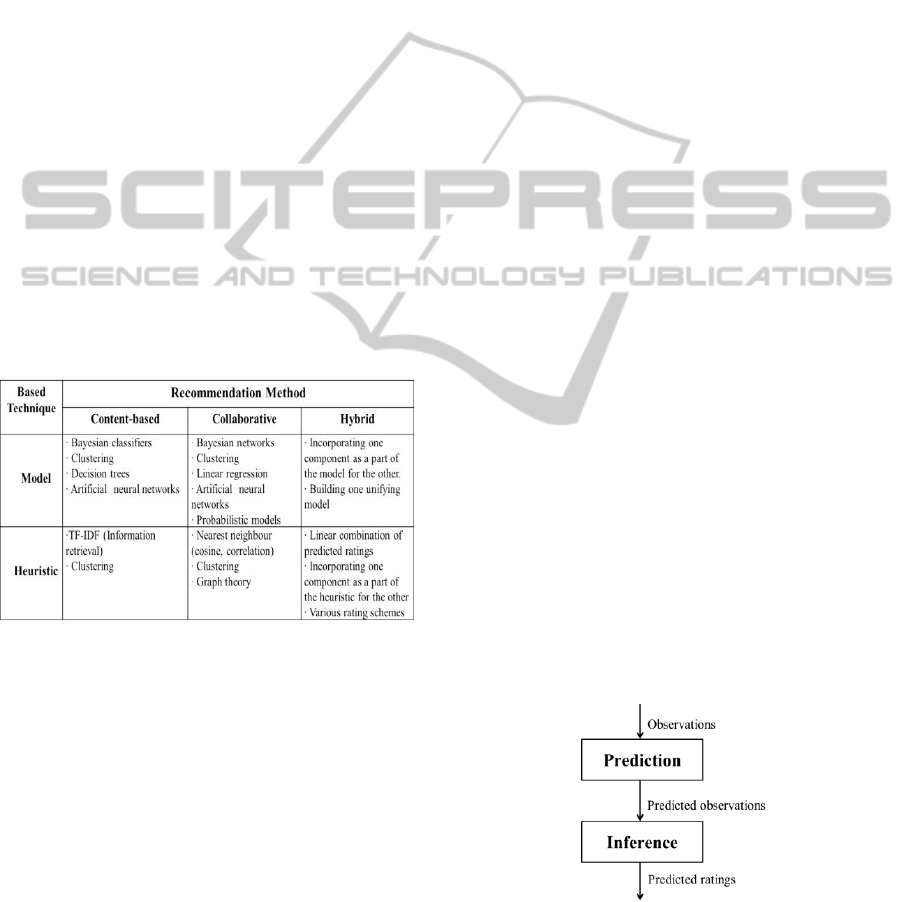
and pure user-based methods of recommendation,
hybrid methods have been created based upon
collaborative filtering and content-based, which
maintains user profiles based on content analysis,
and directly compares these profiles to determine
similar users for collaborative recommendation
(Balabanovic and Shoham, Y., 1997).
To capture the context in which
recommendations are made the multidimensional
approach to recommendations extended to support
additional dimensions capturing the context in which
recommendations are made (Adomavicius and
Tuzhilin, 2001).
All of the work is not only focused on
recommending items to users and users to items, but
takes into consideration additional contextual
information such as time, place, the company of
other people, and other factors affecting
recommendation experiences (Adomavicius et al.,
2005).
Although these different approaches there are
three main recommendation methods, as can be seen
in Figure 1, for finding similar items and similar
users, and can be applied to each of them, techniques
based on heuristics or on models for the rating
estimation (Adomavicius and Tuzhilin, 2005).
Figure 1: Methods used in recommendation systems -
Adapted from: (Adomavicius and Tuzhilin, 2005).
The RS now have the capability to capture
context, through several methods of
recommendation and use of techniques based on
models or heuristics.
To unify user-based and item-based collaborative
filtering approaches (Wang et al., 2006) uses the
similarity fusion. This unification allows the
estimation of final rating by fusing predictions from
three sources: i) predictions based on ratings of the
same item by other users; ii) predictions based on
different item ratings made by the same user; iii)
ratings predicted based on data from other but
similar users rating other but similar items.
The user-based collaborative filtering has been
proven to be the most successful technology for
building recommender systems so far, and is
extensively used in many commercial recommender
systems, although content-based collaborative
recommendation system solves many of the
problems (Patel and Balakrishnan, 2009).
3 OBJECTIVES
The main objective is to develop a website for
electronic commerce oriented to the publication of:
(Scientific Magazines, Books, E-books and
Articles).
Although the existence of several online e-
commerce systems, the purpose is to develop a
flexible website, better than most of the already
existing systems giving each user a different
experience with the online store based on his profile.
The focus is on RS, and should be considered the
several approaches previously mentioned by
focusing on user-based collaborative approach by
applying filtering techniques based on models and
heuristics, putting an emphasis on the user profile.
The differentiating aspect of this system is that it
must be sufficiently intelligent to take the initiative
and proactively recommending the user, content that
we believe is of interest to him.
The user profile must contain essential
information about an individual user and the
motivation of building user profiles is that users
differ in their interest, preferences, backgrounds and
goals when using software applications, discovering
these differences is essential to provide customized
services (Schiaffino and Amandi, 2009).
For the content of the user profile this project
will not only consider the content provided by the
user explicitly, but must infer unobservable
information about users from observable information
about them (Zukerman and Albrecht, 2001).
Figure 2: Predicted observations strategy - Source: (Oard
and Kim, 1998).
It should be combined inference and prediction
to assist the user finding content that is of personal
ICE-B 2012 - International Conference on e-Business
290