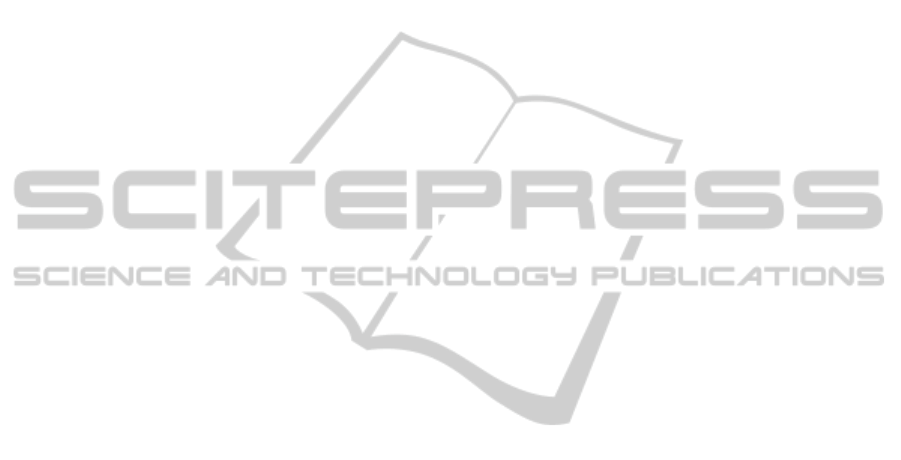
anymore.
Selection of harmonics and topography led to
much more clear improvements. This is to be
expected: this method seeks to adapt the system to
the specificities of each subject. It is noteworthy that
each subject has specific brain responses to SSVEP
(see e.g. Silberstein, et al., 1990), whether
topographically or frequency-wise. It is therefore not
surprising that an adaptation of the system to the
specificities of each subject leads to an improved
classification. The best calibration method between
those two, according to our results, is the selection
of the dominant harmonic in the SSVEP response.
However, the reader should keep in mind that those
two methods are based on very different approaches.
Harmonic selection used 5 minutes of data, whereas
topography selection used only 1 minute of data, but
still led to some significant improvements. Our
results therefore also confirm the interest of
selecting the channels, which was already pointed by
Wang et al. (2006).
ACKNOWLEDGEMENTS
In this project Hovagim Bakardjian was supported
by an International Neuroinformatics Coordinating
Facility grant (june 2011), and by the #3862
fellowship of the Fondation Pierre Gilles de Gennes.
REFERENCES
Bakardjian, H., Tanaka, T., Cichocki, A. 2010,
Optimization of SSVEP brain responses with
application to eight-command Brain–Computer
Interface. Neuroscience Letters, 469(1):34-38.
Bin, G., Gao, X., Yan, Z., Hong, B., Gao, S., 2009. An
online multi-channel SSVEP based brain–computer
interface using a canonical correlation analysis
method. J. Neural Eng. 6 (4) 6: 046002
Friman, O., Volosyak, I., Graser, A., 2007. Multiple
channel detection of steady-state visual evoked
potentials for brain–computer interfaces. IEEE Trans.
Biomed. Eng. 54 (4), 742–750.
Kaplan A. Y., Fingelkurts A. A., Fingelkurts A. A.,
Borisov S. V., Darkhovsky B. S., 2005, Nonstationary
nature of the brain activity as revealed by EEG/MEG:
Methodological, practical and conceptual challenges.
Signal Processing, 85(11):2190-2212.
Krauledat, M., Tangermann, M., Blankertz, B., Muller, K.
R., 2008. Towards zero training for brain–computer
interfacing. PLoS ONE 3(8), e2967.
Lin, Z., Zhang, C., Wu, W., Gao, X., 2006. Frequency
Recognition Based on Canonical Correlation Analysis
for SSVEP-Based BCIs. IEEE Trans. Biomed Eng.
532610-4.
Lotte, F., 2011. Generating Artificial EEG Signals To
Reduce BCI Calibration Time, 5th International
Brain-Computer Interface Workshop
Nicolelis M., 2011, Beyond Boundaries: The New
Neuroscience of Connecting Brains With Machines-
And How It Will Change Our Lives. Times books.
Parini, S., Maggi, L., Turconi, A. C., and Andreoni, G.,
2009. A Robust and SelfPaced BCI System Based on a
Four Class SSVEP Paradigm: Algorithms and
Protocols for a High-Transfer-Rate Direct Brain
Communication. Comput.Intelli. Neurosci ID 864564.
Silberstein, R. B., Schier, M. A., Pipingas, A., Ciorciari,
J., Wood, S. R., Simpson, D. G., 1990. Steady-state
Visually evoked potential topography associated with
a visual vigilance task. Brain Topogr. 3, 337–347.
Shishkin S. L., Nikolaev A. A., Nuzhdin Y. O., Zhigalov
A. Y., Ganin I. P., Kaplan A., Y. 2011. Calibration of
the P300 BCI with the single-stimulus protocol. Proc.
of the Fifth International BCI Conference Graz
University of Technology, Austria. 256-259
Tomita, Y., Gaume, A., Bakardijian, H., Maurice, M.,
Cichocki, A., Yamaguchi, Y., Drefus, G., Vialatte, F.,
2011. Concatenation Method for high temporal
resolution SSVEP-BCI. International Conference on
Neural Computation Theory and Applications.
Vialatte, F. B., Maurice, M., Dauwels, J., Cichocki, A.,
2010. Steady-state visually evoked potentials: Focus
on essential paradigms and future perspectives,
Progress in Neurobiology 90 418–438
Volosyak, I., Malechka, T., Valbuenaand, D., Graser, A.,
2010. A Novel Calibration Method for SSVEPBased
Brain Computer Interfaces.
18th European Signal
Processing Conference, 939-943.
Wang, Y., Wang, R., Gao, X., Hong, B., Gao, S., 2006. A
practical VEP-based brain–computer interface. IEEE
Trans. Neural Syst. Rehabil. Eng. 14 (2), 234–239.
Wolpaw, J. R., Birbaumer, N., McFarland, D. J.,
Pfurtscheller, G., Vaughan, T. M., 2002. Brain–
computer interfaces for communication and control.
Clin. Neurophysiol. 113 (6), 767–791.
Zhang, Y., Jin, J., Qing, X., Wang, B., Wang, X., 2012.
LASSO based stimulus frequency recognition model
for SSVEP BCIs, Biomedical Signal Processing and
Control (7)104– 111
FastBCICalibration-ComparingMethodstoAdaptBCISystemsforNewSubjects
669