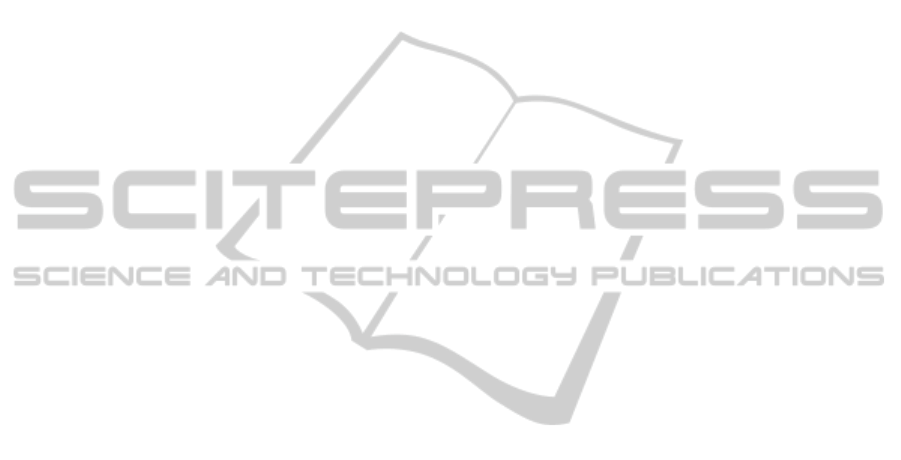
IJKDIJK section should be treated in the same way
as these dike stability models.
2. How to avoid unnecessary running of the CPU
intensive dike stability models? Dike stability
models can be complex and even in non-critical
situations demand a lot of CPU power. Running
these models continuously for thousands of
kilometres dikes can therefore be quite costly.
It is suggested by Langius (2011) to use simple
anomaly detection techniques as a trigger for
running the dike stability models. This results in a
reduction of using the CPU power most of the time.
Only during potentially critical situations additional
CPU power can be required in the cloud to run the
dike stability models to get insight into the changes
of particular dike failure mechanisms.
5 CONCLUSIONS
Based on the work presented in this paper we can
state that monitoring dikes using sensor systems in
combination with information and communication
technology is possible, on a small scale. Based on
the lessons learnt we advise:
• to adapt or develop dike stability models to deal
with new types of sensors used in the dikes;
• to use anomaly detection techniques when there
are no dike stability models available;
• to use noSQL databases to realize a robust
(highly available & partitioning tolerant) sensor data
storage;
• to work on standardization and semantics for a
more mature market where integration of different
components is less costly in terms of time and
money;
• to apply innovative aggregation techniques to
enable viewing data from a large timeframe.
Scaling towards mid and large scale dike monitoring
requires solutions to be found for bringing power
and Internet (i.e. communication) to the (rural) dike
locations.
For large-scale dike monitoring two major IT-
challenges have been identified. To cope with these
challenges we advise to work in the following two
directions.
• To use cloud technology to deal with the
dynamic CPU power needs due to sample rate
changes and/or simulations.
• In order to reduce the costs of CPU power in
non-critical situations, also use anomaly detection
techniques to avoid continuous usage of
computationally intensive dike stability models.
Finally we want to state that to address these IT
lessons learnt, we are developing and combining
suitable technologies. Our goal is to make them as
generic as possible in order to be useable also in
other domains. At the moment we are already
involved in projects concerning the monitoring of
cracks in steel bridges, ground movement of gas
pipes and dairy farming.
ACKNOWLEDGEMENTS
The authors would like to acknowledge the IJkdijk
Foundation for making the fieldlab IJkdijk possible
and the water board Noorderzijlvest for the Livedike
Eemshaven location. And finally the national Flood
Control 2015 project and FP7 UrbanFlood project
for the research opportunities.
REFERENCES
Bezuijen, A., Kruse, G. A. M.,Van, M. A., 2005. Failure
of peat Dikes in the Netherlands, Proc. 16th Int. Conf.
On Soil Mechanics and Geotechnical Engineering,
Osaka, Rotterdam, Millpress, 2005, Vol.3, pp. 1857-
1860.
Brewer, E. A., 2000. Towards robust distributed systems,
(Invited talk) Principles of distributed computering,
Portland July 2000.
Gilbert, S., Lynch, N. A., 2002. Brewer's Conjecture and
the Feasibility of Consistent, Available: Partition-
Tolerant Web Services, News, June, 2002.
Koelewijn, A., Pals, N., Sas, M. J., Zomer, W. S., 2010.
IJkdijk Pipingexperiment - Validatie van sensor- en
meettechnologie voor detective van optreden van
piping in waterkeringen, ISBN 978.90.5773.485.4,
2010-26 PIW.
Kolk, B. J. van der, 2011. Livedijk Eemshaven - analyse
metingen, 1200888-001-GEO-0006.
Kruiver, P., Hopman, V., Research team, 2010. Models
and analysis for flood control systems, Flood Control
2015, 2010.02.02.3.
Krzhizhanovskaya, V. V. et al, 2011. Flood early warning
system: design, implementation and computational
modules, Procedia Computer Science 4 (2011) 106–
115, International Conference on Computational
Science, ICCS 2011.
Lang, B., Poppe, T., Minin, A., Mokhov, I., Kuperin, Y.,
Mekler, A., Liapakina, I., 2008. Neural Clouds for
Monitoring of Complex Systems. Optical Memory and
Neural Networks (Information Optics). 2008. V. 17. N
3, pp. 183–192.
Langius, E. A. F., et al, 2009. Robust monitoring – Added
value of sensor streams in dike monitoring systems,
2009.02.01.1.
SENSORNETS2013-2ndInternationalConferenceonSensorNetworks
78