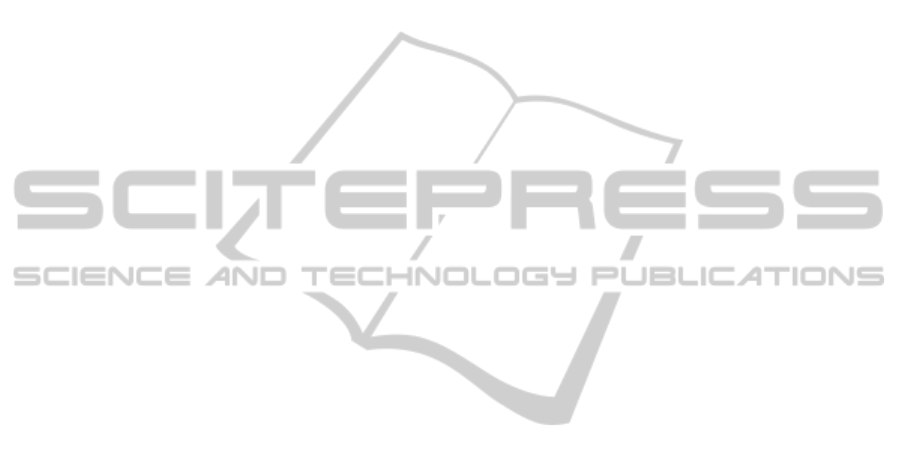
described methods and two clinicians. Mean
duration is not shown because it has similar values
as the median duration, where the median is more
robust. Detection is subjective from rater to rater,
what always leads to a difference between the values
between the raters. In other words, there is no golden
standard for the description of bursts and IBIs.
Nevertheless, a clear distinction between bursts
and IBIs can be found by looking at two features:
average amplitude and average energy operator.
Namely, the average amplitude for IBIs is smaller
than the average amplitude for bursts. This is always
the case, for all methods and for all raters. This is
also true for values of the average energy operator.
IBIs contain less energy than bursts.
Although burst% was considered as the
statistically most significant parameter for
correlations between all raters (Palmu et al., 2010),
representation of this feature in Figure 4 shows that
the correlation between values obtained by the
NLEO algorithm and the clinicians is not that high.
Especially the line length method gives a high
correlation.
Maximum IBI duration has been reported to
decrease as the postmenstrual age increases
(Hayakawa et al., 2001). In the present study, the
maximum IBI decreases from the first measurement
to the second measurement two weeks later for
patients 1 and 4 (Figure 4). Not only a decrease of
the maximum IBI duration, but also a decrease of
the median IBI value has a good prognostic value for
good neurological outcome. Values for this
parameter decrease as well for patients 1 and 4.
By checking differences between values obtained
for clinical and algorithm detection, it can be said
that the developed algorithm approximates well the
values of the parameters after the clinical detection.
5 CONCLUSIONS
The developed algorithm is a successful strategy to
detect patterns in the premature EEG, like bursts and
the intervals between them. The automated analysis
of EEG provides possibilities to look over a longer
period of time and over various records at different
points in time. Also, assessment of the evolution
over time of the unique characteristics of the EEG is
very valuable. Consequently, good approximation of
clinical features is of high importance. Thereby, it
aggregates the experience and trained eyes of more
clinical doctors and researchers in an overarching
model. Future work will focus on fine-tuning the
algorithm based on a larger dataset of validated EEG
segments. Additionally, more features and their
clinical relevance have to be explored. Such a
detection algorithm would dramatically improve the
overall assessment in the NICU for EEG diagnosis.
ACKNOWLEDGEMENTS
Research supported by
Research Council KUL: GOA MaNet, PFV/10/002
(OPTEC), IDO 08/013 Autism, several PhD/postdoc
& fellow grants;
Flemish Government: FWO: PhD/postdoc grants,
projects: G.0427.10N (Integrated EEG-fMRI),
G.0108.11 (Compressed Sensing) G.0869.12N
(Tumor imaging); IWT: TBM070713-Accelero,
TBM070706-IOTA3, TBM080658-MRI (EEG-
fMRI), TBM110697-NeoGuard, PhD Grants; IBBT;
MDV is supported by an Alexander von Humboldt
stipend.
Belgian Federal Science Policy Office: IUAP P7/
(DYSCO, `Dynamical systems, control and
optimization', 2012-2017); ESA AO-PGPF-
01, PRODEX (CardioControl) C4000103224.
EU: RECAP 209G within INTERREG IVB NWE
programme, EU HIP Trial FP7-HEALTH/ 2007-
2013 (n° 260777).
REFERENCES
Accardo, A., Affinito, M., Carrozzi, M., et al., 1996. Use
of the fractal dimension for the analysis of
electroencephalographic time series. In Biological
Cybernetics, 77: p. 339-350.
De Vos, M., Deburchgraeve, W., Cherian, P.J., et al.,
2011. Automated artifact removal as preprocessing
refines neonatal seizure detection. Clinical
Neurophysiolog, 122, p. 2345-2354.
Esteller, R., Echauz, J., Tcheng, T., et al., 2001. Line
length: An efficient feature for seizure onset detection.
Papers from 23
rd
annual International Conference of
the IEEE Engineering in Medicine and biology
Society, Istanbul, Turkey.
Hayakawa, M., Okumura, A., Hayakawa, F., et al., 2001.
Background electroencephalographic (EEG) activities
of very preterm infants born at less than 27 weeks
gestation: a study on the degree of continuity. In
Archives of Disease in Childhood – Fetal and
Neonatal Edition, 84: p. 163–167.
Jennekens, W., Ruijs, L. S., Lommen, C. M. L., et al.,
2011. Automatic burst detection for the EEG of the
preterm infant. In Physiological Measurement, 32: p.
1623–1637.
Le Bihannic, A., Beauvais, K., Busnel, A., et al., 2011.
Prognostic value of EEG in very premature newborns.
BIOSIGNALS2013-InternationalConferenceonBio-inspiredSystemsandSignalProcessing
110