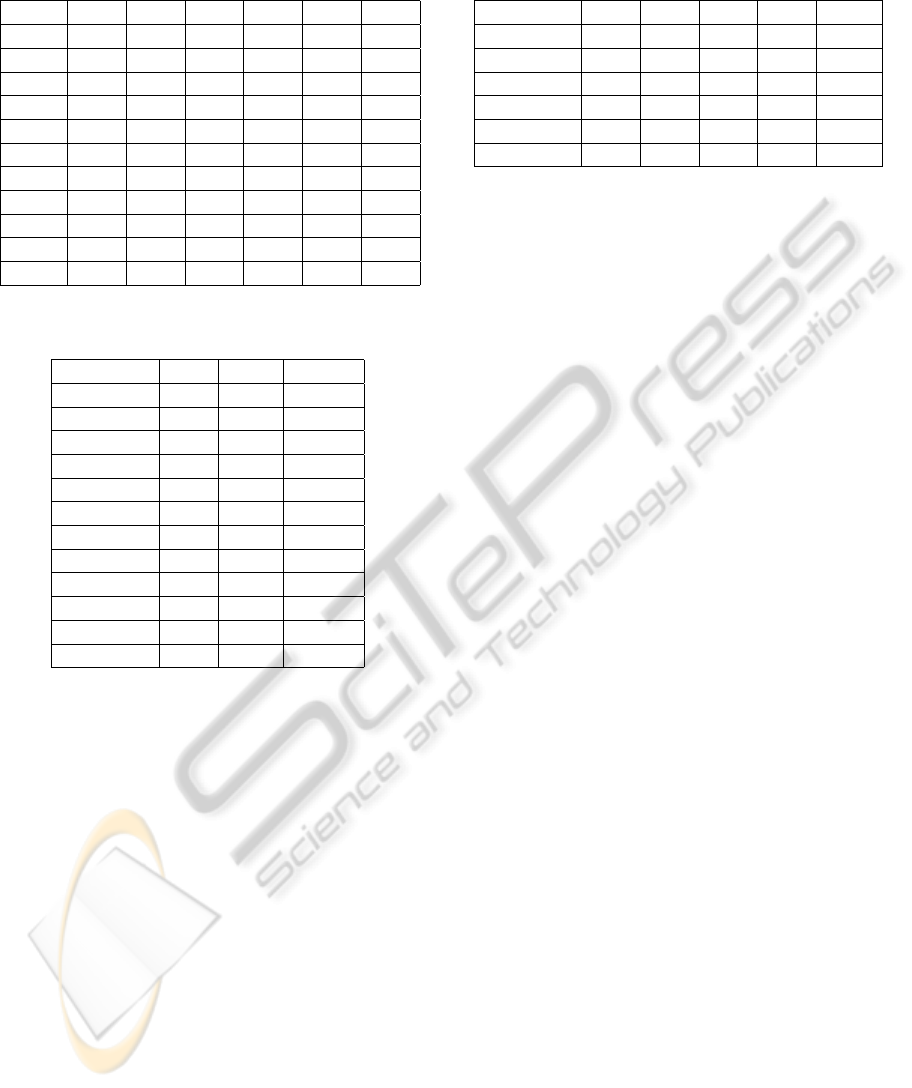
Table 1: DCG (Percent) Performance of DBS with different
coefficients on PSB test.
a2/a3 0.0 0.1 0.2 0.3 0.4 0.5
0.0 61.2 62.7 64.0 65.1 65.5 65.7
0.1 62.5 63.9 65.2 66.1 66.6 66.6
0.2 63.6 64.9 66.1 66.9 67.3 67.1
0.3 64.4 65.7 66.7 67.5 67.6 67.4
0.4 65.1 66.2 67.1 67.7 67.6 67.1
0.5 65.5 66.5 67.2 67.4 67.3 66.4
0.6 65.6 66.6 67.0 67.1 66.4
0.7 65.6 66.2 66.6 66.3
0.8 65.3 65.7 65.9
0.9 64.8 64.9
1.0 63.9
Table 2: Retrieval statistics (Percent) of state-of-art 3D
shape descriptors on PSB test.
Descriptor NN DCG NDCG
DBS 70.5 67.8 8.7
CRSP 67.9 66.8 7.1
DSR 66.5 66.5 6.6
DBF 68.6 65.9 5.7
SWD 46.9 65.4 4.9
LFD 65.7 64.3 3.1
DBI 60.9 61.4 -1.6
REXT 60.2 60.1 -3.7
SIL 55.7 59.7 -4.3
RISH 55.6 58.4 -6.4
3DHT 58.8 57.7 -7.5
SHIST 54.6 54.5 -12.6
model retrieval algorithms on PSB. The other statis-
tics given in Table 2 are taken from the study in
(Akgul et al., 2009).
From Table 2 we see that though PSB is one of
the most challenging databases, DBS is among the top
cluster. The reason is that low resolution and degen-
eracies can be avoided in computing DBS and these
are main characters of models in PSB.
The other statistics in Table 3 are taken from the
study in (Vanamali et al., 2010). From Table 4 we see
that DBS can also perform well on a large database.
Compared to SIL, DBS has a better performance.
4 CONCLUSIONS
In this work, we have introduced a new 3D shape
descriptor, Density-Based Silhouette descriptor. The
new descriptor inherits advantages from both DBF
and SIL and shows high retrieval accuracy on several
3D databases with varying mesh resolution, semantic
content and classification granularity. On PSB, one of
Table 3: Retrieval statistics (Percent) of state-of-art 3D
shape descriptors on GWSB2010.
Descriptor NN FT ST E DCG
DSR472 87.1 49.8 63.9 35.6 83.1
LFD 86.4 48.0 61.3 33.6 81.6
DBS 80.9 41.5 56.0 30.1 78.2
SIL300 80.7 41.2 54.8 30.0 78.0
DSR438 80.9 40.7 53.2 30.6 77.0
RSH136 78.3 38.5 50.8 27.5 75.8
the most challenging database, its DCG is nearly two
percent higher than DBF’s. On GWSB2010 which
has more than 3000 models, DBS also has a high re-
trieval accuracy. The retrieval experiments on two
databases show that DBS can be adapted to a broad
and heterogeneous set of shape categories.
REFERENCES
Akgul, C., Sankur, B., Yemez, Y., and Schmitt, F. (2009). 3d
model retrieval using probability density-based shape
descriptors. IEEE Trans on Pattern Analysis and Ma-
chine Intelligence, 31(6):1117–1133.
Bronstein, A., Bronstein, M., and Kimmel, R. (2006). Effi-
cient computation of isometry-invariant distances be-
tween surfaces. In SIAM J. Scientific Computing, vol-
ume 28, pages 1812–1836.
Li, B., Godil, A., Aono, M., and Bai, X. (2012). Shrec’12
track: Generic 3d shape retrieval. Eurographics Work-
shop on 3D Object Retrieval.
Marini, S., Paraboschi, L., and Biasotti, S. (2007). Shape
retrieval contest 2007: Partial matching track. In Proc.
SHREC in Conjunction with Proc. Intl Conf. Shape
Modeling and Applications, pages 13–16.
Min, P., Kazhdan, M., and Funkhouser, T. (2004). A com-
parison of text and shape matching for retrieval of on-
line 3d models. In Proc. European conference on dig-
ital libraries, pages 209–220.
Shilane, P., Min, P., Kazhdan, M., and Funkhouser, T.
(2004). The princeton shape benchmark. In Proc.
Shape Modeling Int’l, pages 167–178.
Tangelde, J. and Veltkamp, R. (2008). A survey of content
based 3d shape retrieval methods. Multimedia Tools
and Applications.
Vanamali, T., Godil, A., Dutagaci, H., Furuya, T., Lian, Z.,
and Ohbuchi, R. (2010). Shrec’10 track: Generic 3d
warehouse. Eurographics Workshop on 3D Object Re-
trieval.
Vranic, D. (2004). 3D Model Retrieval. PhD dissertation,
Univ. of Leipzig.
Yang, C., Duraiswami, R., Gumerov, N., and Davis, L.
(2003). Improved fast gauss transform and efficient
kernel density estimation. In Proc. Int’l Conf. Com-
puter Vision, volume 1, page 464.
ICPRAM2013-InternationalConferenceonPatternRecognitionApplicationsandMethods
270