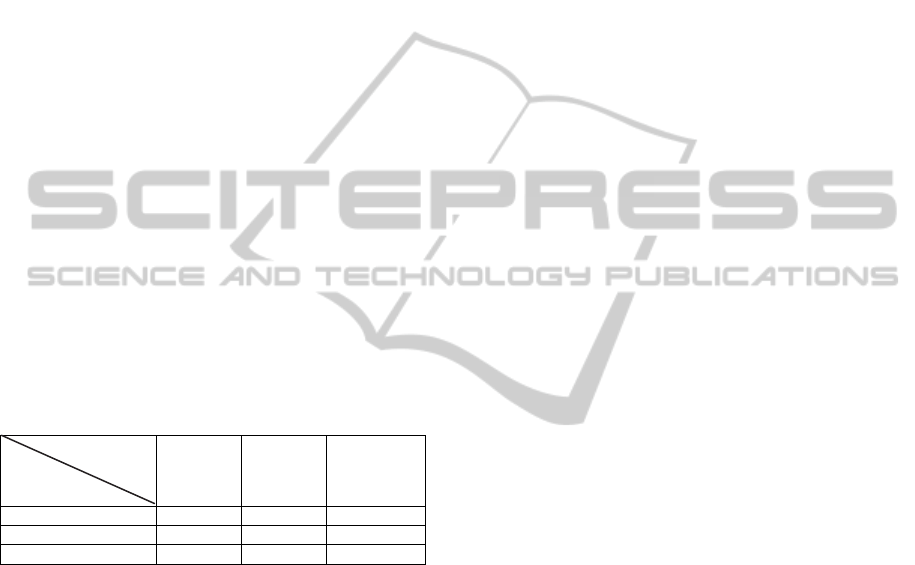
4.4 Discussion
The methods’ accuracies are compared in terms of
RMSE, standard deviation SD, and absolute percent
relative error (APRE). The regression gave for no
correction, average APRE is 111%, RMSE is 210.39
ft/hr and SD is 179.89 ft/hr. For WOB correction,
the average APRE is 85%, RMSE is 133.73ft/hr and
SD is 107.18 ft/hr. For interpolated correction,
average APRE is 30%, RMSE is 57.29 ft/hr and SD
is 57.25 ft/hr. Therefore, the interpolated correction
are compared with the other techniques and the data
plugged in ELM and RBF models are those of the
interpolated corrected.
For ELM, we see each activation function
separately. From Fig. 5, it can be seen that the most
accurate results are at sigmoidal with 5 hidden
neurons. Table 3 shows that the most accurate
method of implementing RBF is with MSE = 6400
ft
2
/hr
2
and spread = 20. Therefore, we take this
combination as the candidate of comparison. Table 4
shows the comparison among the techniques in
terms of testing accuracies.
Comparing the results above, we can see that
RBF is the most accurate technique. However, ELM
is the fastest. Therefore, depending on the objective,
a decision can be made.
Table 4: Comparison of testing accuracies.
technique
ELM RBF Regression
criterion
RMSE(ft/hr) 51.9716 34.996 37.36152
SD(ft/hr) 44.405 34.9756 64.71206
APRE 17.13% 9.6% 33%
5 CONCLUSIONS
This paper has shown a comparison among ELM,
RBF, and a multiple regression model for ROP
Prediction. The professionals and decision makers
are advised, according to the results of this study, to
choose RBF as the ROP prediction technique.
However, if processing speed is more important, the
decision makers might want to use ELM. Additional
ANN techniques can be used in development of this
study. Some of them are being implemented in an
ongoing work.
ACKNOWLEDGEMENTS
The authors would like to thank KACST for
supporting this work under grant: KACST-OIL-AR-
30-258-2012, and Dr. Tuna Eren, for providing real
time data and regression models. Mr. Alarfaj gives
special thanks to KFUPM and Systems Engineering
Department for offering the SE 439 course on
undergraduate research.
REFERENCES
Abtahi A., Butt S., and Molgaard, J., and Arvani F., 2011.
“Bit Wear Analysis and Optimization for Vibration
Assisted Rotary Drilling
) VARD (using Impregnated
Diamond Bits”, Memorial University of
Newfoundland, St. John’s, NL, Canada.
Adrian G. Bors 1996. “Introduction to the Radial Basis
Function (RBF) Networks”, University of York.
Available: www-sers.cs.york.ac.uk/adrian/Papers/
Others/OSEE01.pdf>.
Awasthi, Ankur 2008. “Intelligent oilfield operations with
application to drilling and production of hydrocarbon
wells”, University of Houston, 2008, 370p.
Bataee M., & Mohseni, S., 2011. “Application of artificial
intelligent systems in ROP optimization: A case study
in Shadegan oil field”. SPE Middle East
Unconventional Gas Conference and Exhibition
2011.
Bourgoyne A.T. Jr., Young F.S., 1974 “A Multiple
Regression Approach to Optimal Drilling and
Abnormal Pressure Detection”, SPE 4238, August
1974.Available: ttp://www.onepetro.org/mslib/servlet/
onepetropreview?id=00004238>.
Eren Tuna, 2010. “Real-Time-Optimization Of Drilling
Parameters During Drilling Operations Dissertation”
PhD
Technical University of the Middle East, Turkey.
“Getting Started with Matlab 7” www.mathworks.com
(2007).
Gidh Yashodhan, Ibrahim Hani, Purwanto Arifin, and Bits
smith, 2011. “Real-time drilling parameter
optimization system increases ROP by
predicting/managing bit wear”. SPE Digital Energy
conference and Exhibition, 2011.
Hamrick, Todd Robert, 2011. “Optimization of Operating
Parameters for Minimum Mechanical Specific Energy
in Drilling”, West Virginia University, 148 p.
Huang, Guang-Bin, Wang, Dian Hui, and Lan, Yuan,
2011. “Extreme learning machines: a survey”, Int. J.
Mach. Learn. & Cyber. (2011) 2:107–122.
Huang, Guang-Bin, 2010. “Extreme Learning Machine:
Learning Without Iterative Tuning”, Available:
http://www.extreme-learning-machines.org/.
Huang, Guang-Bin, Zhu, Qin-Yu, Siew, Chee-Kheong,
2006. “Extreme learning machine: Theory and
applications”, SienceDirect.
http://www.mathworks.com/help/toolbox/nnet/ref/newrb.h
tml.
Khoukhi A., 2012. “Hybrid soft computing systems for
reservoir PVT properties prediction”, Computers and
Geosciences, Elsevier, vol. 44, p: 109–119 2012.
RateofPenetrationPredictionandOptimizationusingAdvancesinArtificialNeuralNetworks,aComparativeStudy
651