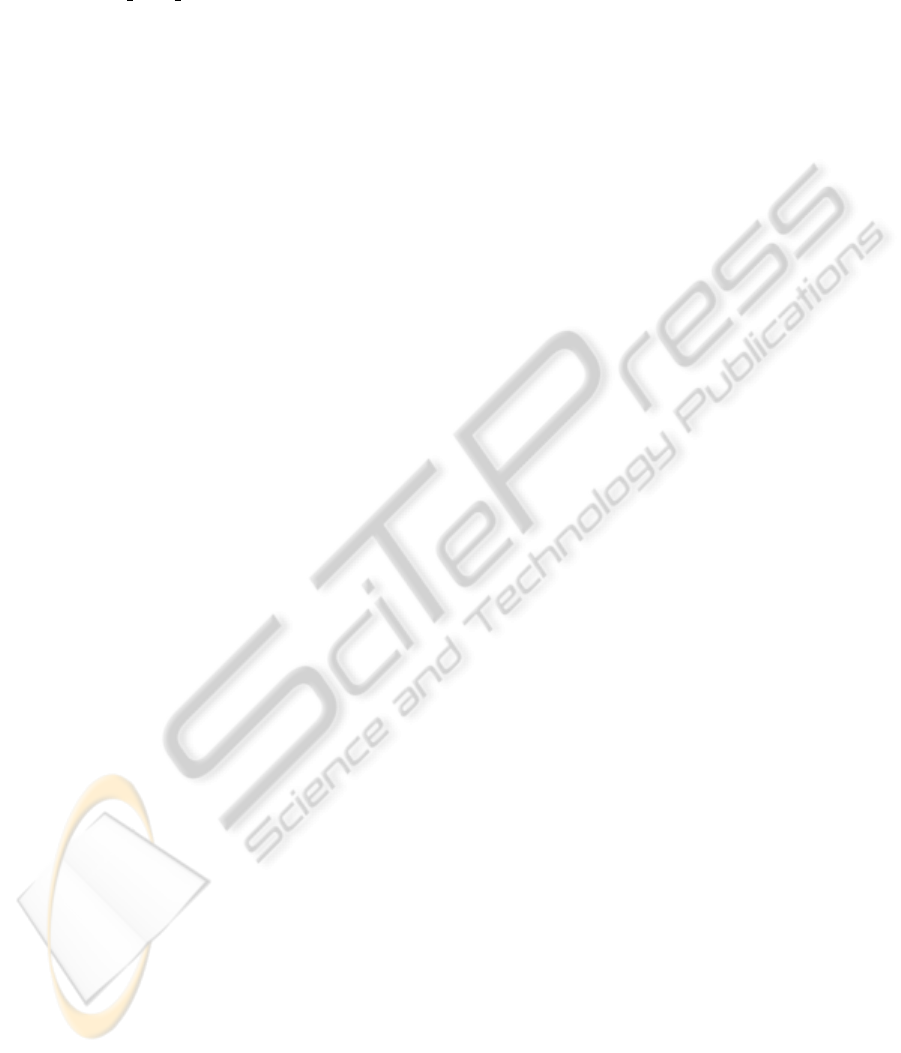
valid in this situation as well. It is worth noting the re-
markable discriminant and invariant properties of the
all the features extracted by KPCA from the source
image. The QDA KPCA 1DOM curve is the most sta-
ble across the entire range of features provided to the
model.
In conclusion, Fig. 1(f) uncovers the behavior of
some of the LDA models when asked, after HM and
after the projection, to predict the class labels back
on the source image. Although the pattern is not as
evident as expected, we can appreciate the loss in
accuracy induced by the FE based also on pixels is-
sued from another domain. This confirms that out-
domain data interfere with the proper extraction of
discriminant domain-specific features, while improv-
ing the overall generalization abilities of the system
when dealing with cross-domain knowledge transfer.
5 CONCLUSIONS
In this paper, the analysis of feature extraction tech-
niques to jointly transform two related remote sens-
ing images to align their feature spaces has been pre-
sented. After the projection, the matched images
display an increased discrimination between ground
cover classes, allowing a supervised classifier to ob-
tain an accurate generalization on both source and tar-
get domains.
Experiments proved that the combination of the
histogram matching procedure with the feature ex-
traction step is extremely beneficial, confirming the
mandatory application of the former before any do-
main adaptation task. Among the extraction tech-
niques, we noticed the slight superiority of kernel-
based features extractors (KPCA and TCA) with re-
spect to simple linear techniques such as PCA. No no-
table differences have been observed between the two
kernel methods. This fact suggests that, rather than
the reduction of the divergence between marginal dis-
tributions governing the two images, as pursued by
TCA, the key benefit is the increased class separabil-
ity. Also, we found that the use of pixels from one im-
age only to compute the projection provides equally
invariant features as a joint sampling of the images.
These results open a number of opportunities to
practitioners of the field dealing with large scale
land cover mapping applications involving several re-
motely sensed images.
As an outlook on new research directions, we plan
to test supervised FE methods. Techniques such as
Kernel Fisher Discriminant Analysis, Kernel Canon-
ical Correlation Analysis, Kernel Orthogonal Partial
Least Squares, etc. could be used to find the proper
projections based on the labeled source domain data.
ACKNOWLEDGEMENTS
This work has been supported by the Swiss National
Science Foundation with grants no. 200021-126505
and PZ00P2-136827.
REFERENCES
Arenas-Garc
´
ıa, J. and Petersen, K. B. (2009). Kernel mul-
tivariate analysis in remote sensing feature extraction.
In Camps-Valls, G. and Bruzzone, L., editors, Kernel
Methods for Remote Sensing Data Analysis. J. Wiley
& Sons, NJ, USA.
Borgwardt, K. M., Gretton, A., Rasch, M. J., Kriegel, H.-P.,
Sch
¨
olkopf, B., and Smola, A. J. (2006). Integrating
structured biological data by Kernel Maximum Mean
Discrepancy. Bioinformatics, 22(14):e49–e57.
Bruzzone, L. and Persello, C. (2009). A novel approach
to the selection of spatially invariant features for the
classification of hyperspectral images with improved
generalization capability. IEEE Trans. Geosci. Remote
Sens., 47(9):3180–3191.
Bruzzone, L. and Prieto, D. F. (2001). Unsupervised retrain-
ing of a maximum likelihood classifier for the analysis
of multitemporal remote sensing images. IEEE Trans.
Geosci. Remote Sens., 39(2):456–460.
Inamdar, S., Bovolo, F., and Bruzzone, L. (2008). Multidi-
mensional probability density function matching for
preprocessing of multitemporal remote sensing im-
ages. IEEE Trans. Geosci. Remote Sens., 46(4):1243–
1252.
Nielsen, A. A., Conradsen, K., and Simpson, J. J. (1998).
Multivariate alteration detection (MAD) and MAF
postprocessing in multispectral, bitemporal image
data: New approaches to change detection studies. Re-
mote Sens. Environ., 64:1–19.
Pan, S. J., Tsang, I., Kwok, J. T., and Yang, Q. (2011).
Domain adaptation via transfer component analysis.
IEEE Trans. Neural Netw., 22(2):199–210.
Pan, S. J. and Yang, Q. (2010). A survey on transfer learn-
ing. IEEE Trans. Knowl. Data Eng., 22(10):1345–
1359.
Sch
¨
olkopf, B., Smola, A., and M
¨
uller, K.-R. (1998). Non-
linear component analysis as a kernel eigenvalue prob-
lem. Neural Comput., 10(5):1299–1319.
Tuia, D., Mu
˜
noz-Mar
´
ı, J., Gomez-Chova, L., and Malo, J.
(2012). Graph matching for adaptation in remote sens-
ing. IEEE Trans. Geosci. Remote Sens., PP(99):1–13.
Woodcock, C. E., Macomber, S. A., Pax-Lenney, M., and
Cohen, W. B. (2001). Monitoring large areas for forest
change using Landsat: Generalization across space,
time and Landsat sensors. Remote Sens. Environ.,
78(1-2):194–203.
ICPRAM2013-InternationalConferenceonPatternRecognitionApplicationsandMethods
424