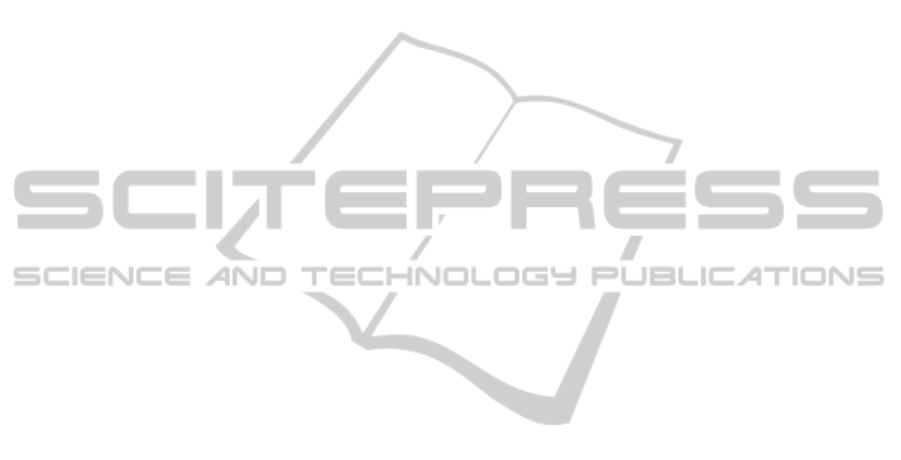
however, the different potential pathways for
anthropogenic GHG emissions, which are used to
drive the climate models. Future emissions depend
on numerous driving forces, including population
growth, economic development, energy supply and
use, land-use patterns, and a variety of other human
activities (Special Report on Emissions Scenarios,
SRES). Future temperature scenarios have been
obtained with the emission profiles corresponding to
the four principal SRES families (A1, A2, B1, and
B2) (Nakicenovic et al., 2000). From the point of
view of a policy-maker, the results of the 3rd and 4th
IPCC’s assessments regarding the projection of
global or regional temperature increases are difficult
to interpret due to the wide range of the estimated
warming. Nevertheless this is an aspect of
uncertainty that scientists and ultimately policy-
makers have to deal with. Furthermore, most of the
available methodologies that have been proposed for
supporting decision-making under uncertainty do not
take into account the nature of climate change’s
uncertainty and are based on classic statistical theory
that might not be adequate. Climate change’s
uncertainty is predominately epistemic and,
therefore, it is critical to produce or adapt
methodologies that are suitable to deal with it and
that can produce policy-useful information. The lack
of such methodologies is noticeable in the IPCC’s
AR4 Contribution of the Working Group I, where
the proposed best estimates, likely ranges and
probabilistic scenarios are produced using
statistically questionable devices (Gay and Estrada,
2009).
Two main strategies have been proposed for
dealing with uncertainty: trying to reduce it by
improving the science of climate change a feat tried
in the AR4 of the IPCC, and integrating it into the
decision-making processes (Schneider, 2003). There
are clear limitations regarding how much of the
uncertainty can be reduced by improving the state of
knowledge of the climate system, since there
remains the uncertainty about the emissions which is
more a result of political and economic decisions
that do not necessarily obey natural laws.
Therefore, we propose that the modern view of
climate modelling and decision-making should
become more tolerant to uncertainty because it is a
feature of the real world (Klir and Elias, 2002).
Choosing a modelling approach that includes
uncertainty from the start tends to reduce its
complexity and promotes a better understanding of
the model itself and of its results. Science and
decision-making have always had to deal with
uncertainty and various methods and even branches
of science, such as Probability, have been developed
for this matter (Jaynes, 1957). Important efforts have
been made for developing approaches that can
integrate subjective and partial information, being
the most successful ones Bayesian and maximum
entropy methods and more recently, fuzzy set theory
where the concept of objects that have not precise
boundaries was introduced (Zadeh, 1965). Fuzzy
logic provides a meaningful and powerful
representation of uncertainties and is able to express
efficiently the vague concepts of natural language
(Zadeh, 1965). These characteristics could make it a
very powerful and efficient tool for policy makers
due to the fact that the models are based on
linguistic rules that could be easily understood.
In this paper two fuzzy logic models are
proposed for the global temperature changes (in the
year 2100) that are expected to occur in this century.
The first model incorporates the uncertainties related
to the wide range of emission scenarios and
illustrates in a simple manner the importance of the
emissions in determining future temperatures. The
second incorporates the uncertainty due to climate
sensitivity that pretends to emulate the diversity in
modelling approaches. Both models are built using
the Magicc (Wigley, 2008) model and Zadeh´s
extension principle for functions where the
independent variable belongs to a fuzzy set. Magicc
is capable of emulating the behaviour of complex
GCMs using a relative simple one dimensional
model that incorporates different processes e.g.
carbon cycle, earth-ocean diffusivity, multiple gases
and climate sensitivity. In our second case we intend
to illustrate the combined effects of two sources of
uncertainty: emissions and model sensitivity. It is
clear that we are leaving out of this paper other
important sources of uncertainty whose contribution
would be interesting to explore. The GCMs are,
from our point of view, useful and very valuable
tools when it is intended to study specific aspects or
details of the global temperature change.
Nevertheless, when the goal is to study and to test
global warming policies, simpler models much
easier to understand become very attractive. Fuzzy
models can perform this task very efficiently.
2 FUZZY LOGIC MODEL
OBTAINED FROM IPCC DATA
The Fourth Assessment Report of the IPCC shows
estimates of emissions, concentrations, forcing and
temperatures through 2100 (IPCC, 2007). Although
there are relationships among these variables, as
SimpleFuzzyLogicModelstoEstimatetheGlobalTemperatureChangeDuetoGHGEmissions
519