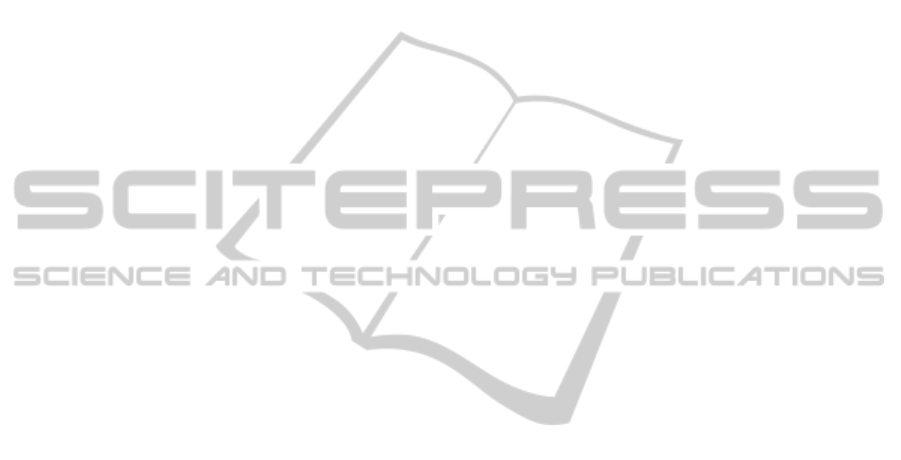
presented in contiguity with an alarmed
demonstrator, the unconditioned stimulus. More
importantly, there are properties of socially acquired
predator avoidance (e.g., the intensity of the
unconditioned response increases with that of the
unconditioned stimulus, and the fact that there is
preferential learning about particular types of
stimuli) that provide support of the idea that socially
acquired behaviours are mediated by individual
learning processes and not by independent social
learning mechanisms.
This line of research is complementary to the
work done in imitation in the Artificial Intelligence
community. Such approach has used social learning
theories from psychology to develop adaptive agents
that learn from others by observing their behaviour.
In particular, (Mataric, 1994) has used vicarious
reinforcement to deal with the Credit Assignment
Problem.
5 CONCLUSIONS
It is our contention that the proposal outlined in this
position paper will strengthen the connection
between the study of computational and biological
systems. In particular, the approach we advocate will
contribute to answering the question of how
psychological concepts such as motivation, attention
and intention can be modelled in artificial organisms
to affect adaptive behavioural modifications and
control.
Reinforcement learning algorithms have
successfully been applied to simple domains in areas
such as navigation robotics, manufacturing, and
process control. More powerful algorithms will, no
doubt, benefit larger scenarios in industrial
applications such as telecommunications systems,
air traffic control, traffic and transportation
management, information filtering and gathering,
electronic commerce, business process management,
entertainment, and medical care.
REFERENCES
Alonso, E., (2002). AI and Agents: State of the Art. AI
Magazine, 23, 25-29.
Bowling, M. and Veloso, M., (2001), Rational and
convergent learning in stochastic games. In
Proceedings of the Seventeenth International Joint
Conference on Artificial Inelligence (IJCAI-2001),
pages 1021-1026, Seattle, WA.
Claus, C. and Boutilier, C., (1998), The dynamics of
reinforcement learning in cooperative multiagent
systems. In Proceedings of the Fifteenth National
Conference on Artificial Intelligence, pages 746-752.
Greenwald, A., Hall, K., and Serrano, R., (2002),
Correlated-Q learning. In NIPS Workshop on
Multiagent Learning.
Griffin, A. S., (2004), Social learning about predators: A
review and prospectus. Learning & Behavior 32(1),
131-140.
Hu, J. and Wellman, M., (2001), Learning about other
agents in a dynamic multiagent system. Journal of
Cognitive Systems Research, 2:67-79.
Littman, M. L., (2001), Friend-or-foe Q-learning in
general-sum games. In Proceedings of the Eighteen
International Conference on Machine Learning.
Littman, M. L., (1994), Markov games as a framework for
multi-agent reinforcement learning. In Proceedings of
the 11
th
International Conference on Machine
Learning, 157-163.
Mataric, M., (1994), Learning to behave socially. In
Proceedings of the Third International Conference on
Simulation and Adaptive Behavior.
Sen , S., Sekaran, M., and Hale, J., (1994), Learning to co-
ordinate without sharing information. In Proceedings
of the Twelfth National Conference on Artificial
Intelligence, 426-431, Seattle, WA.
Shoham, Y., Powers, R., and Grenager, T., (2003), Multi-
Agent Reinforcement Learning: a critical survey.
Technical Report.
Stone, P. and Veloso, M., (2000), Multiagent Systems: A
Survey from a Machine Learning Perspective,
Autonomous Robots, Volume 8( 3), 345-383.
Suematsu, N. and Hayashi, A., (2002), A multiagent
reinforcement learning algorithm using extended
optimal response. In Proceedings of the Fifteenth
International Joint Conference on Artificial
Intelligence (IJCAI-97), 370-377.
Sutton, R. S. and Barto, A. G., (1998), Reinforcement
Learning: An Introduction, Cambridge, MA: The MIT
Press.
Wang, X. and Sandholm, T., (2002), Reinforcement
learning to play an optimal Nash equilibrium in team
Markov games. In Advances in Neural Information
Processing Systems (NIPS-2002).
ICAART2013-InternationalConferenceonAgentsandArtificialIntelligence
146