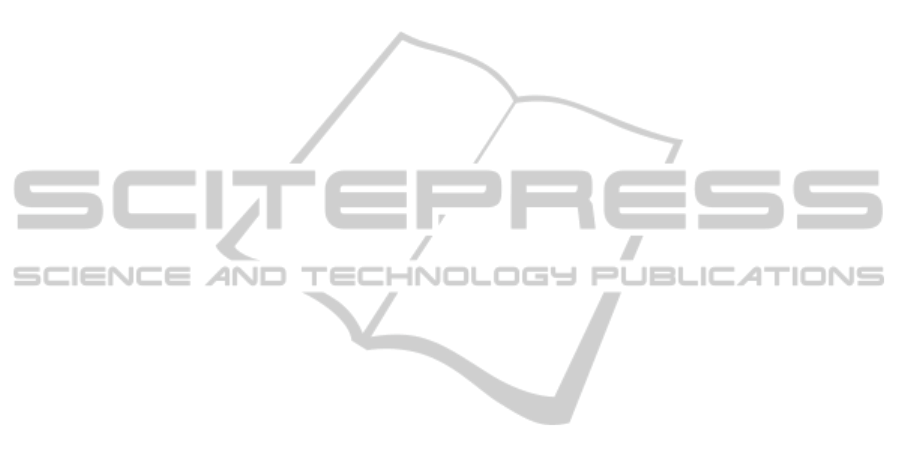
system while R does). Finally one can note that the
ITR follows the same evolution (for growing number
of repetitions) before and after integration of our er-
ror correction system. If one aims at improving the
ITR it seems that the best strategy would be RC (ie,
replacement under conditions).
4.3 Utility Metric
Once again, the utility metric (U) does not necessarily
increase with growing number of repetitions. For low
performances of our ED (T
e
= 0.6 and T
c
= 0.7) there
does not seem to be one particular strategy clearly
outperforming the others. However one can see that
strategy R gives very poor results. For higher perfor-
mances of our ED (T
e
≥ 0.8 and T
c
≥ 0.9) it seems that
strategy A is slightly better than the other but this is
not significant. However one can note that with these
performances each strategy brings high improvement.
4.4 Discussion
In this paper we have simulated the integration of the
ErrP as an error-correction system in a P300 speller
BCI . We have presented different strategies of inte-
gration and studied their impact on the BCI perfor-
mances. Using different types of performance mea-
sures we have seen that each strategy had some ad-
vantages and some disadvantages. The overall best
strategy seems to be the strategy of canceling the erro-
neous order when it is detected since it gives the best
results in terms of classification accuracy and of util-
ity measure. However the measure used in most stud-
ies to assess the performance of multiclass BCIs is the
ITR. For the ITR it seems that the best strategy is the
strategy of replacing the erroneous command by the
one obtaining the second best score under condition.
Thus, one can not state for a best strategy. However
what can be noted is that for high performances of
our error-detection system every strategy allows im-
provement of our system for any type of performance
measure. Moreover for T
e
= 0.8 and T
c
= 0.9 we get a
mean improvement (over subject) of 11% of the clas-
sification accuracy , of 9% of the ITR and of 10% of
the utility metric (each time for the best integration
strategy). Thus we have seen that the ErrP could be
used as an error-correction system in a multiclass BCI
and that, even if it did not bring results as performant
as for a two class BCI, one can get an improvement
of around 10% of our system which is very encourag-
ing. However we have also seen that the integration
strategy should be carefully chosen according to the
systems characteristics and to what one wants to im-
prove.
ACKNOWLEDGEMENTS
We are grateful to the project ANR OpenVibe.
REFERENCES
Blankertz, B., Muller, K., Curio, G., Vaughan, T., Schalk,
G., Wolpaw, J., Schlogl, A., Neuper, C., Pfurtscheller,
G., Hinterberger, T., and et al. (2004). The bci compe-
tition 2003: progress and perspectives in detection and
discrimination of eeg single trials. Biomedical Engi-
neering, IEEE Transactions on, 51(6):1044–1051.
Bollon, J. and Chavarriaga, R. and Mill
´
an, J. and Bessiere,
P. (2009). Eeg error-related potentials detection with
a bayesian filter.
Chavarriaga, R., Ferrez, P. W., and Mill
´
an, J. (2007). To err
is human: Learning from error potentials in braincom-
puter interfaces. Int Conf Cognitive Neurodynamics,
pages 777–782.
Dal Seno, B., Matteucci, M., and Mainardi, L. (2010).
The utility metric: a novel method to assess the over-
all performance of discrete brain computer interfaces.
Neural Systems and Rehabilitation Engineering, IEEE
Transactions on, 18(1):20–28.
Falkenstein, M., Hohnsbein, J., Hoormann, J., and Blanke,
L. (1991). Effects of crossmodal divided attention on
late erp components.ii. error processing in choice re-
action tasks. Electroencephalogr. Clin. Neurophysiol.,
78:447–455.
Farwell, L. A. and Donchin, E. (1988). Talking off the
top of your head: toward a mental prosthesis utiliz-
ing event-related brain potentials. Electroencephalogr.
Clin. Neurophysiol, 70(6):510–523.
Ferrez, P. and Mill
´
an, J. (2005). You are wrong! automatic
detection of interaction errors from brain waves. In
Proceedings of the 19th International Joint Confer-
ence on Artificial Intelligence.
Ferrez, P. and Mill
´
an, J. (2007). Eeg-based brain computer
interaction: Improved accuracy by automatic single-
trial error detection. In Proc. NIPS.
Ferrez, P. and Mill
´
an, J. (2008). Simultaneous real-time de-
tection of motor imagery and error-related potentials
for improved bci accuracy. In Proc 4th Intl. Brain
Computer Interface Workshop and Training Course.
Gehring, W., Goss, B., Coles, M., Meyer, D., and Donchin,
E. (1993). A neural system for error detection and
compensation. Psychol. Sci., 4:385–390.
Gentsch, A., Ullsperger, P., and Ullsperger, M. (2009). Dis-
sociable medial frontal negativities from a common
monitoring system for self-and externally caused fail-
ure of goal achievement. Neuroimage, 47(4):2023–
2030.
MacKay, D. (1992). Bayesian interpolation. Neural com-
putation, 4(3):415–447.
Rivet, B., Souloumiac, A., Attina, V., and Gibert, G. (2009).
xdawn algorithm to enhance evoked potentials: Appli-
cation to brain computer interface. Biomedical Engi-
neering, IEEE Transactions on, 56(8):2035–2043.
Steinhauser, M. and Kiesel, A. (2011). Performance moni-
toring and the causal attribution of errors. Cognitive,
Affective, & Behavioral Neuroscience.
Closed-loopingaP300BCIusingtheErrP
737