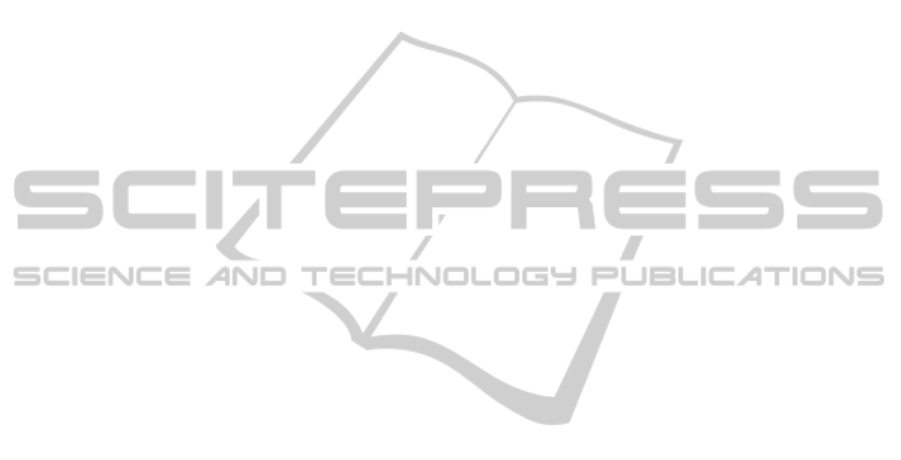
Bruña, R., Poza, J., Gómez, C., Fernández, A., García, M.
and Hornero, R. (2012). Analysis of Spontaneous
MEG Activity in Mild Cognitive Impairment and
Alzheimer’s Disease using Spectral Entropies and
Statistical Complexity Measures. Journal of Neural
Engineering, 9(3), 036007.
Escudero, J., Sanei, S., Jarchi, D., Abásolo, D. and
Hornero, R. (2011). Regional Coherence Evaluation in
Mild Cognitive Impairment and Alzheimer’s Disease
based on Adaptively Extracted
Magnetoencephalogram Rhythms. Physiological
Measurement, 32(8), 1163-1180.
Fernández, A., Hornero, R., Mayo, A., Poza, J., Gil-
Gregorio, P. and Ortiz, T. (2006). MEG Spectral
Profile in Alzheimer’s Disease and Mild Cognitive
Impairment. Clinical Neurophysiology, 117(2), 306-
314.
Fernández, A., Hornero, R., Gómez, C., Turrero, A., Gil-
Gregorio, P., Matías-Santos, J. and Ortiz, T. (2010).
Complexity Analysis of Spontaneous Brain Activity in
Alzheimer’s Disease and Mild Cognitive Impairment:
an MEG Study. Alzheimer Disease and Associated
Disorders, 24(2) 182-189.
Figliola, A. and Serrano, E. (1997). Analysis of
Physiological Time Series using Wavelet Transforms.
IEEE Engineering in Medicine and Biology Magazine,
16(3), 74-79.
Gómez, C., Stam, C. J., Hornero, R., Fernández, A. and
Maestú, F. (2009). Disturbed Beta Band Functional
Connectivity in Patients with Mild Cognitive
Impairment: an MEG Study. IEEE Transactions on
Biomedical Engineering, 56(6), 1683-1690.
Kelen, G. J., Henkin, R., Starr, A. M., Caref, E. B.,
Bloomfield, D. and el-Sherif, N. (1991). Spectral
Turbulence Analysis of the Signal-Averaged
Electrocardiogram and its Predictive Accuracy for
Inducible Sustained Monomorphic Ventricular
Tachycardia. American Journal of Cardiology, 67(11),
965-975.
Morris, J. C. (2012). Revised Criteria for Mild Cognitive
Impairment May Compromise the Diagnosis of
Alzheimer Disease Dementia. Achives of Neurology,
69(6), 700-708.
Percival, D. B. (1995). On Estimation of the Wavelet
Variance. Biometrika, 82(3), 619-631.
Petersen, R. C., Doody, R., Kurz, A., Mohs, R. C., Morris,
J. C., Rabins, P. V., Ritchie, K., Rossor, M., Thal, L.
and Winblad, B. (2001). Current Concepts in Mild
Cognitive Impairment. Archives of Neurology, 58(12),
1985-1992.
Petersen, R. C. and Morris, J. C. (2003). Conceptual
Overview. In Petersen, R. C. (Ed.), Mild Cognitive
Impairment: Aging to Alzheimer’s Disease (pp. 1-14).
New York: Oxford University Press.
Poza, J., Escudero, J., Hornero, R., Fernández, A. and
Sanchez, C. I. (2008a). Regional Analysis of
Spontaneous MEG Rhythms in Patients with
Alzheimer’s Disease using Spectral Entropies. Annals
of Biomedical Engineering, 36(1), 141-152.
Poza, J., Hornero, R., Escudero, J., Fernández, A. and
Gómez, C. (2008b). Analysis of Spontaneous MEG
Activity in Alzheimer’s Disease using Time-
Frequency Parameters. In IEEE-EMBC’08 30th
Annual International Conference of the IEEE
Engineering in Medicine and Biology Society, pp.
5712-5715.
Rampp, S. and Stefan, H. (2007). On the Opposition of
EEG and MEG. Clinical Neurophysiology, 118(8),
1658-1659.
Reitz, C., Brayne, C. and Mayeux, R. (2011).
Epidemiology of Alzheimer Disease. Nature Reviews.
Neurology, 7(3), 137-52.
Stam, C. J. (2010). Use of Magnetoencephalography
(MEG) to Study Functional Brain Networks in
Neurodegenerative Disorders. Journal of the
Neurological Sciences, 289(1-2), 128–134.
Vialatte, F. B., Dauwels, J., Maurice, M., Musha, T. and
Cichocki, A. (2011) Improving the Specificity of EEG
for diagnosing Alzheimer’s Disease. International
Journal of Alzheimer’s Disease, 2011, 259069.
IJCCI2012-InternationalJointConferenceonComputationalIntelligence
726