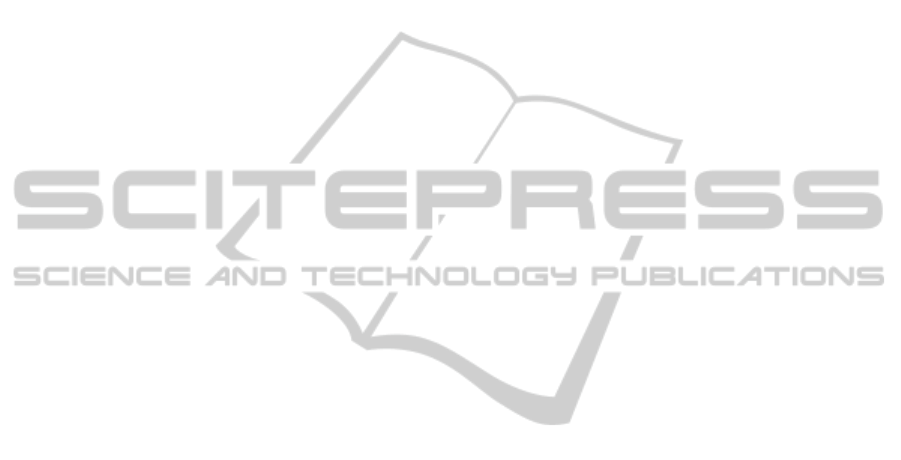
if bed occupancy level i is low then the LoS of
a patient is increased in one day with certain
probability, PIi. There is a maximum of days
DEi that the LoS of a patient can be extended.
These rules can be identically defined for all type
of patients or they can be different for different
groups of patients. For example, we could
distinguish the group of programmed-surgery
patients -usually whit a short LoS- and consider that
it can only be shortened in one day with certain
probability PC
i
when bed occupancy level is i.
These set of rules defines infinite management
policies for the ICU: one for each set of parameter
values. To choose the rule that better fit the decision
making process in the ICU, in (Mallor and Azcárate
2012), we formulated an optimization problem with
the aim of matching as much as possible the output
of the simulation with the ICU historical data (see
Figure 2).
The decision variables are the parameters PR,
DR, PI, DE and PC above defined. Constraints
represent realistic monotonous relationships into
each set of parameters and upper bounds for their
values (uPR, uDR, uPI, uDE and uPC). The number
of ICU beds is denoted by n and by k1 and k2 the
boundaries for low and high occupancy levels,
respectively. We set as objective function to
minimize the squared differences of both occupancy
bed frequency distributions: the one observed in the
real ICU, real_freq(i), and the one obtained from the
simulation output, simul_freq(i). The proposed
optimization problem can be solved by combining
simulation and optimization techniques. The
optimizer produces a sequence of solutions whose
performance is tested in the simulator.
Figure 2: Optimization problem to determine the
parameter values of the medical management rules.
3 SENSITIVITY ANALYSIS
The discharge policy is influenced by the occupancy
level which also depends on the bed availability and
the input rate of patients. Then a bed capacity study
should take into account variability in management
policies. We show in this section that main
performance measures greatly depend on the
adopted policy.
3.1 Case Study. Parameterization of
The Rules
In a first step of our research we developed a
simulation model that included the representation of
the medical decisions made at the ICU of the
Hospital of Navarre, in Spain, following the
methodology presented in Section 2.
The Hospital of Navarra is a general public
hospital with reference specialties in the Community
of Navarra (Neurosurgery, cardiac surgery, vascular
surgery, oncology, infectious diseases, etc.). It has
483 beds, 2015 members of staff and 10 surgery
rooms. The ICU of this hospital has 20 beds and 86
physicians and nurses. It receives patients from 3
sources (emergency, operating theatre and ward).
A thorough data analysis was conducted to
obtain good statistical models for the arrival pattern
and LoS of each of the 8 groups of patients
considered. The necessary data for the statistical
estimation were recorded and provided by the
Hospital administration. We used two files: a patient
file and a bed occupancy file, containing 9 years of
data. The patient file includes all records of patients
attended in the ICU. For each patient the following
variables are known: age, arrival date, illness group
(8 groups were considered), output date, APACHE
(illness severity), infections in the ICU, and exitus
(recovered or died). The bed occupancy file records
the number of occupied beds at 4 p.m., each day. It
was used to validate the simulation model.
Cardiac surgery patients are special patients in
the performance of this ICU and represent 1/3 of the
total amount of patient arrivals.
We simulate the ICU model under different rules
to analyse their influence in a set of performance
measures. In order to make an easier comparison of
the results we simplify the structure of values that
can take the decision rule’s parameters. We
distinguish two levels for the state of high bed
occupancy:
moderate high occupancy (75%-85%): when
there are a number of occupied beds from 15
to 17. It is denoted by level h1, and
very high occupancy (+85%): when there are a
number of occupied beds from 18 to 20. It is
denoted by level h2.
Low occupation levels are also reduced: level l1
from 1 to 8 beds, and level l2, from 9 to 13 beds.
21 ,11 2integer
0...
0.....
0.....
0...
0...
)(_)(_
21
11
11
21
21
0
2
kk,...,kj,...,nkni ,DIDR
PCPCPCuPC
PIPI uPI
DEDEuDE
PRPRPR uPR
DRDRDR uDR
tosubject
ifreqsimulifreqrealMin
ji
knnn
k
k
knnn
knnn
n
i
SIMULTECH 2012 - 2nd International Conference on Simulation and Modeling Methodologies, Technologies and
Applications
550