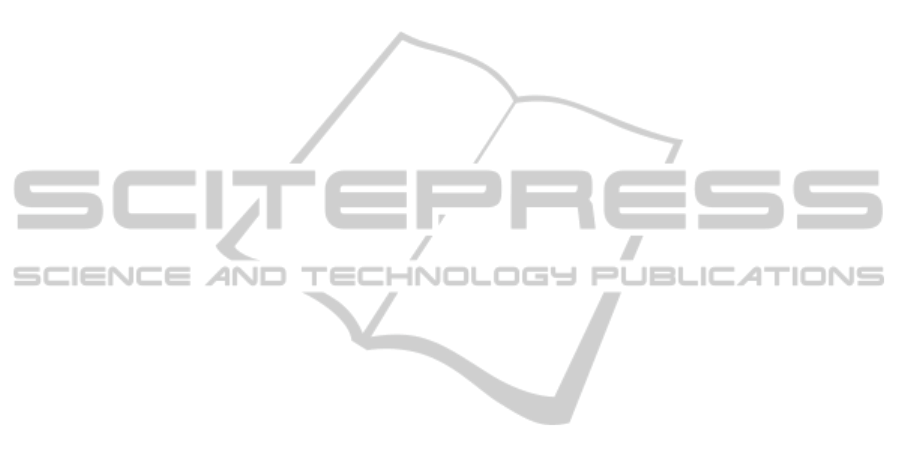
a 6x6 non-uniform 2D-CA and a BN of size N = 36
and were considered as different species. In addition,
the different genome cases evolved in a setup with
only limited computational resources.
The search for a cycle attractor problem showed
that the common genome was not able to show favor-
able results for the development of the architectures.
The separately evolved genome was able to give par-
tially better results; the CA was able to evolve requir-
ing less than half of the resources available.In the case
of transient phase and attractor search, both genome
cases ended up with a similar fitness performance but
the commonly evolved genome consumed consider-
ably fewer resources. In the synchronization task
problem, the commonly evolved genome was able to
evolve the architectures acquiring again less resources
than in the separately evolved genome case.
The construction of a common genome able to de-
velop different computational architectures proved to
be beneficial. There are cases where resources are
not infinitely available or not available at the given
moment. Artificial organisms need to have ways to
overcome such problems if they are to continue to
evolve at all (much like in the nature). Commonly
evolved genomes boosted the evolvability of the ar-
chitectures. In two of the experiments we studied,
it required considerably fewer resources than in the
case of the genome evolved separately. The reason
behind the superiority of the common developmental
genomes is somewhat intuitive. Common genomes in
this configuration, involve two fitness functions (i.e.,
one for node generation and another for connectivity),
defining a set of optimal solutions over each evolu-
tionary cycle. So, we can say that the nodes genome
stands as an ideal source of information for the con-
nectivity genome and ultimately, the development of
the phenotypes.
But there is more to that. It may be that common
developmental genomes are more amenable to devel-
opmental drive (Arthur, 2001). Or, they may have a
positive influence in directing evolution and pushing
the developmental system in phenotypic directions
where it would have been impossible to achieve with
ordinary genomes (i.e., genomes evolved separately
for each architecture at hand). The latter is identi-
fied as developmental bias (Raff, 2000). To conclude,
more research needs to be done towards the identi-
fication of: i. potential relations between mutation
and selection in the underlying genetic process, and
ii. inherent ontogenetic directionalities (i.e., dynam-
ics) for common developmental genomes, during the
stages of evolution.
Closing, the notion of chromosomes in our repre-
sentation, allows us to exploit the genome in a modu-
lar way in a sense that if additional computational ar-
chitectures need to be incorporated in the future and
expressed by the same genome, more chromosomes
can be attached. Changing the way of looking into the
architectures, i.e., instead of looking at them as dif-
ferent species, we could consider them as organs of
a common developing biological entity. That brings
up a case where architectures need to be merged (as
is the case in biological organs). Since the overall
goal of this work is to target more adaptive scalable
systems able of complex computation, the exploration
of these merged computational architectures (i.e., hy-
brid architectures) with the same genome and devel-
opmental model but even further, the ability to shape
the phenotype of our system (phenotypic shaping) as
modules in order to change the dynamic properties of
the entire system, paves the way for promising future
research.
REFERENCES
Antonakopoulos, K. and Tufte, G. (2009). Possibilities and
constraints of basic computational units in develop-
mental systems. In Norsk Informatikkonferanse, NIK-
2009, pages 73–84.
Antonakopoulos, K. and Tufte, G. (2011). A common
genetic representation capable of developing distinct
computational architectures. In IEEE Congress on
Evolutionary Computation (CEC), pages 1264–1271.
Arthur, W. (2001). Developmental drive: an important
determinant of the direction of phenotypic evolution.
Evolution & Development, 3(4):271–278.
Astor, J. C. and Adami, C. (2000). A developmental model
for the evolution of artificial neural networks. Artifi-
cial Life, 6(3):189–218.
Chua, L. and Yang, L. (1988). Cellular neural networks:
theory. IEEE Transactions on Circuits and Systems,
35(10):1257–1272.
Das, R., Crutchfield, J. P., Mitchell, M., and Hanson, J. E.
(1995). Evolving globally synchronized cellular au-
tomata. In Eshelman, L. J., editor, Proceedings of
the Sixth International Conference on Genetic Algo-
rithms, pages 336–343. Morgan Kaufmann Publishers
Inc.
Harding, S. L., Miller, J. F., and Banzhaf, W. (2007).
Self-modifying cartesian genetic programming. In
GECCO ’07: Proceedings of the 9th annual confer-
ence on Genetic and evolutionary computation, pages
1021–1028, New York, NY, USA. ACM.
Kauffman, S. A. (1993). The Origins of Order. Oxford
University Press.
Lindenmayer, A. and Prusinkiewicz, P. (1989). Develop-
mental models of multicellular organisms: A com-
puter graphics perspective. In Langton, C. G., edi-
tor, Artificial Life: Proceedings of an Interdisciplinary
Workshop on the Synthesis and Simulation of Living
IJCCI2012-InternationalJointConferenceonComputationalIntelligence
128