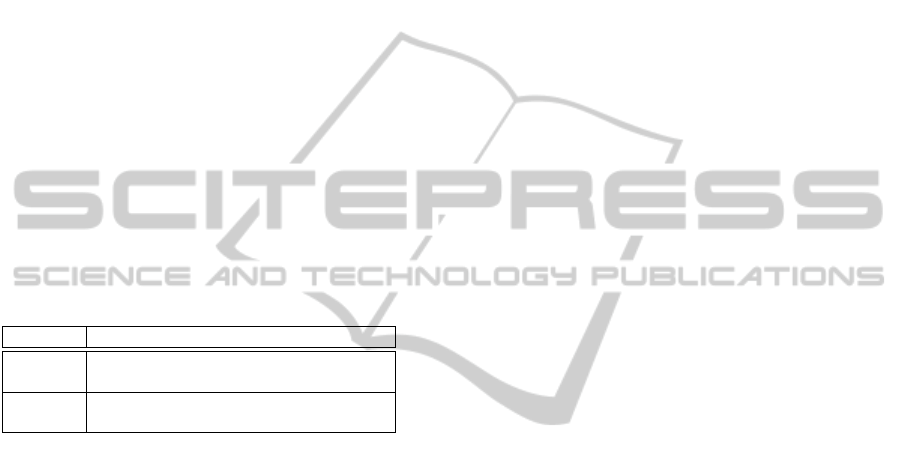
(bottom) depicts relevance values obtained for each
DB1 channel at the patient 1 by RCE method and
stochastic relevance. Note that SRA measures suc-
cessfully selects the channels with more seizure ac-
tivity (T1, T3 and T5) showing high relevance values
close to 1. All results show that our method provide
a better medical interpretability about epileptic region
compared to the weights computed by RCE method.
Shortest weights values corresponding to EEG deriva-
tions are discarded in this figure.
4.2 EEG Segment Classification
Table 2 shows classification results achieved in DB1
and DB2 databases by RCE and stochastic variability
(SRA). As seen from Table 2, there is an improve-
ment in terms of classifier performance when we use
stochastic relevance, DB1 achieves 95.38% and DB2
achieves 94.60% in accuracy values, compare to RCE
that present accuracy values for DB1 (93.60%) and
DB2 (92.10%).
Table 2: Comparison in classification performance of RCE
and SRA by method by k-NN algorithm.
Method DB Acc( %) Sens(%) Spec(%)
RCE
DB1 93.60 93.60 93.60
DB2 92.10 93.85 90.35
SRA
DB1 95.38 95.90 96.23
DB2 94.60 94.60 94.32
Note that values in classifier performance are
close each to other, even though DB2 is more con-
taminated by artifacts than DB1, which has a pre-
processing to eliminate ocular movements (Guerrero-
Mosquera and Navia-Vazquez, 2012). The proposed
model is then stable for EEG classification problems
in presence of noise.
5 CONCLUSIONS AND FUTURE
WORK
A method for classification and channel selection for
EEG multi-channel data with epilepsy is proposed.
This method, based on Exponentially Damped Sinu-
soidal model (EDS) and Stochastic Relevance Anal-
ysis (SRA), is simple and do not requires high com-
putational cost in training than others methods pro-
posed at the state-of-the-art (Peters et al., 2001; Yang
et al., 2012; Duun-Henriksen et al., 2012). Achieved
results show this method is an alternative for extract-
ing relevant EEG rhythms and selecting EEG chan-
nels with epileptic activity. Results validated by ex-
perts through visual inspection and scalp topographic
map, show that the approach also provides a better
medical support in epileptic region localization.
Future work includes: comparing our approach with
other seizure detection methods proposed in the state-
of-the-art; exploring other brain abnormalities such
as Alzheimer, sleep disorders and dementia; exploit-
ing the features for epileptogenic region analysis, and
consider the feasibility of our method to seizure an-
ticipation.
ACKNOWLEDGEMENTS
This research is carried out under the grant Centro de
Investigaci
´
on e Innovaci
´
on de Excelencia ARTICA,
sponsored by COLCIENCIAS and Convocatoria de
apoyo a tesis de posgrado DIMA 2011, Universidad
Nacional de Colombia, project 13753. Also thanks
to Instituto de Epilepsia y Parkinson del Eje Cafetero
(Pereira,Colombia) with the EEG data collection.
REFERENCES
De Clercq, W., Vanrumste, B., Papy, J.-M., Van Paesschen,
W., and Van Huffel, S. (2005). Modeling common
dynamics in multichannel signals with applications
to artifact and background removal in EEG record-
ings. IEEE Transactions on Biomedical Engineering,
52(12):2006–2015.
Duun-Henriksen, J., Kjaer, T. W., and et al (2012). Chan-
nel selection for automatic seizure detection. Clinical
Neurophysiology, 123:84–92.
Guerrero-Mosquera, C., Malanda-Trigueros, A., Iriarte-
Franco, J., and Navia-Vazquez, A. (2010). New fea-
ture extraction approach for epileptic EEG signal de-
tection using time-frequency distributions. Med. Biol.
Eng. Comput., 48:321–330.
Guerrero-Mosquera, C. and Navia-Vazquez, A. (2012). Au-
tomatic removal of ocular artifacts using Adaptive Fil-
tering and Independent Component Analysis for EEG
data. IET Signal Processing, 6:99–106.
Lodder, S. and Putten, M. V. (2011). Automated eeg anal-
ysis: Characterizing the posterior dominant rhythm.
Journal of Neuroscience Methods , 200:86–93.
Nam, H., Yim, T.-G., Han, S. K., Oh, J.-B., and Lee,
S. K. (2002). Independent Component Analysis of ic-
tal EEG in medial temporal lobe epilepsy. Epilepsia,
43:160–164.
Orekhova, E., Elam, M., and Orekhov, V. (2011). Adaptive
estimation of EEG-rhythms for optimal band identi-
fication in BCI. Journal of Neuroscience Methods,
195:47–60.
Peters, B., Pfurtscheller, G., and Flyvbjerg, H. (2001). Au-
tomatic differentiation of Multichannel EEG signals.
IEE Trans. On Bio Med Eng, 48:111–116.
BIOSIGNALS2013-InternationalConferenceonBio-inspiredSystemsandSignalProcessing
288