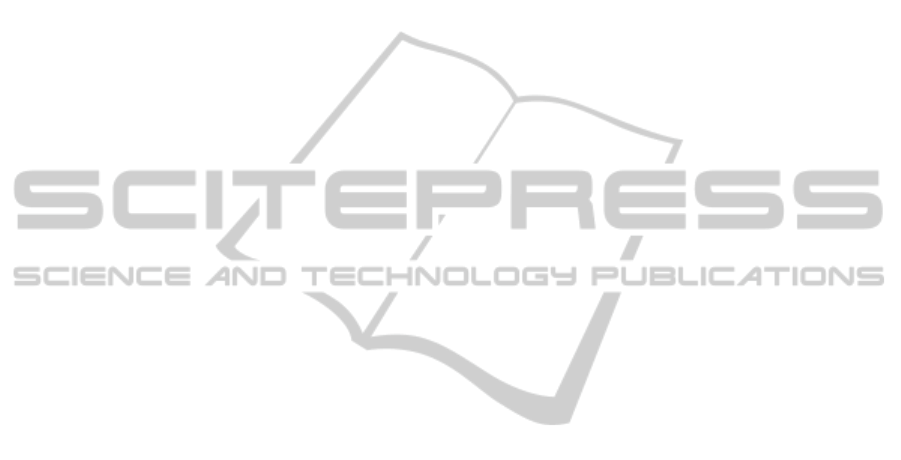
14. K. Anyanwu and A. Sheth, “The ρ Operator : Discovering and Ranking Associations on the
Semantic Web,” ACM SIGMOD Record, vol. 31, no. 4, pp. 42-47, 2002.
15. K. Anyanwu and A. Sheth, “The ρ-Operator : Enabling Querying for Semantic Associations
on the Semantic Web .,” in Proceedings of the 12th International Conference on World
Wide Web, 2003.
16. S. Barton, “Designing Indexing Structure for Discovering Relationships in RDF Graphs,”
in Database, Texts, Specifications and Objects Workshop (DATESO), 2004, pp. 7-17.
17. S. Barton, “Indexing Structure for Discovering Relationships in RDF Graph Recursively
Applying Tree Transformation,” in Semantic Web Workshop at 27th Annual International
ACM SIGIR Conference, 2004, pp. 58-68.
18. G. Wu, J. Li, L. Feng, and K. Wang, “Identifying Potentially Important Concepts and
Relations in an Ontology,” in Proceedings of the 7th International Conference on The
Semantic Web (ISWC ’08), 2008, vol. 5318, pp. 33-49.
19. R. Rada, H. Mili, E. Bicknell, and M. Blettner, “Development and Application of a Metric
on Semantic Nets,” IEEE Transactions on Systems, Man and Cybernetics, vol. 19, no. 1,
1989.
20. P. Hayes, “RDF Model Theory.” [Online]. Available: http://www.w3.org/TR/rdf-mt.
[Accessed: 30-May-2012].
21. O. Lassila and R. Swick, Resource Description Framework: Model and Syntax
Specification. 1999.
22. M. Hildebrand, J. V. Ossenbruggen, and L. Hardman, “An Analysis of Search-based User
Interaction on the Semantic Web,” Information Systems, no. INS–E0706, pp. 1386–3681,
2007.
23. R. C. Backhouse and B. A. Carré, “Regular Algebra Applied to Path-finding Problems,”
Journal of the Institute of Mathematics and Applications, vol. 15, pp. 161-186, 1975.
24. R. E. Tarjan, “Fast Algorithms for Solving Path Problems,” Journal of the ACM, vol. 28,
no. 3, pp. 594-614, Jul. 1981.
25. R. E. Tarjan, “A Unified Approach to Path Problems,” Journal of ACM, vol. 28, no. 3, pp.
577-593, 1981.
26. S. Barton and P. Zezula, “ρ-index – An Index for Graph Structured Data,” in 8th
International Workshop of the DELOS Network of Excellence on Digital Libraries, 2005,
pp. 57–64.
27. S. Warshall, “A Theorem on Boolean Matrices*,” Journal of ACM, vol. 9, no. 1, pp. 11-12,
1962.
28. R. E. Tarjan, “Depth first search and linear graph algorithms,” SIAM Journal of
Computing, pp. 146-160, 1972.
29. P. Walton Purdom, “A transitive closure algorithm,” BIT, vol. 10, pp. 76-94, 1970.
30. Y. E. Ioannidis and R. Ramakrishnan, “Efficient Transitive Closure Algorithms,” in
Proceedings of the Fourteenth International Conference on Very Large Data Bases, 1988,
pp. 382-394.
31. R. Agrawal, “Hybrid Transitive Closure Algorithms,” in 16th International Conference on
Very Large DataBases, 1990, pp. 326-334.
32. S. Barton, “Indexing Graph Structured Data,” Masaryk University, 2007.
33. P. Zezula, G. Amato, F. Debole, and F. Rabitti, “Tree Signatures for XML Querying and
Navigation,” in In 1st International XML Database Symposium, 2003, pp. 149-163.
34. A. Matono, T. Amagasa, M. Yoshikawa, and S. Uemura, “An Indexing Scheme for RDF
and RDF Schema based on Suffix Arrays,” in Proceedings of SWDB’03, The first
InternationalWorkshop on SemanticWeb and Databases, Colocated with VLDB 2003,
2003.
35. R. Agrawal, A. Borgida, and H. V. Jagadish, “Eficient Managemente of Transitive
Relationships in Large Data and Knowledge Bases,” in Proceedings of the 1989
ACMSIGMODi nternational con- ference on Management of data, 1989, pp. 253-262.
53