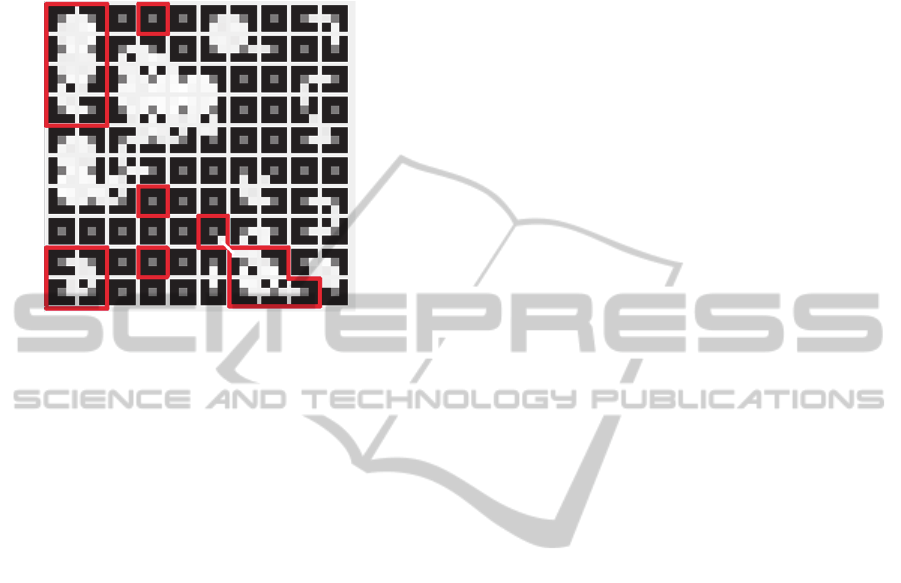
considered as statistically significant. However, it
shows us, that the way we decided to solve the
problem with ERPs detection which affects method
described in Svoboda et al. (2008), may be right.
Figure 6: Neuron weights similarity in a two-dimensional
map with 100 neurons with manually highlighted clusters
which are related to Gabor atoms which approximate ERP
P3 waveform.
Looking at results given in Table 1, it does not
matter which of two feature vectors presented in this
paper will be used. The only difficulty which affects
the described method is that clusters which
approximate (or partially approximate) ERP
waveforms must be marked manually by an expert.
In the future, we will use the proposed method in
ERP detection algorithm based on MP to prove, that
this method can improve the reliability of ERPs on a
statistically significant level.
ACKNOWLEDGEMENTS
The work was supported by the UWB grant SGS-
2010-038 Methods and Applications of Bio- and
Medical Informatics and by the European Regional
Development Fund (ERDF), Project "NTIS - New
Technologies for Information Society", European
Centre of Excellence, CZ.1.05/1.1.00/02.0090.
REFERENCES
Luck, S. J., (2005). An Introduction to the Event-Related
Potential Technique. Cambridge: The MIT Press.
Rondik, T. and Ciniburk, J., (2011). Comparison of
Various Approaches for P3 Component Detection
Using Basic Methods for Signal Processing. 4th
International Conference on Biomedical Engineering
and Informatics (BMEI), 700 - 704, New York: IEEE.
Picton, T. W., (1992). The p300 wave of the human event-
related potential. Journal of Clinical Neurophysiology,
9, 456-479.
Svoboda, J., Mautner, P. and Moucek, R., (2008).
Detection of ERP using Matching Pursuit Algorithm.
Xth International conference on cognitive
neuroscience, 226, Istanbul.
Ferrando, S. E., Kolasa, L. A. and Kovacevic, N., (2002).
Algorithm 820: A Flexible Implementation of
Matching Pursuit for Gabor Functions on the Interval.
ACM Transactions on Mathematical Software, 28(3),
2002, 337-353.
Wann, C. D. and Thomopoulos, S. C. A., (1997). A
Comparative Study of Self-organizing Clustering
Algorithms Dignet and ART2. Neural Networks,
10(4), 737–753.
Lotte, F., Congedo, M., Lecuyer, A., Lamarche, F. and
Arnaldi, B., (2007). A Review of Classification
Algorithms for EEG-based Brain-Computer Interfaces.
Journal of Neural Engineering, 4.
Pradhan, N., Sadasivan, P. K. and Arunodaya, G. R.,
(1996). Detection of seizure activity in EEG by an
artificial neural network: A preliminary study.
Computers and Biomedical Research, 29, 303–313.
Gotman, J. and Wang, L., (1991). State-dependent spike
detection: Concepts and preliminary results.
Electroencephalography and Clinical
Neurophysiology, 79(1), 11–19.
Squires, N. K., Squires, K. C. and Hillyard, S. A., (1975).
Two varieties of long-latency positive waves evoked
by unpredictable auditory stimuli in man.
Electroencephalography and Clinical
Neurophysiology , 38(4), 387-401.
Niedermeyer, E. and Lopes da Silva, F., (2004).
Electroencephalography: Basic Principles, Clinical
Applications, and Related Fields. Philadelphia:
Lippincott Williams & Wilkins.
Durka, P. J., (2007). Matching pursuit. Retrieved April 22,
2010, from: http://www.scholarpedia.org/article/
Matching_pursuit.
HEALTHINF2013-InternationalConferenceonHealthInformatics
314