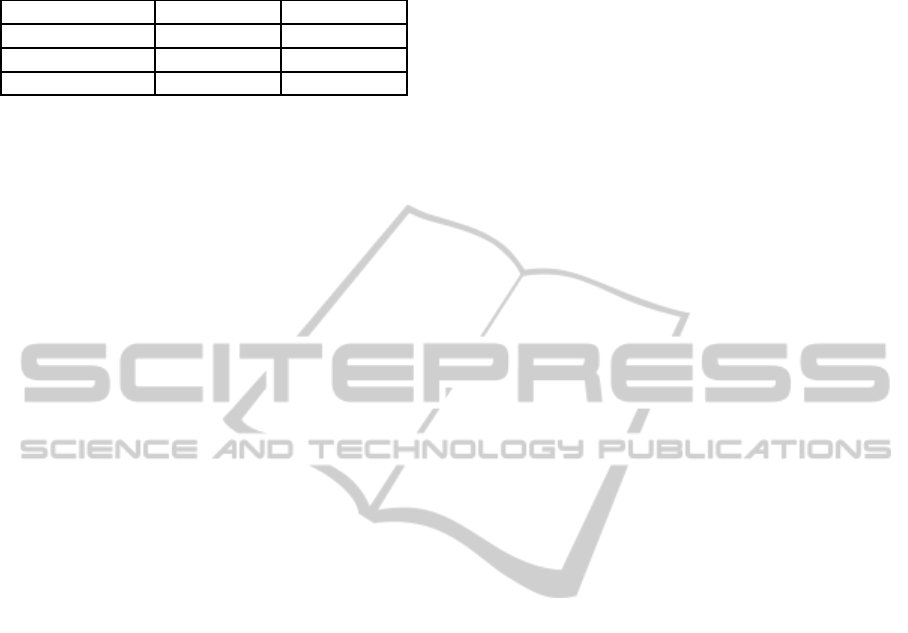
Table 3: Comparison results in the case of the first floor of
Charles Nicole hospital.
Hopfield Som
Patterns number 18 18
Error rate 23 % 36%
Time steps 50 350
The results of the tests were in the favor of Hop-
field as they showed respectable error rates and ra-
pidity in convergence time of Hopfield comparing to
SOM. Hopfield also provided more logical and intel-
ligent results.
In fact, we noticed that SOM tends to an illogical
generation of the shortest path even when it hasn’t to
do it.
7 CONCLUSIONS
The problem of evacuation is an important subject of
actuality which was the object of a lot of interest in the
research’s field. This paper dealt with the microscopic
type of evacuation which focuses on the behavior of
each evacuee during the egress process.
We carried our interest on Hopfield neural net-
works known by their simple architecture and their
effectiveness in different domains especially the do-
main of pattern recognition and combinatory opti-
mization. The model is based on an auto-associative
memory concept which permits to recoverany learned
information providing the network with a respectable
degree of intelligence.
We proposed a model able to intelligently draw
evacuee’s itineraries in a building, where there is
a panic situation, according to some characteristics.
The parameters we thought effective in getting the
evacuee behavior are his age category, his gender, his
familiarity with the building, his fire experience and
his starting position.
To lighten our model’s contribution in artificial
neural network approaches for evacuation, we sup-
plied a comparative study between our model and
SOM model proposed in (Ben Salem et al., 2011).
Hopfield gave largely better results than SOM. It con-
served its rapidity quality adding to it the accomplish-
ment of a better rate of error than SOM.
Even if Hopfield networks succeeded in simulat-
ing the evacuation process, the model could be im-
proved by including other factors as the time of evac-
uation and the speed of people.
It would be also interesting to improve the quality
of the learning set as we could deepen it by taking
the opinion of sociologists and specialists of human
behavior.
Finally, it would be promising to enlarge Hopfield
capacity of learning.
REFERENCES
Abraham, A. (2005). Artificial Neural Networks. Peter H.
Sydenham and Richard Thorn, John Wiley and Sons
Ltd.
Ben Salem, Z. N., Bouziri, H., and Jelassi, S. (2011). A
som based predictive model for fire building evacu-
ation. In Communications, Computing and Control
Applications (CCCA), 2011 International Conference,
pp.1-5.
Heaton, J. (2005). Introduction to Neural Networks with
Java. The Heaton Research Inc.
Klupfel, H., Konig, T., Wahle, J., and Schreckenberg, M.
(2000). Microscopic simulation of evacuation pro-
cesses on passenger ships. In Proceedings of the
Fourth International Conference on Celular Automata
for Research and Industry, Karlsruhe, Springer-
Verlag, pp. 63-71, London.
Letavec, C. and Ruggiero, J. (2002). The n-Queens Prob-
lem. The INFORMS Transactions on Education 2, pp.
101-103.
Letchford, A., Lodi, A., and Wenger, K. M. (2011). The
Travelling Salesman Problem. Mathematical Pro-
gramming Computation, vol. 3, no. 1, pp. 59-78.
Pandey, B., Ranjan, S., Shukla, A., and R., T. (2010). Sen-
tence recognition using hopield neural network. In
IJCSI International Journal of Computer Science Is-
sues, vol. 7, iss. 4, no. 6.
Yu, J. (2003). Artificial Neural Net-
work: Assignment. Retrieved from
http://users.cecs.anu.edu.au/jinyu/JinYules/preproj/A
NN.pdf.
HopfieldNeuralNetworkforMicroscopicEvacuationofBuildings
581