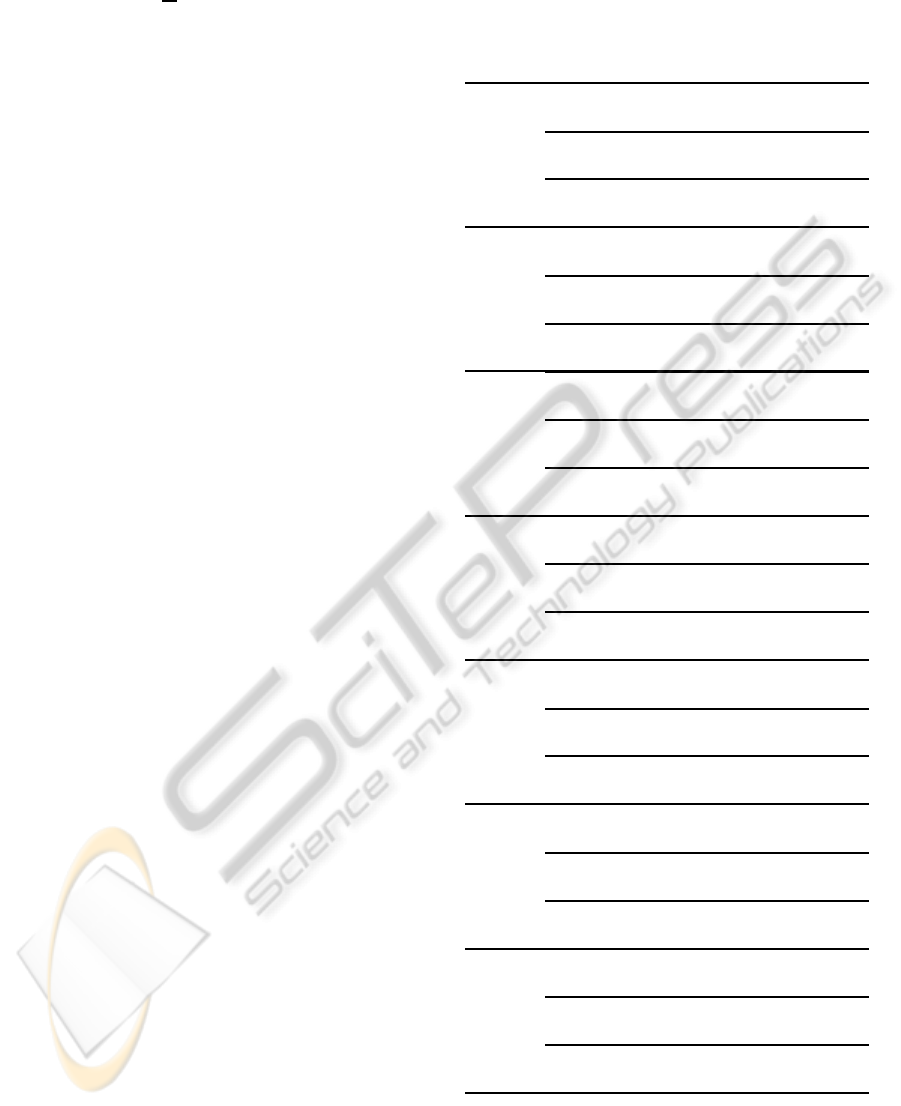
MAE =
1
N
N
∑
i=1
|y
i
− ˆy
i
| (14)
where y
i
is the observed output and ˆy
i
is the output
predicted by the model.
Another model is included in Table 2 (Min.),
which refers to the minimum MAE of the ELM net-
work inside the whole committee of ELMs, that is, the
member of the committee which presented the mini-
mum MAE. In this way, we obtain a reference value
that indicates if the committee provides best results as
a whole than the best ELM network that takes part of
the committee.
Table 2 shows the median value of the 50 experi-
ments that were considered for each configuration of
ELM-committee. Moreover, only three of the five val-
ues of the different number of neurons in the hidden
layer tested are presented for the sake of simplicity.
This values are 20, 60, 100 initial number of neurons,
which corresponds with first, second and third rows of
each method respectively (Table 2). The columns cor-
responds to the several number of committee mem-
bers tested, which were varied from 20 to 100 with
increments of 20, as mentioned previously.
To summarize the information contained in Table
2, a comparison of three methods has been carried
out. To do such comparison, the value of the MAE
in each method, for each number of committee tested
(columns) and each number of hidden neurons tested,
is compared. The result of this comparison shows
that, in general terms, the proposed method outper-
form the other two methods. Specifically, the 71.43%
of the times the proposed method won, the 9.52% of
the times the linear committee won and the 19.05% of
the times they tied. The third method, the member of
the committee which presented the minimum MAE,
never was the best method.
In order to illustrate the performance of the pro-
posed algorithm, graphically, compared with the lin-
ear comittee of ELMs and with the member of the
committee which presented the minimum error, we
present Figures 1 and 2. These figures show the re-
sults for Abalone (Figure 1) and Autoprice (Figure 2)
data sets. Each figure presents four cases that cor-
respond to several committees. The first case corre-
sponds to a committee composed of 20 members, the
second case to one of 40 members, the third case to
one of 60 members and, finally, the latter case corre-
sponds to a committee composed of 80 members.
Notice that for Abalone data set, Figures 1a and
1b the bayesian regularized ELM committee presents
always the minimum MAE; the second best method
is the linear committee of ELM. However, Figures 1c
and 1d show that the proposed method presents al-
ways the minimum MAE again, but in these cases (60
Table 2: Performance in terms of MAE in the validation set
of the proposed method (Bayes.), in comparison with the
results of the linear committee (LS) and the member of the
committee which presented the minimum MAE.
Data set Method C1 C2 C3 C4 C5
0,311 0,309 0,306 0,310 0,309
Min. 0,309 0,307 0,311 0,308 0,312
0,376 0,365 0,364 0,364 0,364
Abalone 0,282 0,288 0,307 0,324 0,371
LS 0,285 0,299 0,318 0,350 0,388
0,319 0,347 0,394 0,425 0,486
0,271 0,271 0,267 0,266 0,262
Bayes. 0,265 0,263 0,268 0,269 0,273
0,290 0,289 0,300 0,305 0,309
0,497 0,491 0,494 0,493 0,495
Min. 0,425 0,425 0,427 0,427 0,425
0,407 0,407 0,407 0,407 0,407
Add10 0,408 0,398 0,394 0,390 0,384
LS 0,375 0,361 0,348 0,339 0,331
0,353 0,336 0,323 0,313 0,301
0,408 0,398 0,394 0,389 0,383
Bayes. 0,375 0,361 0,348 0,338 0,331
0,353 0,336 0,323 0,313 0,301
0,311 0,309 0,306 0,310 0,309
Min. 0,309 0,307 0,311 0,308 0,312
0,376 0,365 0,364 0,364 0,364
Auto 0,282 0,288 0,307 0,324 0,371
LS 0,285 0,299 0,318 0,350 0,388
0,319 0,347 0,394 0,425 0,486
0,271 0,271 0,267 0,266 0,262
Bayes. 0,265 0,263 0,268 0,269 0,273
0,290 0,289 0,300 0,305 0,309
0,444 0,448 0,444 0,438 0,424
Min. 0,769 0,768 0,710 0,720 0,732
2,159 2,043 1,791 1,758 1,773
Autoprice 0,391 0,459 0,692 5,373 1,850
LS 0,571 0,776 1,273 6,883 1,791
0,822 0,599 0,587 0,504 0,505
0,359 0,356 0,356 0,335 0,341
Bayes. 0,416 0,399 0,410 0,379 0,397
0,822 0,560 0,587 0,515 0,505
0,480 0,477 0,479 0,477 0,477
Min. 0,463 0,462 0,463 0,462 0,461
0,460 0,459 0,460 0,459 0,459
Delta elev. 0,459 0,457 0,457 0,456 0,456
LS 0,455 0,454 0,455 0,455 0,455
0,453 0,454 0,455 0,455 0,456
0,459 0,456 0,456 0,454 0,453
Bayes. 0,454 0,453 0,453 0,452 0,451
0,453 0,453 0,453 0,452 0,452
0,439 0,440 0,440 0,441 0,438
Min. 0,415 0,408 0,410 0,403 0,406
0,422 0,412 0,420 0,419 0,416
Housing 0,374 0,376 0,383 0,390 0,388
LS 0,330 0,331 0,348 0,346 0,361
0,309 0,317 0,327 0,341 0,359
0,364 0,351 0,348 0,339 0,327
Bayes. 0,319 0,308 0,310 0,302 0,300
0,301 0,286 0,289 0,285 0,287
0,753 0,755 0,754 0,752 0,750
Min. 0,702 0,704 0,705 0,702 0,700
0,669 0,669 0,671 0,669 0,669
Parkinson 0,704 0,678 0,658 0,647 0,631
LS 0,633 0,616 0,605 0,592 0,589
0,594 0,581 0,574 0,565 0,560
0,705 0,679 0,659 0,648 0,633
Bayes. 0,633 0,617 0,606 0,592 0,589
0,593 0,582 0,575 0,565 0,558
and 80 committee members) the second best method
is Min. (the member of the committee which pre-
sented the minimum MAE).
For Autoprice data set, Figures 2a and 2b show
BayesianRegularizedCommitteeofExtremeLearningMachine
113