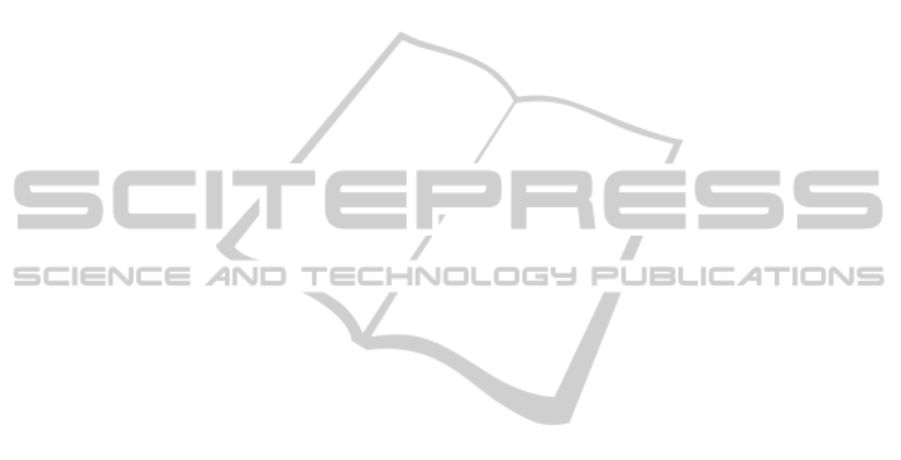
priate for the target group.
The results show that the TEBRA system is able
to deal with the huge variance in spatial and tempo-
ral execution of the task: the system mostly gives se-
mantically correct prompts and is able to assist users
through the entire task of brushing teeth.
7 CONCLUSIONS
In this paper, we describe a novel cognitive assis-
tive technology: the TEBRA system aims to assist
users with cognitive disabilities in the complex task
of brushing teeth. We use a structured approach based
on IU analysis by utilizing the results in the develop-
ment of the TEBRA system. We tackle the huge vari-
ance in spatial and temporal execution of the task: in
the hierarchical recognition framework, we abstract
from tracking objects or the user’s hands. Instead, we
infer behaviors based on the environmental state of
objects. We deal with the temporal variance in task
execution in our planning framework: we apply a Fi-
nite State Machine and a dynamic timing model to
allow for different velocities of users. We showed in
a study with regular users that the TEBRA system is
able to monitor the user’s progress in the task and pro-
vide appropriate prompts to the user if necessary. The
user’s reactions show that prompting modalities are
meaningful to a minimum degree with regard to reg-
ular users. Due to the structured approach using IU
analysis, the TEBRA system can be easily extended
to different tasks.
Future work includes technical enhancements of
the system: we aim to recognize user behaviors more
robustly by improving the classification mechanisms.
We will enhance the dynamic timing model by learn-
ing the parameters on a larger set of training data. We
aim to evaluate the TEBRA system with persons with
cognitive disabilities in the near future.
ACKNOWLEDGEMENTS
The authors would like to thank the inhabitants and
caregivers of Haus Bersaba for their cooperation. This
work was funded by the German Research Founda-
tion (DFG), Excellence Cluster 277 “Cognitive Inter-
action Technology“. Special thanks to Simon Schulz
from the CITEC Central Lab Facilities for integrating
the sensor module into the toothbrush.
REFERENCES
Bauchet, J., Giroux, S., Pigot, H., Lussier-Desrochers, D.,
and Lachapelle, Y. (2008). Pervasive assistance in
smart homes for people with intellectual disabilities:
a case study on meal preparation. Assistive Robotics
and Mechatronics, 9(4):42–54.
Giroux, S., Bauchet, J., Pigot, H., Lussier-Desrochers, D.,
and Lachappelle, Y. (2008). Pervasive behavior track-
ing for cognitive assistance. In PETRA’08, pages 1–7.
Hoey, J., Ploetz, T., Jackson, D., Monk, A., Pham, C., and
Olivier, P. (2011). Rapid specification and automated
generation of prompting systems to assist people with
dementia. Pervasive and Mobile Computing, 7(3):299
– 318.
Hoey, J., Poupart, P., Bertoldi, A. v., Craig, T., Boutilier, C.,
and Mihailidis, A. (2010). Automated handwashing
assistance for persons with dementia using video and
a partially observable markov decision process. Com-
puter Vision and Image Understanding, 114:503–519.
Moore, D., Essa, I., and Hayes, M. (1999). Object Spaces:
Context Management for Human Activity Recogni-
tion. In AVBPA’99.
Najjar, M., Courtemanche, F., Hamam, H., Dion, A., and
Bauchet, J. (2009). Intelligent recognition of activities
of daily living for assisting memory and/or cognitively
impaired elders in smart homes. Ambient Computing
and Intelligence, 1(4):46–62.
Nguyen, N., Phung, D., Venkatesh, S., and Bui, H. (2005).
Learning and detecting activities from movement tra-
jectories using the hierarchical hidden markov model.
In CVPR’05.
Patterson, D. J., Liao, L., Gajos, K., Collier, M., Livic,
N., Olson, K., Wang, S., Fox, D., and Kautz, H. A.
(2004). Opportunity knocks: A system to provide
cognitive assistance with transportation services. In
Ubicomp’04, pages 433–450.
Peters, C., Hermann, T., and Wachsmuth, S. (2011). Pro-
totyping of an automatic prompting system for a resi-
dential home. In RESNA/ICTA 2011 Conf. Proc. (on-
line).
Peters, C., Hermann, T., and Wachsmuth, S. (2012). User
behavior recognition for an automatic prompting sys-
tem - a structured approach based on task analysis. In
ICPRAM’12, pages 162–171.
Pollack, M. E., Brown, L. E., Colbry, D., McCarthy,
C. E., Orosz, C., Peintner, B., Ramakrishnan, S., and
Tsamardinos, I. (2003). Autominder: an intelligent
cognitive orthotic system for people with memory im-
pairment. Robotics and Autonomous Systems, 44(3–
4):273–282.
Pusiol, G., Patino, L., Bremond, F., Thonnat, M., and
Suresh, S. (2008). Optimizing Trajectories Clustering
for Activity Recognition. In MLVMA’08, Int. Work-
shop on Machine Learning for Vision-based Motion
Analysis.
Ryu, H. and Monk, A. (2009). Interaction Unit Analy-
sis: A New Interaction Design Framework. Human-
Computer Interaction, 24(4).
TEBRA:AnAutomaticPromptingSystemforPersonswithCognitiveDisabilitiesinBrushingTeeth
23