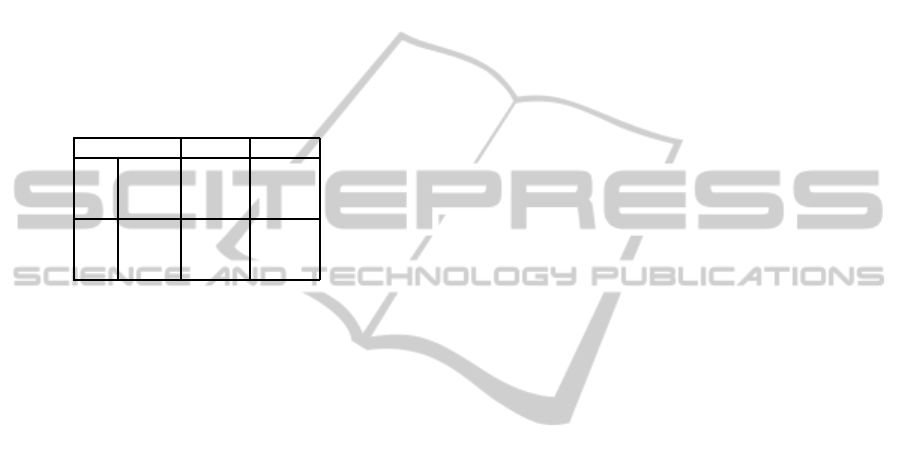
temporal window are shown, besides the mean, for all
subjects of the temporal window size.
For DWS
1
the lower boundary t
lo
was the highest
for subjects 10 and 14, removing approximately 98
ms immediately after the stimulus. This means that
this signal segment does not contain yet enough dis-
criminative information to be useful. For the upper
boundary t
up
, the lowest value was obtained by sub-
ject 22, removing a little more than half of the original
window, because the window was consider until 488
ms to perform the analysis.
Table 2: Results obtained by DWS
1
and DWS
2
on the tem-
poral window selection. t
lo
and t
up
are respectively the
lower and the higher bounds in ms over all subjects and
channels.
DWS
1
DWS
2
t
lo
min 1 1
mean 20 23
max 98 305
t
up
min 488 277
mean 848 820
max 1000 1000
Please recall that, for the algorithm DWS
2
, the
window selected is different for every channel. The
lower boundary t
lo
was the highest for subject 19
in channel 9 (Oz), suggesting that the information
recorded in the first 305 ms does not contain enough
discriminative information. The lowest upper bound-
ary t
up
, obtained for subject 3 at time 277 ms for cha-
nnel 3 (Cz), which is strange because this channel is
usually the one that contains more information. One
explanation is that the electrode is not well-fixed to
the skull or maybe it was moving at the time of the
recording.
6 CONCLUSIONS
Wavelets techniques are becoming an increasingly
important exploration tool in BCI, providing temporal
and spectral information of the signals under study. In
this paper we have introduceda new method to exploit
the correlated information among channels based on
the wavelet-based semblance, measure that was ini-
tially developed to be used in Geosciences. This tech-
nique removes the noise and establishes an appropri-
ate temporal window adapted to each subject. Fur-
thermore, the method is quite general and easy to im-
plement, been possible to be used with others brain
signals.
We empirically demonstrate using the P300
speller application that our method is useful to remove
undesirable component of the signals, improving the
letter accuracy for most of the subjects under study.
One advantage of this method is the ability to adapt to
each subject showing more stability compared with
XDAWN. Also, as the number of features is reduced
by the window selection algorithm, it is likely that the
speed of the classifier may be improved. Despite its
advantages, further studies are needed in order to de-
termine the best threshold parameters.
REFERENCES
Antoniadis, A. (2007). Wavelet methods in statistics: Some
recent developements and their applications. Statistics
Surveys, 1:16–55.
Bougrain, L., Saavedra, C., and Ranta, R. (2012). Finally,
what is the best filter for p300 detection? Proceedings
of the 3rd TOBI Workshop, pages 53–54.
Cooper, G. (2009). Wavelet-based semblance filtering.
Computers & Geosciences, 35(10):1988–1991.
Cooper, G. and Cowan, D. (2008). Wavelet based sem-
blance analysis. Computers & Geosciences, 34(2):95–
102.
Donoho, D. and Johnstone, I. M. (1995). Adapting to un-
known smoothness via wavelet shrinkage. Journal of
the American Statistical Association, 90:1200–1224.
Farwell, L. and Donchin, E. (1988). Talking off the top of
your head: toward a mental prosthesis utilizing event-
related brain potentials. Electroencephalogr Clin Neu-
rophysiol, 70(6):510–523.
Hoffmann, U., Vesin, J.-M., and Ebrahimi, T. (2006). Spa-
tial filters for the classification of event-related poten-
tials. in Proceedings of the 14th European Symposium
on Artificial Neural Networks (ESANN).
Mallat, S. (2008). A wavelet tour of signal processing. Aca-
demic Press, 3rd edition.
Quiroga, R. and Garcia, H. (2003). Single-trial event-
related potentials with wavelet denoising. Clinical
Neurophysiology, 114(2):376–390.
Rivet, B., Souloumiac, A., Attina, V., and Gibert, G. (2009).
xdawn algorithm to enhance evoked potentials: Ap-
plication to brain computer interface. IEEE Trans.
Biomed. Engineering, 56(8):2035–2043.
Schalk, G., McFarland, D., Hinterberger, T., Birbaumer, N.,
and Wolpaw, J. (2004). BCI2000: a general-purpose
brain-computer interface (bci) system. IEEE Transac-
tions on Biomedical Engineering, 51(6):1034–1043.
Torrence, C. and Compo, G. (1998). A practical guide to
wavelet analysis. Bulletin of the American Meteoro-
logical Society, 79(1):61–78.
Wolpaw, J., Birbaumer, N., McFarland, D., Pfurtscheller,
G., and Vaughan, T. (2002). Brain-computer inter-
faces for communication and control. Clinical Neuro-
physiology, 113(6):767–791.
Yong, Y., Hurley, N., and Silvestre, G. (2005). Single-trial
EEG classification for brain-computer interface using
wavelet decomposition. In European Signal Process-
ing Conference, EUSIPCO 2005.
Wavelet-basedSemblanceforP300Single-trialDetection
125