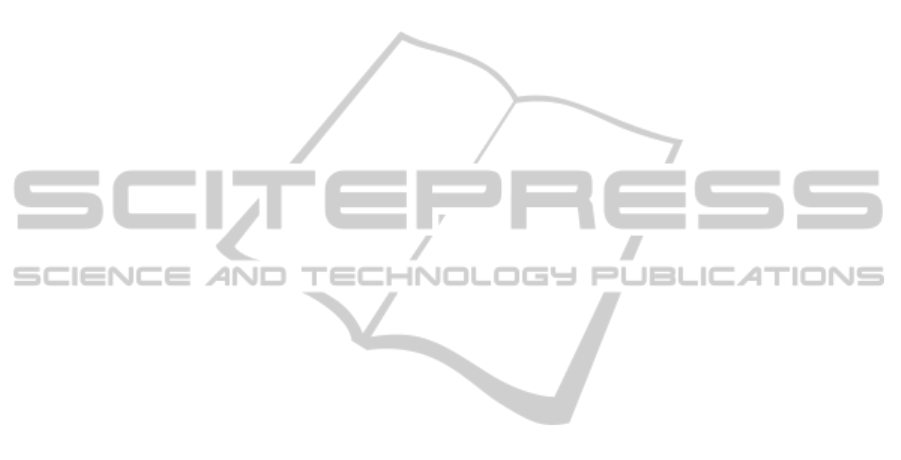
but both reasons have similar impacts on total egress
times. The type of consensus mechanism used, the
amount of knowledge shared, and the cost of sharing
knowledge are all shown to have a significant impact
on the overallegress times predicted by the simulation
and are therefore important factors to include when
designing a realistic pedestrian simulator.
This work is supported by NSF research grant
#0812039 entitled “Coalition Formation with Agent
Leadership.”
REFERENCES
Antonini, G., Bierlaire, M., and Weber, M. (2006). Dis-
crete choice models of pedestrian walking behav-
ior. Transportation Research Part B: Methodological,
40(8):667–687.
Bosse, T., Hoogendoorn, M., Klein, M. C. A., Treur, J.,
and Van Der Wal, C. N. (2011). Agent-based analysis
of patterns in crowd behaviour involving contagion of
mental states. In Proceedings of the 24th international
conference on Industrial engineering and other appli-
cations of applied intelligent systems conference on
Modern approaches in applied intelligence - Volume
Part II, pages 566–577, Berlin, Heidelberg. Springer-
Verlag.
Feuz, K. (2011). Pedestrian leadership and egress assistance
simulation environment. Master’s thesis, Utah State
University.
Feuz, K. and Allan, V. (2012a). Simulating knowledge
and information in pedestrain egress. In 4th Inter-
national Conference on Agents and Artificial Intelli-
gence, pages 246–253.
Feuz, K. and Allan, V. (2012b). Simulating pedestrain
route selection with imperfect knowledge. In 4th In-
ternational Conference on Agents and Artificial Intel-
ligence, pages 146–153.
Helbing, D. and Johansson, A. (2009). Pedestrian,
crowd and evacuation dynamics. In Encyclopedia of
Complexity and Systems Science, pages 6476–6495.
Springer.
Hoogendoorn, M., Treur, J., van der Wal, C., and van Wis-
sen, A. (2010). Modelling the interplay of emotions,
beliefs and intentions within collective decision mak-
ing based on insights from social neuroscience. In
Wong, K., Mendis, B., and Bouzerdoum, A., edi-
tors, Neural Information Processing. Theory and Al-
gorithms, volume 6443 of Lecture Notes in Computer
Science, pages 196–206. Springer Berlin / Heidelberg.
Hoogendoorn, S. and Bovy, P. (2004). Pedestrian route-
choice and activity scheduling theory and models.
Transportation Research Part B: Methodological,
38(2):169–190.
Kretz, T., Hengst, S., and Vortisch, P. (2008). Pedes-
trian flow at bottlenecks - validation and calibration
of vissim’s social force model of pedestrian traf-
fic and its empirical foundations. Technical Report
arXiv:0805.1788. Comments: Accepted for presenta-
tion at International Symposium of Transport Simula-
tion 2008 (ISTS08) in Gold Coast, Australia.
Kułakowski, K. and Gawro´nski, P. (2009). To cooperate or
to defect? altruism and reputation. Physica A: Statis-
tical Mechanics and its Applications, 388(17):3581–
3584.
Luber, M., Stork, J., Tipaldi, G., and Arras, K. (2010). Peo-
ple tracking with human motion predictions from so-
cial forces. In 2010 IEEE International Conference
on Robotics and Automation (ICRA), pages 464–469.
IEEE.
Moussa¨ıd, M., Perozo, N., Garnier, S., Helbing, D., and
Theraulaz, G. (2010). The walking behaviour of
pedestrian social groups and its impact on crowd dy-
namics. PLoS One, 5(4):e10047.
Murakami, Y., Minami, K., Kawasoe, T., and Ishida, T.
(2002). Multi-agent simulation for crisis management.
In 2002. Proceedings. IEEE Workshop on Knowledge
Media Networking, pages 135–139. IEEE.
Ozel, F. (2001). Time pressure and stress as a factor during
emergency egress. Safety Science, 38(2):95–107.
Pan, X. (2006). Computational Modeling of Human and
Social Behaviors for Emergency Egress Analysis. PhD
thesis, Stanford University, Stanford, California.
Qingge, J. and Can, G. (2007). Simulating crowd evacua-
tion with a leader-follower model. IJCSES, 1(4):250.
Qiu, F. (2010). A Framework for Group Modeling in Agent-
Based Pedestrian Crowd Simulations. PhD thesis,
Georgia State University, Atlanta, Georgia.
Qiu, F. and Hu, X. (2010). Modeling group structures in
pedestrian crowd simulation. Simulation Modelling
Practice and Theory, 18(2):190–205.
Russell, S. and Norvig, P. (2010). Artificial Intelligence: A
Modern Approach. Prentice Hall.
Santos, G. and Aguirre, B. E. (2004). A critical review of
emergency evacuation simulation models. In Build-
ing Occupant Movement during Fire Emergencies,
Gaithersburg, Maryland.
Shehory, O. and Kraus, S. (1998). Methods for task allo-
cation via agent coalition formation. Artificial Intelli-
gence, 101(1-2):165 – 200.
Tsai, J., Fridman, N., Bowring, E., Brown, M., Epstein, S.,
Kaminka, G., Marsella, S., Ogden, A., Rika, I., Sheel,
A., Taylor, M. E., Wang, X., Zilka, A., and Tambe,
M. (2011). Escapes: Evacuation simulation with chil-
dren, authorities, parents, emotions, and social com-
parison. In Proceedings of the International Confer-
ence on Autonomous Agents and Multiagent Systems
(AAMAS).
Yang, L., Zhao, D., Li, J., and Fang, T. (2005). Simulation
of the kin behavior in building occupant evacuation
based on cellular automaton. Building and Environ-
ment, 40(3):411–415.
Zhao, D., Yang, L., and Li, J. (2008). Occupants’ behavior
of going with the crowd based on cellular automata
occupant evacuation model. Physica A: Statistical
Mechanics and its Applications, 387(14):3708–3718.
ICAART2013-InternationalConferenceonAgentsandArtificialIntelligence
364