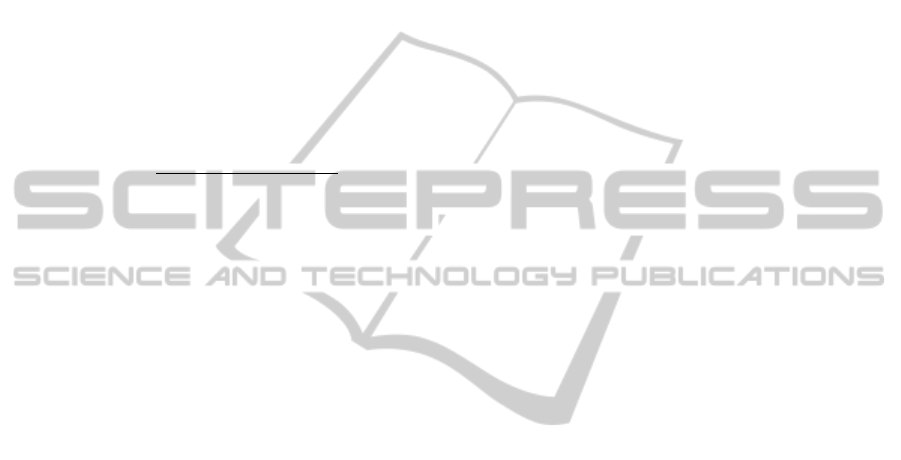
well as environmental factors. Therefore a subset of
genes is likely to have similar or nearly identical
pattern of gene expression if probed under similar
conditions. Thus, when global gene expression data
in the form of EST expression levels is compared
between similarly prepared EST libraries (e.g. non-
normalised preparations) from the identical tissues,
the Pearson correlation between such libraries is
likely to be close to "+1", for many genes.
Previously, some ~1,500 transcripts were
identified as tissue specific from investigations using
CGAP’s cDNA DGED (Milnthorpe and Soloviev,
2012). This was optimised further by summing
together all the libraries in each tissue to make a
super-library. All possible Pearson correlations were
calculated between all super-libraries (equation 1).
,
∑
∑
∑
∑
(1)
Where x and y are the total EST counts for the
transcript concerned in super-libraries X and Y, m
and n are the mean EST counts across all transcripts
in super-libraries X and Y, and Correl(X,Y) is the
calculated Pearson Correlation Coefficient between
the two super-libraries X and Y.
Higher correlation value means higher inter-
tissue correlation and is undesirable; ideally all
correlations should be equal to "0". Hence sum of
squares values were calculated from the correlations.
1
(2)
Where Correl is the calculated Pearson Correlation
coefficient between two super-libraries and S is the
sum of squares value for the correlations between all
possible pairs of super-libraries.
To optimise the initial selection and decrease the
overall inter-tissue correlations individual genes
were then removed from the super-libraries and the
sum of squares values were recalculated. The gene
whose removal resulted in the lowest overall inter-
tissue correlations was permanently removed and the
iteration steps were repeated again. The decrease in
inter-tissue correlations slowed shortly before the
1,000th gene was removed. The remainder included
high-quality tissue-specific markers and were
retained. These were optimised further to improve
intra-tissue correlation between libraries from the
same tissue using the original libraries (data not
shown). This produced an EST expression matrix
containing 244 genes. We have earlier reported a
few applications of the matrix for elucidation of
tissue identity (Milnthorpe and Soloviev, 2012).
In order to investigate the robustness of our
quality control approach based on the developed
matrix, here we used modelled data to simulate
small expression datasets. These were generated
from the expression data, by proportionally reducing
the reported EST counts and rounding any fractional
values to the nearest whole count each time. This
continued until each library ceased to present any
ESTs mapping onto the 244 marker transcripts or
ceased to be identified as a positive match for the
tissue from which it was created. Using this
approach we scaled down expression datasets and
compared all of the model libraries with the original
libraries by calculating the correlation values for the
genes in our matrix. Virtually every library
continues to correlate well with the tissue of origin
until the very last EST mapping onto one of the
transcripts is removed (a typical outcome is shown
in Figure 1 for pancreas). Furthermore, the majority
of the scaled down libraries remain identifiable until
total EST counts fall below 10 – 50 which is equal to
some of the smallest libraries in CGAP’s database.
Our results for pancreas are summarised in Table
1 which details results for each of the original
libraries used and model data sets. The initial and
final numbers of total ESTs are shown and the
correlation values are indicated for each pair.
Remarkably, the final counts across all transcripts in
each library which still yield positive intra-tissue
correlation are below 100 ESTs for all but 3 libraries
tested and are below 10 ESTs for 15 out of 33
libraries tested. The tissue typing quality does not
change dramatically. These findings show that the
EST expression matrix can be used to confirm the
identity of virtually any library including small
libraries, making it a very robust method for the
quality control of expression libraries. Similar
results were obtained for all other tissues tested so
far: lung, placenta, retina and testis, data not shown.
3 DISCUSSION
We created an EST expression matrix based on
carefully selected marker genes and demonstrated its
potential for quality control of EST data and
elucidation of the tissue identity of uncharacterised
libraries and cancer staging. The model libraries
described here were analysed using the matrix. The
findings presented in Figure 1 and Table 1 and the
results for the other tissues show that the EST matrix
can be used to identify the tissue of origin for
libraries containing as few as 2 ESTs. These findings
show that tissue-specific gene expression can be
used as a quality control method, which substantially
OptimisationandValidationofaMinimumDataSetfortheIdentificationandQualityControlofESTExpressionLibraries
279