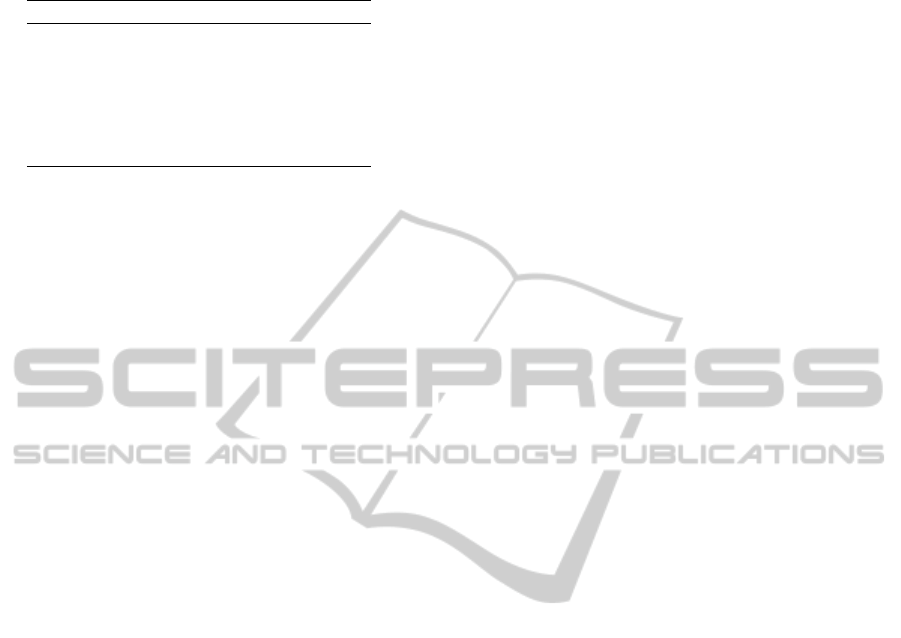
Table 1: Comparison of rest and tilt parameters. *p<0.05,
**p<0.001.
Rest Tilt
ˆ
α 0.52 ± 0.24 0.51 ± 0.30
ˆ
τ
min
1
(s) 0.37 ± 0.09 0.38 ± 0.10
ˆ
τ
min
2
(s) 0.46 ± 0.12 0.47 ± 0.09
ˆ
τ
p,1
(s) 0.23 ± 0.20 0.11 ± 0.10 **
ˆ
τ
p,2
(s) 0.24 ± 0.31 0.16 ± 0.19 *
ˆ
λ (Hz) 6.25 ± 0.58 6.32 ± 0.61 *
5 CONCLUSIONS
We have proposed an updated AV node model in
which i) the characterization of the AV nodal path-
ways is made more detailed using two different pa-
rameters representing the prolongation of related re-
fractory periods, ii) the number of pathways is deter-
mined by the BIC, and iii) the arrival rate is corrected
to take into account there is a minimum time inter-
val between successive impulses arriving to the AV
node. The updated model leads to better estimation
of the PDF when two peaks with different width are
to be modeled, and also the most parsimonious model
is selected (choosing between single or dual pathway
model). Considering physiological aspects, our re-
sults indicate that tilting is associated with significant
changes in AV conduction that are well-described by
the model and reflected by shortening of both τ
p,1
and
τ
p,2
during adrenergic activation. Thus, the present
AV node model is adequate for studying and describ-
ing the functional characteristics of AV conduction in
AF patients.
REFERENCES
Climent, A. M., Guillem, M. S., Zhang, Y., Millet, J.,
and Mazgalev, T. N. (2011). Functional mathemati-
cal model of dual pathway AV nodal conduction. Am.
J. Physiol. Heart. Circ. Physiol., 300:H1393–H1401.
Cohen, R. J., Berger, R. D., and Dushane, T. (1983). A
quantitative model for the ventricular response during
atrial fibrillation. IEEE Trans. Biomed. Eng., 30:769–
781.
Corino, V., Sandberg, F., Mainardi, L., and S
¨
ornmo, L.
(2011). An atrioventricular node model for analysis
of the ventricular response during atrial fibrillation.
IEEE Trans. Biomed. Eng., 58:3386–3395.
Corino, V., Sandberg, F., Mainardi, L., and S
¨
ornmo, L.
(2012). Non-invasive, robust estimation of refractory
period of atrioventricular node during atrial fibrilla-
tion. In Proceedings of the VII Workshop on Biosignal
Interpretation.
Fuster, V., Ryd
´
en, L. E., Cannom, D. S., Crijns, H. J.,
Curtis, A. B., Ellenbogen, K. A., et al. (2006).
ACC/AHA/ESC 2006 guidelines for the management
of patients with atrial fibrillation: A report of the
American College of Cardiology/American Heart As-
sociation task force on practice guidelines and the Eu-
ropean Society of Cardiology committee for practice
guidelines. Circulation, 114(7):e257–e354.
Jørgensen, P., Sch
¨
afer, C., Guerra, P. G., Talajic, M., Nat-
tel, S., and Glass, L. (2002). A mathematical model
of human atrioventricular nodal function incorporat-
ing concealed conduction. Bull. Math. Biol., 64:1083–
1099.
Larsen, M. L. and Kostinski, A. B. (2009). Simple
dead-time corrections for discrete time series of non-
Poisson data. Meas. Sci. Technol., 20:1–10.
Lian, J., M
¨
ussig, D., and Lang, V. (2006). Computer mod-
eling of ventricular rhythm during atrial fibrillation
and ventricular pacing. IEEE Trans. Biomed. Eng.,
53:1512–1520.
Mangin, L., Vinet, A., Page, P., and Glass, L. (2005).
Effects of antiarrhythmic drug therapy on atrio-
ventricular nodal function during atrial fibrillation in
humans. Europace, 7:S71–S82.
Mazgalev, T., Dreifus, L., Ininuma, H., and Michelson, E.
(1984). Effects of the site and timing of atrioventric-
ular nodal input on atrioventricular conduction in the
isolated perfused rabbit heart. Circulation, 70:748–
759.
Ogden, R. (1997). Essential Wavelets for Statistical Appli-
cations and Data Analysis. Birkh
¨
auser, Boston.
Sandberg, F., Stridh, M., and S
¨
ornmo, L. (2008). Frequency
tracking of atrial fibrillation using hidden Markov
models. IEEE Trans. Biomed. Eng., 55:502–511.
StatisticalModelingofAtrioventricularNodalFunctionduringAtrialFibrillation-AnUpdate
29