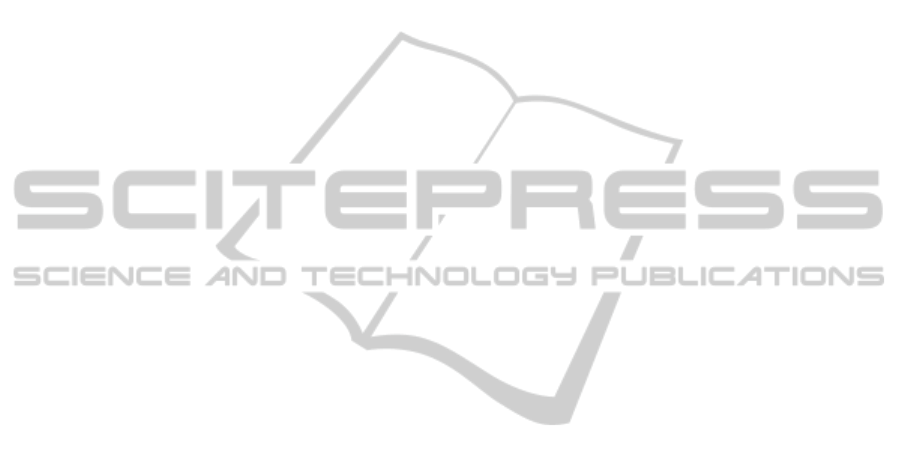
erations that cannot be apprehended by the classical
Turing machine model yet play a crucial role in many
aspects of neural computation
According to (Siegelmann, 2003), such results
“embeds a possible answer to the superiority of bio-
logical intelligence within the framework of classical
computer science”. We prefer to remain cautious on
this issue, and do not intend to argue in favor of an
ontological existence of super-Turing capabilities of
biological neural networks in nature, but rather in fa-
vor of the relevance of considering super-Turing neu-
ral models in order to describe neurobiological fea-
tures that fail be captured via Turing-equivalent clas-
sical models of computation. In this sense, we be-
lieve that the consideration of super-Turing brain-like
computational models present some interest beyond
the philosophical controversial considerations about
hypercomputation (Copeland, 2004).
Finally, we expect that such theoretical stud-
ies about the computational power of neural models
might lead to a better understanding of the basic prin-
ciples that underlie the processing of information in
the brain. In this context, we believe that the compar-
ative approach between the computational powers of
bio-inspired and artificial abstract models of compu-
tation shall ultimately provide a better understanding
of the intrinsic natures of both biological and artificial
intelligences. We further believe that the foundational
approach to alternative models of computation might
in the long term not only lead to relevant theoretical
considerations, but also to important practical impli-
cations. Similarly to the theoretical work from Turing
which played a crucial role in the practical realization
of modern computers, further foundational consider-
ations of alternative models of computation will cer-
tainly contribute to the emergence of novel computa-
tional technologies and computers, and step by step,
open the way to the next computational era.
REFERENCES
Cabessa, J. (2012). Interactive evolving recurrent neural
networks are super-Turing. In Filipe, J. and Fred, A.,
editors, ICAART 2012: Proceedings of the 4th Inter-
national Conference on Agents and Artificial Intelli-
gence 2012, volume 1, pages 328–333. SciTePress.
Cabessa, J. and Siegelmann, H. T. (2011). Evolving re-
current neural networks are super-Turing. In IJCNN
2011: Proceedings of the International Joint Con-
ference on Neural Networks 2011, pages 3200–3206.
IEEE.
Cabessa, J. and Siegelmann, H. T. (2012). The computa-
tional power of interactive recurrent neural networks.
Neural Comput., 24(4):996–1019.
Cabessa, J. and Villa, A. E. (2012). The expressive power
of analog recurrent neural networks on infinite input
streams. Theor. Comput. Sci., 436:23–34.
Copeland, B. J. (2004). Hypercomputation: philosophical
issues. Theor. Comput. Sci., 317(1–3):251–267.
Goldin, D., Smolka, S. A., and Wegner, P. (2006). Inter-
active Computation: The New Paradigm. Springer-
Verlag, Secaucus, NJ, USA.
Kaneko, K. and Tsuda, I. (2003). Chaotic itinerancy. Chaos,
13(3):926–936.
Kilian, J. and Siegelmann, H. T. (1996). The dynamic uni-
versality of sigmoidal neural networks. Inf. Comput.,
128(1):48–56.
Kleene, S. C. (1956). Representation of events in nerve nets
and finite automata. In Automata Studies, volume 34
of Annals of Mathematics Studies, pages 3–42. Prince-
ton University Press, Princeton, N. J.
McCulloch, W. S. and Pitts, W. (1943). A logical calculus
of the ideas immanent in nervous activity. Bulletin of
Mathematical Biophysic, 5:115–133.
Minsky, M. and Papert, S. (1969). Perceptrons: An Intro-
duction to Computational Geometry. MIT Press.
Minsky, M. L. (1967). Computation: finite and infinite ma-
chines. Prentice-Hall, Inc.
Rosenblatt, F. (1957). The perceptron: A perceiving and
recognizing automaton. Technical Report 85-460-1,
Cornell Aeronautical Laboratory, Ithaca, New York.
Siegelmann, H. T. (1995). Computation beyond the Turing
limit. Science, 268(5210):545–548.
Siegelmann, H. T. (2003). Neural and super-Turing com-
puting. Minds Mach., 13(1):103–114.
Siegelmann, H. T. and Sontag, E. D. (1994). Analog com-
putation via neural networks. Theor. Comput. Sci.,
131(2):331–360.
Siegelmann, H. T. and Sontag, E. D. (1995). On the com-
putational power of neural nets. J. Comput. Syst. Sci.,
50(1):132–150.
Tsuda, I. (1991). Chaotic itinerancy as a dynamical basis
of hermeneutics of brain and mind. World Futures,
32:167–185.
Tsuda, I. (2001). Toward an interpretation of dynamic neu-
ral activity in terms of chaotic dynamical systems. Be-
hav. Brain Sci., 24(5):793–847.
Turing, A. M. (1936). On computable numbers, with an ap-
plication to the Entscheidungsproblem. Proc. London
Math. Soc., 2(42):230–265.
Van Leeuwen, J. and Wiedermann, J. (2001). Beyond the
Turing limit: Evolving interactive systems. In Pa-
cholski, L. and Ruˇzicka, P., editors, SOFSEM 2001:
Theory and Practice of Informatics, volume 2234 of
LNCS, pages 90–109. Springer-Verlag.
Van Leeuwen, J. and Wiedermann, J. (2008). How we think
of computing today. In Beckmann, A., Dimitracopou-
los, C., and L¨owe, B., editors, Logic and Theory of
Algorithms, volume 5028 of LNCS, pages 579–593.
Springer-Verlag.
Von Neumann, J. (1958). The computer and the brain. Yale
University Press, New Haven, CT, USA.
Wegner, P. (1998). Interactive foundations of computing.
Theor. Comput. Sci., 192:315–351.
RecurrentNeuralNetworks-ANaturalModelofComputationbeyondtheTuringLimits
599