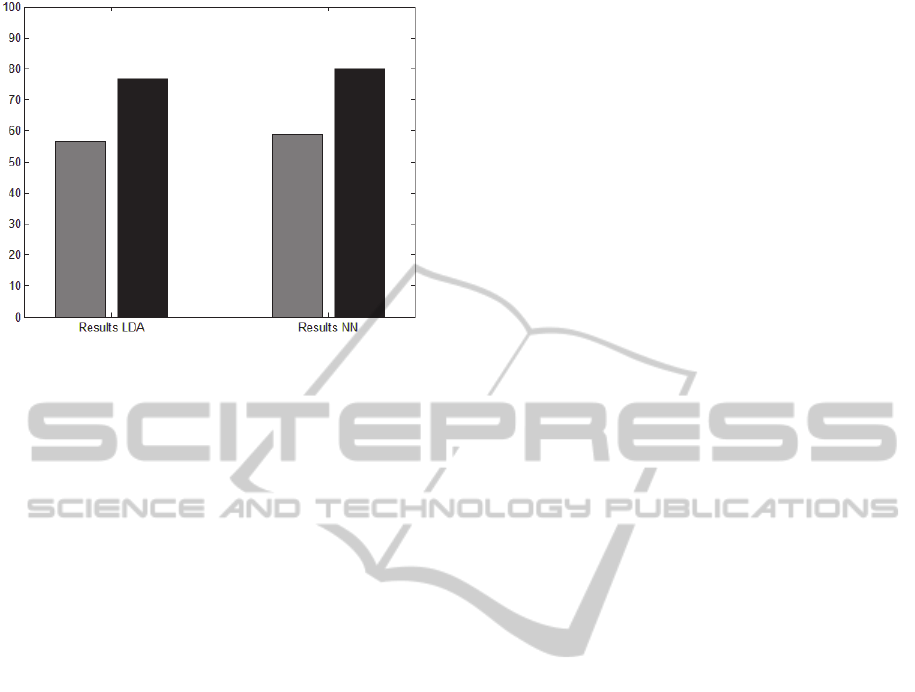
Figure 3: Classification Rates obtained after classifying
the synchrony values with LDA and NN. In both
classifiers, grey bars correspond to results obtained with
raw EEG data, black bars correspond to results obtained
with clean EEG data.
signal preprocessing, a necessary step to be taken
before any kind of EEG signal analysis.
5 CONCLUSIONS
In this paper a procedure for removing artifacts from
EEG data is tested in real data. This method is based
on an EEG decomposing technique, which allows
flexible signal decomposition of the original time
series in different oscillatory modes. The so-
obtained components from each EEG channel have
been analyzed and those that were present in all the
electrodes have been removed from the
reconstructed signal. Then phase synchrony has been
computed for all the subjects, and the obtained
values have been classified using two different
classifiers, linear discriminant analysis and neural
network.
Future work will include the comparison of this
method with ICA-based cleaning procedures (Solé-
Casals et al., 2010), or Wavelet-based cleaning
procedures (Krishnaveni et al., 2006, Vialatte et al.,
2008).
Of course, it is important to point out that the
data set at hand is fairly small. A larger sample size
and a more diverse data set will be used in order to
generalize the results of this study.
ACKNOWLEDGEMENTS
This work has been partially supported by the
Secretaria d’Universitats i Recerca of the
Departament d’Economia i Coneixement of the
Generalitat de Catalunya under the grant 2010BE1-
00772 to Dr. Jordi Solé-Casals; and under a
predoctoral grant from the University of Vic to Mr.
Esteve Gallego-Jutglà, ("Amb el suport de l'ajut
predoctoral de la Universitat de Vic").
REFERENCES
Croft, R. J., Barry, R. J., (2000). Removal of ocular
artefact form the EEG: a review. Neurophysiol
Clin,30, 5-19.
Bishop, C.M., (1995). Neural Networks for Pattern
Recognition. Oxford University Press.
Dauwels, J., Vialatte, F., Musha, T., Cichocki, A. (2010)
A comparative study of synchrony measures for the
early diagnosis of alzheimer’s disease based on EEG.
NeuroImage, 49, 668-693,
Diez, P. F., Mut, V., Laciar, E., Torres, A., Avilla, E.
(2009). Application of the Empirical Mode
Decomposition to the Extraction of Features form
EEG signals for Mental Task Classification. 31
st
Annual International Conference of the IEEE EMBS.
2579-2582.
Duda, R. O., Hart, P. E., Stork, D. (2000). Pattern
Classification. Wiley.
Freeman, J.A., Skapura, D.M. (1991). Neural Networks:
Algorithms, Applications and Programming
Techniques. Addison-Wesley Publishing Company,
Inc. Reading, MA
Fukunaga, K. (1990). Introduction to Statistical Pattern
Classification. Academic Press, San Diego, California,
USA.
Gallego-Jutglà, E., Solé-Casals, J., Rutkowski, T.,
Cichocki, A. (2011). Application of multivariate
empirical mode decomposition for cleaning eye blinks
artifacts from EEG signals. NCTA conference
proceedings, INSTICC 2011.
Huang, N. E., Shen, Z., Long,S. R., Wu, M. C., Shih, H.
H:, Zheng, Q., Yen, N.C., Tung, C. C., Liu, H. H.
(1998). The empirical mode decomposition and the
Hilbert spectrum for nonlinear and non-stationary time
series analysis. Proc. R. Soc. Lond., 495, 2317-2345.
Hush, D. R., Horne, B. G. (1993). Progress in supervised
neural networks. IEEE Signal Processing Magazine,10
(1), pp. 8-39.
Krishnaveni, V., Jayaraman, S., Aravind, S.,
Hariharasudhan, V., Ramadoss, K. (2006). Automatic
Identification and Removal of Ocular Artifacts from
EEG using Wavelet Transform. Measurement Science
Review, Vol. 6, Sec. 2, No. 4.
Lippmann, D. E. (1987). An Introduction to Computing
with Neural Networks. IEEE ASSP Magazine, 3(4),
pp. 4-22
Molla, K. I., Tanaka, T., Rutkowski, T. M., Cichocki, A.,
(2010). Separation of EOG artifacts from EEG singals
using bivariate EMD. Acoustics Speech and Signal
EEGSignalAnalysisviaaCleaningProcedurebasedonMultivariateEmpiricalModeDecomposition
675