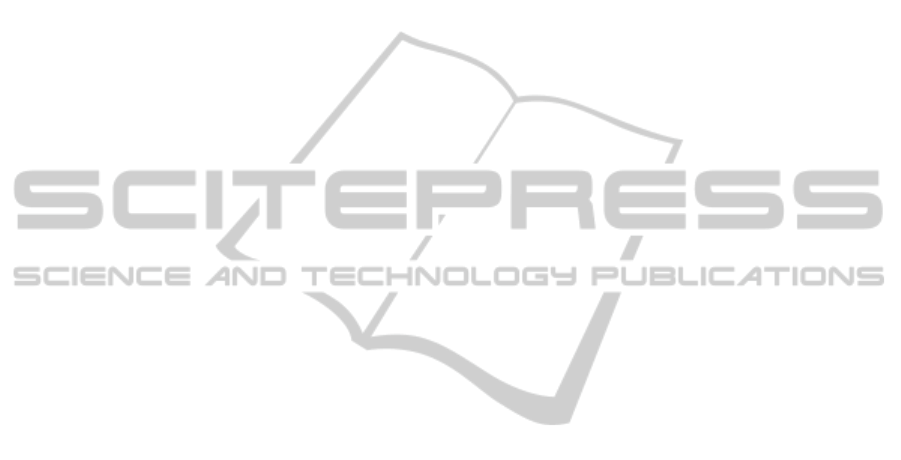
In figure 7 we plot the classification results for the
ten first subjects for error trials and correct trials us-
ing the different preprocessing methods. We can see
that for classification on error trials xDAWN only out-
performs our adaptive algorithm for two subjects and
for one of them results are very bad for any method.
For correct trials spatial filtering clearly outperforms
classification on raw data, however no clear differ-
ence can be drawn between our two methods, with
xDAWN outperforming the adaptive one for half the
subjects.
5 CONCLUSIONS
In this paper we have studied the error-related poten-
tial and its single-trial detection. We have seen that
the ErrP did occur even in high cognitive load con-
ditions and that its latency could be highly variable
from one subject to another. Based on this observa-
tion we have proposed a new algorithm for adaptive
spatial filtering and time window selection based on
xDAWN algorithm. Classification results have shown
that our algorithm performs better than the xDAWN
algorithm and than classification on raw data with an
improvement up to 17% for correct trials (which is the
most important one as we have said before). Thus,
we have developped a new algorithm that allows our
classifier to adapt to ErrP latency variability. This
is of great interest given its high inter-subject vari-
ability. Moreover it is known that ErrP characteris-
tics may vary with experimental and environmental
conditions. ErrP latency could then evolve within an
experiment, using an adaptive filtering method (that
would be updated along the experiment) would allow
consistent classification results along time. In con-
clusion we have shown that using adaptive filters for
ErrP single trial detection could significantly improve
classification results. Moreover we have developped
a new algorithm that could tackle the problem of ErrP
latency variability. Further studies should be done in
this way, as for example to adapt to latency variability
between trials or maybe to find otpimal time windows
for the different ErrP peaks (since it is known that the
ErrP is characterized by a negative peak and a positive
one).
ACKNOWLEDGEMENTS
We are grateful to Dr. Bertrand Rivet for making us
available the code of his xDAWN algorithm and ex-
plaining us his method. We are grateful to the project
ANR OpenVibe.
REFERENCES
Chavarriaga, R. and Mill
´
an, J. (2010). Learning from eeg
error-related potentials in noninvasive brain-computer
interfaces. IEEE Trans Neural Syst Rehabil Eng,
18(4):381–388.
Comon, P. and Jutten, C. (2010). Handbook of Blind Source
Separation: Independent component analysis and ap-
plications. Academic Press.
Dal Seno, B., Matteucci, M., and Mainardi, L. (2010). nline
detection of p300 and error potentials in a bci speller.
O. Computational intelligence and neuroscience.
Falkenstein, M., Hohnsbein, J., Hoormann, J., and Blanke,
L. (1991). Effects of crossmodal divided attention on
late erp components.ii. error processing in choice re-
action tasks. Electroencephalogr. Clin. Neurophysiol.,
78:447–455.
Ferrez, P. and Mill´an, J. (2005). You are wrong! auto-
matic detection of interaction errors from brain waves.
In Proceedings of the 19th International Joint Confer-
ence on Artificial Intelligence.
Ferrez, P. and Mill´an, J. (2007). Eeg-based brain-computer
interaction: Improved accuracy by automatic single-
trial error detection. In Proc. NIPS.
Fukunaga, K. (1990). Introduction to statistical pattern
recognition (2nd ed.). Academic Press Professional,
Inc., San Diego, CA, USA.
Gentsch, A., Ullsperger, P., and Ullsperger, M. (2009). Dis-
sociable medial frontal negativities from a common
monitoring system for self-and externally caused fail-
ure of goal achievement. Neuroimage, 47(4):2023–
2030.
Gray, R. M. (2006). Toeplitz and circulant matrices: A re-
view. Foundations and Trends in Communications and
Information Theory, 2(3):155–239.
Hajcak, G., Moser, J., Holroyd, C., and Simons, R. (2007).
It’s worse than you thought: The feedback negativity
and violations of reward prediction in gambling tasks.
Psychophysiology, 44(6):905–912.
MacKay, D. (1992). Bayesian interpolation. Neural com-
putation, 4(3):415–447.
Pfurtscheller, G. (1981). Central beta rhythm during sen-
sorimotor activities in man. Electroencephalography
and clinical Neurophysiology, 51(3):253–264.
Rivet, B., Souloumiac, A., Gibert, G., and Attina, V.
(2008). P300 speller brain-computer interface: En-
hancement of p300 evoked potential by spatial fil-
ters. In Proceedings of the 16th European Signal
Processing Conference (EUSIPCO-2008), EURASIP,
Lausanne, Switzerland.
Steinhauser, M. and Kiesel, A. (2011). Performance moni-
toring and the causal attribution of errors. Cognitive,
Affective, & Behavioral Neuroscience.
Yeung, N., Holroyd, C., and Cohen, J. (2005). Erp corre-
lates of feedback and reward processing in the pres-
ence and absence of response choice. Cerebral Cor-
tex, 15(5):535.
IJCCI2012-InternationalJointConferenceonComputationalIntelligence
720