J., Boada, B., and D´ıaz, V. (2011). Mod-
eling of a magnetorheological damper by recursive
lazy learning. International Journal of Non-Linear
Mechanics, 46:479–485.
C¸ esmeci, S. and Engin, T. (2010). Modeling and test-
ing of a field-controllable magnetorheological fluid
damper. International Journal of Mechanical Sci-
ences, 52(8):1036–1046.
Chang, C. and Zhou, L. (2002). Neural network emulation
of inverse dynamics for a magnetorheological damper.
Journal of Structural Engineering, 128(2):231–239.
Chen, E., Si, C., Yan, M., and Ma, B. (2009). Dy-
namic characteristics identification of magnetic rhe-
ological damper based on neural network. In Ar-
tificial Intelligence and Computational Intelligence,
2009, Shangai, China, pages 525–529.
Choi, S., Lee, S., and Park, Y. (2001). A hysteresis
model for field-dependent damping force of a mag-
netorheological damper. J. of Sound and Vibration,
245(2):375–383.
Dong, X., Yu, M., Liao, C., and Chen, W. (2010). Com-
parative research on semi-active control strategies for
magneto-rheological suspension. Nonlinear Dynam-
ics, 59:433–453.
Du, H., Szeb, K., and Lam, J. (2005). Semiactive h
1
con-
trol of vehicle suspension with magneto-rheological
dampers. J. of Sound and Vibration, 283:981–996.
Freeman, J. and Skapura, D. (1991). Neural Networks: Al-
gorithms, Applications and Programming Techniques.
Adisson-Wesley.
Gamota, D. and Filisko, F. (1991). Dynamic mechanical
studies of electrorheological materials: Moderate fre-
quencies. Journal of Rheology, 35:399–425.
Guo, S., Yang, S., and Pan, C. (2006). Dynamical modeling
of magneto-rheological damper behaviors. Int. Mater.,
Sys. and Struct., 16:3–14.
Hagan, M., Demuth, H., and Beale, M. (1996). Neural Net-
work Design. PWS Publishing.
Hong, K., Sohn, H., and Hedrick, J. (2002). Modi-
fied skyhook control of semi-active suspensions: A
new model, gain scheduling, and hardware-in-the-
loop tuning. J. Dyn. Sys., Meas., Control, 124(1):158–
167.
Korbicz, J., Koscielny, J., Kowalczuk, Z., and Cholewa,
W. (2004). Fault Diagnosis Models, Artificial Intel-
ligence, Applications. Springer.
Kwok, N., Ha, Q., Nguyen, T., Li, J., and Samali, B. (2006).
A novel hysteretic model for magnetorheological fluid
dampers and parameter identification using particle
MRDamperIdentificationusingANNbasedon1-Sensor-AToolforSemiactiveSuspensionControlCompliance
501