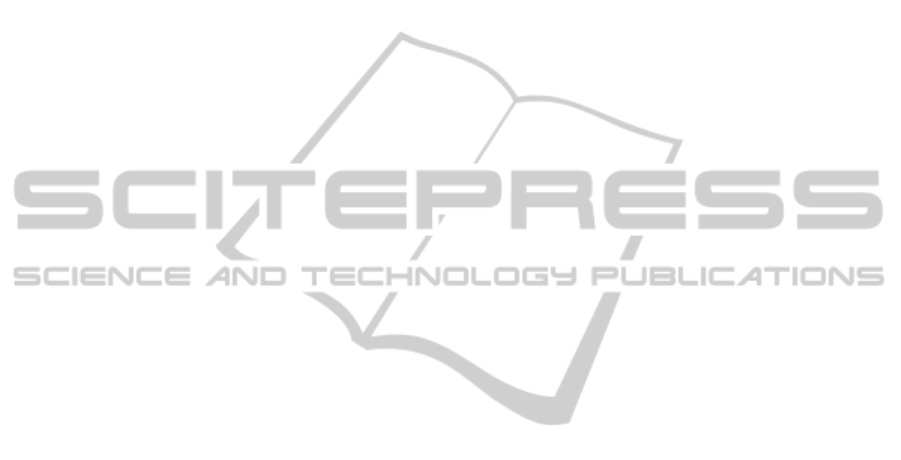
datasets, for which skeleton stream is provided. The
main result is that the descriptor based on bag-of-
gestures outperforms three very recent methods of the
state-of-the-art on the two databases : 88.9% of F
0.5
measure on CUHA database and 84.5% of correct
classification on TUM dataset.
We also study the proposed algorithm on a more
difficult database not designed to extract skeleton: the
public RGBD-HuDaAct database. During the skele-
ton estimation performed with NITE software, some
problems occurred with side or back views of peo-
ple, or when the person has very specific postures that
do not allow skeleton extraction. Even if the RGBD-
HuDaAct database is really challenging to perform
action recognition based on skeletons, it has been con-
sidered here to represent some conditions of video
surveillance system at home. The main result of these
tests if that evenin these conditions, and with a signif-
icant amount of missing data, our descriptor achieves
the state-of-the-art performance of 82% of recogni-
tion rate. To our knowledge, it is the first time that
failures in skeleton extraction are considered during
action recognition.
In future works, we will continue to explore the
links between high-level human actions and elemen-
tary gestures and design a framework to learn middle-
semantic gestures which are the most relevant for hu-
man action recognition. Such framework will allow
us to recognize an action from a small number of
middle-semantic gestures whereas the algorithm pre-
sented in this work recognizes actions from the set of
all gestures. Hence, such framework is likely to both
speed up and increase accuracy of the system. In addi-
tion, we will extend current bag-of-gestures descrip-
tor by taking into account the co-occurrence relation
of different articulations and co-occurrencerelation of
pairs of successive gestures of a given articulation.
REFERENCES
Aggarwal, J. K. and Ryoo, M. S. (2011). Human activity
analysis: A review. ACM Comput. Surv.
Baak, A., Muller, M., Bharaj, G., Seidel, H., and Theobalt,
C. (2011). A data-driven approach for real-time full
body pose reconstruction from a depth camera. In
Computer Vision (ICCV), 2011 IEEE International
Conference on, pages 1092–1099. IEEE.
Ballas, N., Delezoide, B., and Prˆeteux, F. (2011). Tra-
jectories based descriptor for dynamic events annota-
tion. In Proceedings of the 2011 joint ACM workshop
on Modeling and representing events, pages 13–18.
ACM.
Barnachon, M., Bouakaz, S., Guillou, E., and Boufama, B.
(2012). Interpr´etation de mouvements temps r´eel. In
RFIA.
Bashir, F., Khokhar, A., and Schonfeld, D. (2007). Ob-
ject trajectory-based activity classification and recog-
nition using hidden markov models. Image Process-
ing, IEEE Transactions on, 16(7):1912–1919.
Breiman, L. (1992). Probability. Society for Industrial and
Applied Mathematics, Philadelphia, PA, USA.
Campbell, L. and Bobick, A. (1995). Recognition of human
body motion using phase space constraints. In Com-
puter Vision, 1995. Proceedings., Fifth International
Conference on, pages 624–630. IEEE.
Chang, C. and Lin, C. (2011). Libsvm: a library for sup-
port vector machines. ACM Transactions on Intelli-
gent Systems and Technology (TIST), 2(3):27.
Fengjun, L., Nevatia, R., and Lee, M. W. (2005). 3d hu-
man action recognition using spatio-temporal motion
templates. ICCV’05.
Girshick, R., Shotton, J., Kohli, P., Criminisi, A., and
Fitzgibbon, A. (2011). Efficient regression of general-
activity human poses from depth images. In Computer
Vision (ICCV), 2011 IEEE International Conference
on, pages 415–422. IEEE.
He, H. and Ghodsi, A. (2010). Rare class classification
by support vector machine. In Pattern Recognition
(ICPR), 2010 20th International Conference on, pages
548–551. IEEE.
Heikkil¨a, M., Pietik¨ainen, M., and Schmid, C. (2009). De-
scription of interest regions with local binary patterns.
Pattern recognition, 42(3):425–436.
Hsu, C. and Lin, C. (2002). A comparison of methods for
multiclass support vector machines. Neural Networks,
IEEE Transactions on, 13(2):415–425.
Johansson, G. (1973). Visual perception of biological mo-
tion and a model for its analysis. Attention, Percep-
tion, & Psychophysics, 14(2):201–211.
Just, A., Marcel, S., and Bernier, O. (2004). Hmm and
iohmm for the recognition of mono-and bi-manual 3d
hand gestures. In ICPR workshop on Visual Observa-
tion of Deictic Gestures (POINTING04).
Laptev, I., Marszalek, M., Schmid, C., and Rozenfeld,
B. (2008). Learning realistic human actions from
movies. In Computer Vision and Pattern Recognition,
2008. CVPR 2008. IEEE Conference on, pages 1–8.
IEEE.
Lazebnik, S., Schmid, C., and Ponce, J. (2005). A sparse
texture representation using local affine regions. Pat-
tern Analysis and Machine Intelligence, IEEE Trans-
actions on, 27(8):1265–1278.
Li, W., Zhang, Z., and Liu, Z. (2010). Action recognition
based on a bag of 3d points. In Computer Vision and
Pattern Recognition Workshops (CVPRW),2010 IEEE
Computer Society Conference on, pages 9–14. IEEE.
Liu, J., Luo, J., and Shah, M. (2009). Recognizing real-
istic actions from videos ’in the wild’. In Computer
Vision and Pattern Recognition, 2009. CVPR 2009.
IEEE Conference on, pages 1996–2003. IEEE.
Lowe, D. (1999). Object recognition from local scale-
invariant features. In Computer Vision, 1999. The Pro-
ceedings of the Seventh IEEE International Confer-
ence on, volume 2, pages 1150–1157. Ieee.
VISAPP2013-InternationalConferenceonComputerVisionTheoryandApplications
528