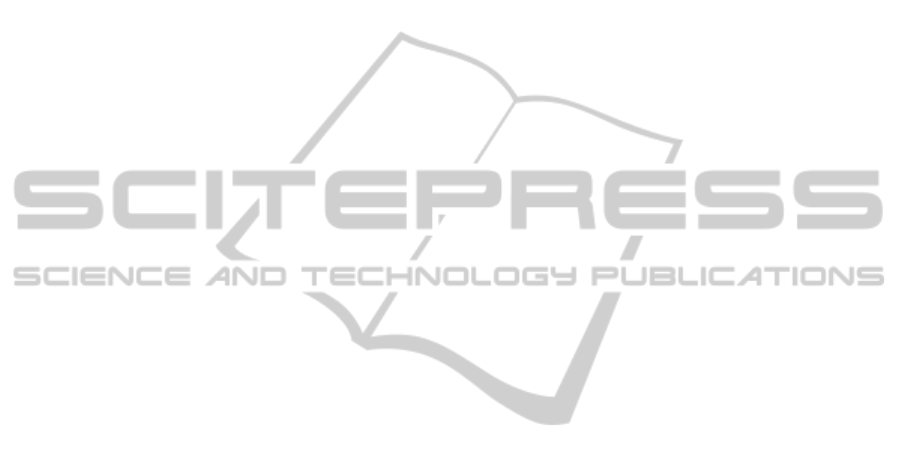
block probability of reporting a significant p-value.
While FDR-based strategies are robust in
dependence scenarios, the same is not true for SGoF,
which crucially depends on the correct estimation of
the variance associated to the number of discoveries.
In most practical situations with dependent tests, the
final number of discoveries reported by SGoF will
be too liberal, because it will be based on an
underestimated variance (Owen, 2005). To solve this
issue, de Uña-Álvarez (2012) introduced a
correction of SGoF method to deal with dependent
tests. This correction is based on the beta-binomial
extension of the binomial model, which arises when
the number of successes S among the n trials is
conditionally distributed as a binomial given the
probability of success π, which is a random variate
following a beta distribution. The beta-binomial
model has three parameters: the number of trials n,
the mean probability of success p=E(π), and the
pairwise correlation between the outcomes
τ=Var(π)/p(1-p). The mean and the variance of the
beta-binomial model are given respectively by
E(S)=np and Var(S)=np(1-p)(1+(n-1)τ); this shows
that, by putting τ>0, the beta-binomial model allows
for a variance larger than binomial. See Johnson and
Kotz (1970) for further details and illustrations of
the model.
More specifically, given the set of n p-values
u
1
,…,u
n
coming from the n nulls being tested, BB-
SGoF (from beta-binomial SGoF) correction starts
by computing the binary sequence X
i
=I(u
i
≤γ),
i=1,…,n. Then, by assuming that there are k
independent blocks of p-values of sizes n
1
,…,n
k
(with n
1
+…+n
k
=n), the number of successes sj
within each block j is computed. Here, X
i
=1 is called
‘success’. Each s
j
is assumed to be a realization of a
beta-binomial variable with parameters (n
j
,p,τ). In
this setting, p represents the average proportion of p-
values falling below γ, which under the complete
null is just γ, and τ is the within-block correlation
between two outcomes X
i
and X
j
. Estimation of p
and τ is performed by maximum-likelihood, and the
lower bound of a 100(1-α)% interval for the excess
of significant cases n(p-γ) is reported; this bound is
the number of effects declared by BB-SGoF (which
weakly controls FWER at level α in this manner).
Therefore, BB-SGoF follows the same spirit of
SGoF method, but some preliminary estimation of
the p-values’ dependence structure is performed to
correct for it. This correction may have a big impact
in the researcher’s decision; for example, de Uña-
Álvarez (2012) illustrates for two real datasets that
ordinary SGoF rejects 10% (Hedenfalk data) or
about 4% (Diz data) nulls more than BB-SGoF, and
that BB-SGoF rather than SGoF should be applied
due to significant correlation.
Simulations in de Uña-Álvarez (2012) for n=500
and n=1000 tests reported the mean and standard
deviation of the number of rejections for SGoF-type
methods, as well as the family-wise rejection rate
(which reduces to the FWER and FDR under the
complete null of no effects); these simulation results
showed that BB-SGoF is able to control FWER at
level α even when the number of blocks k is
unknown (which is the usual situation in practice),
provided that some conservative criterion in the
estimation of k is used. Besides, this conservative
criterion did not result in a great loss of power
(compared to the ‘benchmark’ method based on the
true value of k). Ordinary SGoF performed badly
otherwise, being unable to control for dependences
(as expected). However, the simulation study
reported in the referred paper has some limitations.
First, no computation of the FDR in the presence of
effects was made, neither results on the methods’
power were reported. This was because of the
employed simulation procedure, which does not
allow for distinguishing between true and non-true
nulls. Also because of this, comparison to the
Benjamini-Hochberg (BH) FDR-controlling
procedure was not possible. Second, the simulated
model was a beta-binomial, and therefore the
simulations are useless to know how the beta-
binomial approach will work in other scenarios with
blocks of dependent tests. The simulation study in
the present work aims to overcome these limitations.
The rest of the paper is organized as follows. In
Section 2 we describe the simulated setting. In
Section 3 we report the results of our simulation
study, and we summarized them in a number of
relevant findings. A final discussion is reported in
Section 4.
2 SIMULATED SETTING
Having in mind the study of Hedenfalk data (see e.g.
de Uña-Álvarez, 2012), we simulated n=500 or
n=1000 2-sample t-tests for comparison of normally
distributed ‘gene expression levels’ in two groups A
and B with sizes 7 and 8 respectively. The
proportion of true nulls (i.e. genes equally
expressed) Π
0
was 1 (complete null), 0.9 (10% of
effects), or 0.67 (33% of effects). Mean was always
taken as zero in group A, while in group B it was μ
for 1/3 of the effects and –μ for the other 2/3 of
effects, with μ=1 (weak effects), μ=2 (intermediate
effects), or μ=4 (strong effects). Random allocation
BIOINFORMATICS2013-InternationalConferenceonBioinformaticsModels,MethodsandAlgorithms
94