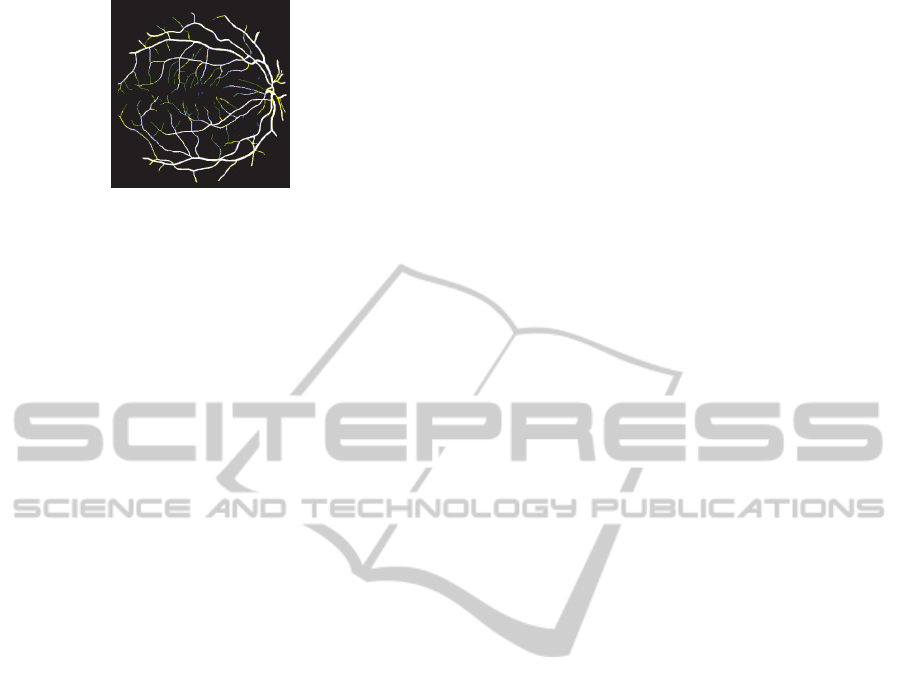
Figure 7: Hand labeled image superimposition with the
hand labeled image after the morphological opening and
with MAS result.
4 CONCLUSIONS
In this study, a MAS approach is proposed where
agents enrich a traditional edge detector algorithm.
The experiments show that the use of a MAS model
in the micro level could be an effective way to
segment structures in complex images such as retinal
images. In fact, through environment perception and
local interactions, a simple agent organization can
have as global behavior the most part of retinal
vasculature detection. The use of an improved
version of agent society with some knowledge a
priori about the retina proprieties, complemented
with some other traditional image processing
algorithms, could have the potential to develop a
system to detect and differentiate all the anatomic
and pathological structures of the fundus images.
Such an approach will overcome the classic image
processing algorithms that are limited to macro
results which cannot take into account the local
characteristics of a complex image. Therefore, it
could be a fundamental tool responsible for a very
efficient system development to be used in screening
programs concerning DR diagnosis.
ACKNOWLEDGEMENTS
Work supported by FEDER funds through the
"Programa Operacional Factores de Competitividade
– COMPETE" and by national funds by FCT-
Fundação para a Ciência e a Tecnologia. C. Pereira
thanks the FCT for the SFRH/BD/61829/2009 grant.
REFERENCES
Bovenkamp, E.G.P., Dijkstra, J., Bosch, J.G., and Reiber,
J. H. C. (2004). Multi-agent segmentation of IVUS
images. Pattern Recognition, 37:647-663.
Gutknecht, O., Ferber, J., (2000). Madkit: a generic multi-
agent platform. In: Proceedings of the 4th
international conference on Autonomous agents,
Barcelona, Spain, 78-79.
Kuwahara, M. , Hachimura, K., Eiho, S., and Kinoshita,
M. (1976) Digital Processing of Biomedical Images
(pp. 187-203). Plenum Press: New York.
Lalkhen, A. G., McCluskey, A. (2008). Statistics VI:
Clinical tests: sensitivity and specificity. Contin Educ
Anaesth Crit Care Pain, 8(6):221-223.
Mahdjoub, J., Guessoum, Z., Michel, F., and Herbin, M.
(2006). A multi-agent approach for the edge detection
in image processings. in 4th European Workshop on
Multi-Agent Systems, Lisbon, Portugal.
Mendonça, A., and Campilho, A. (2006). Segmentation of
retinal blood vessel by combining the detection of
centerlines and morphological reconstruction. IEEE
Trans. Med. Imag., 25(9):1200-1213.
Niemeijer, M., Ginneken, B. van, Staal, J., Suttorp-
Schulten, M., and Abràmoff, M.D.(2005). Automatic
detection of red lesions in digital color fundus
photographs. IEEE Trans. Med. Imag., 24(5): 584-
592.
Papari, G., Petkov, N., Campisi, P., (2007). Artistic edge
and corner enhancing smoothing. IEEE Transactions
on Medical Imaging 16 (10):2449 - 2462.
Richard, N., Dojat, M., and Garbay, C. (2004). Distributed
Markovian segmentation: Application to MR brain
scans. Pattern Recognition, 40:3467 – 3480.
Staal, J. J., Abrámoff, M. D., Niemeijer, M., Viergever, M.
A., and Ginneken, B. van (2004). Ridge-based vessel
segmentation in color images of the retina. IEEE
Trans. Med. Imag., 23(4): 501-509.
Zhang, B., Zhang, L., Zhang, L., and Karray, F. (2010).
Retinal vessel extraction by matched filter with first-
order derivative of Gaussian. Computers in Biology
and Medicine, 40:438-445.
RetinalBloodVesselSegmentationbyaMASApproach
293