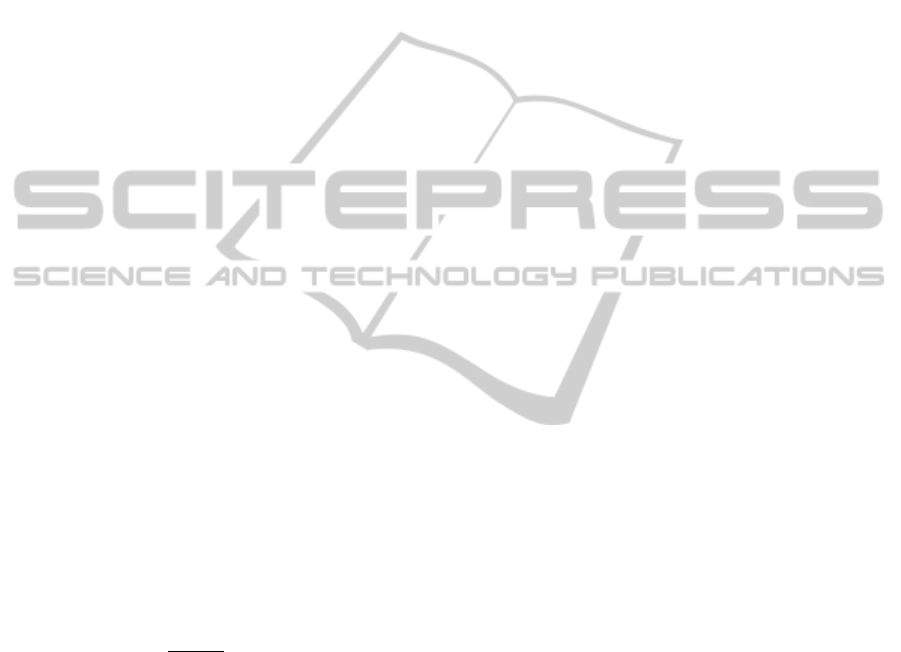
2 METHODS
2.1 Dataset and Preprocessing
Our database consisted of 14 7-day Holter record-
ings from patients with persistent AF. The orthogo-
nal X, Y, Z leads were acquired using a commercially
available device (Lifecard CF
T M
, Del Mar Reynolds).
Since QRST cancellation of the entire recordings
was not possible due to computational restrictions, the
first 5 min of each hour throughout the 7 days were
analyzed. The residual ECG was obtained using spa-
tiotemporal QRST cancellation. Furthermore, QRST-
related residuals were replaced by spline interpolation
if they exceeded ±6 times the standard deviation of
the residual.
Despite preprocessing, 5 recordings still had sig-
nificant QRS-related residuals and had to be excluded
since f-waves characterization could not be done.
Thus, the remaining dataset consisted of 9 recordings.
2.2 Morphology, Frequency and
Irregularity Parameters
The morphology of f-waves is characterized in indi-
vidual leads using PCA information (Jackson, 1980).
For this purpose, the parameter r
3
is introduced,
which exploits the short-term temporal redundancy in
the AF signal. It is obtained by first dividing each 5-
min segment into 1-s segments, and then computing
the sample covariance matrix from the resulting 300
segments. The resulting eigenvectors are arranged in
order of decreasing eigenvalues λ
i
. The variance con-
centrated to the 3 largest eigenvalues can be assessed
by the percentage of their cumulative normalized vari-
ance r
3
, i.e.,
r
3
=
∑
3
i=1
λ
i
∑
300
i=1
λ
i
· 100. (1)
This parameter is computed for each 5-min segment
throughout the 7-day recordings, and thus provides an
hourly characterization. Higher values of r
3
reflect
more regular and organized f-wave morphology since
the 3 first PCs explain most of the variance, and vice
versa. An advantage with r
3
is that no assumption is
required on an underlying signal model.
The AF frequency, which reflects the repetition
rate of the f-waves, plays a central role when analyz-
ing atrial activity. Several studies have demonstrated
a significant correlation between AF frequency and
the likelihood of spontaneous or drug-induced AF
termination (Mainardi et al., 2009; Bollmann et al.,
2006; Nilsson et al., 2006). The AF frequency trend
is here estimated by short-term Fourier analysis of the
residual signal. In order to reduce the influence of
intermittent noise, HMM-based tracking is employed
which yields an optimal trend from a sequence of ob-
served AF frequencies (Sandberg et al., 2008). AF
frequency is determined every 5 min to be compara-
ble with the other parameters.
SampEn holds certain properties which are suit-
able for the study of physiological signals (Richman
and Moorman, 2000). SampEn is the negative natural
logarithm of the conditional probability that two se-
quences which are similar for m points remain similar
for m + 1 points. In order to compute SampEn, the
embedded dimension m, i.e., the length of the vec-
tors to be compared, and the noise filter threshold r
need to be specified. For the AF signal different val-
ues for m and r were estudied in (Alcar
´
az and Rieta,
2008), accordingly SampEn is computed for every 5-
min segment, using m = 2 and r = 0.2 · the standard
deviation of the signal segment.
2.3 Circadianity Analysis
Circadian rhythms are assessed in 7-day recordings
using hourly values of the parameters under study.
The data sequence can be represented by a temporal
regression model known as the cosinor model (Bing-
ham et al., 1982), defined by
y
n
= M + A
0
cos(2π f
0
t
n
+ ϕ
0
) + e
n
, (2)
where M denotes the rhythm-adjusted mesor (i.e.,
mean value), A
0
the fitted cosine amplitude, f
0
the
fundamental frequency (set to 24 hours) and ϕ
0
the
acrophase (i.e., the lag from a defined reference time
point to the crest time in the cosine curve fitted to
the data). The random variable e
n
corresponds to the
residuals, this is, the difference between the observed
sample y
n
and the value provided by the estimated re-
gression model ˆy
n
. The least squares method is ap-
plied to determine the regression parameters.
The significance of a circadian rhythm is de-
termined here by means of a bootstrap hypothesis
test (Efron and Tibshirani, 1993). The circadian
model ( ˆy
n
) is compared against a model consisting
of the mesor component (M). The mean square er-
ror between the signal and each model (E
ˆy
n
, E
M
) is
computed to obtain the difference between the resid-
uals of the two models (∆E = E
M
− E
ˆy
n
). The sta-
tistical relevance of ˆy
n
versus M is assessed using a
paired bootstrap hypothesis test. B random resam-
plings with replacement of residuals are made to ob-
tain ∆E for each resampling (B = 2500). A suitable
statistical hypothesis test contrasts the null hypothesis
that the models have the same unexplained variance
(∆E = 0) against the alternative hypothesis that both
Seven-dayAnalysisofAtrialFibrillationandCircadianRhythms
21