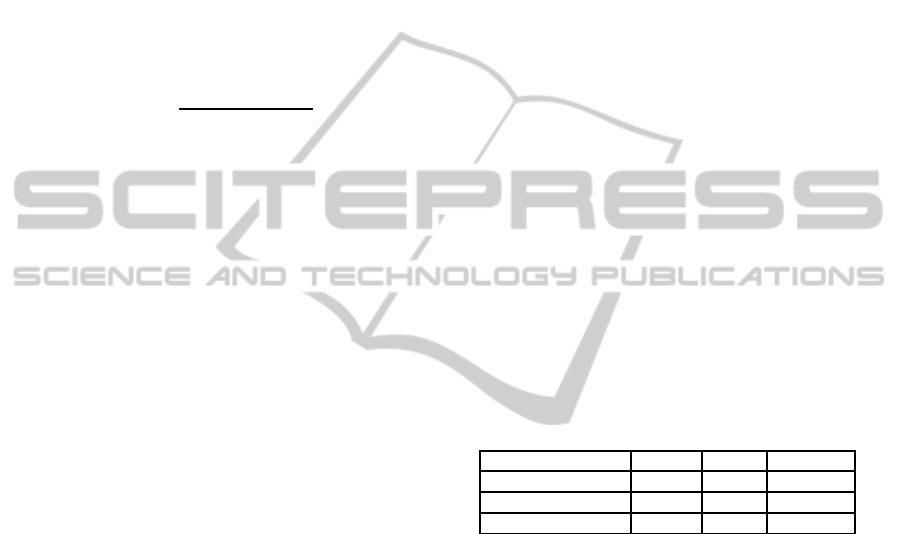
where α is a weight parameter that controls the impact
of AC-Patterns and DC-Patterns histogram.
3.2 Similarity Measurement
The similarity between query and images in the
database is assessed by the distance between feature
descriptors. A similarity measure assigns a lower dis-
tance or high score to more similar objects.
In our approach, χ
2
distance is used to measure
the similarity. Assuming D
i
and D
j
are the query and
target feature descriptors respectively, the χ
2
distance
is defined as follows:
Dis
i,j
=
m
∑
k=1
(D
i
(k) − D
j
(k))
2
D
i
(k) + D
j
(k)
(2)
where m indicates the dimension of descriptors.
4 EXPERIMENTAL RESULTS
To evaluate the proposal, we perform experiments on
ORL face database (AT&T Laboratories Cambridge,
1992), GTF face database (Georgia Tech, 1999),
FERET face database (Phillips et al., 2000) and Vis-
Tex texture database (Media Laboratory, 1995).
4.1 Experiments on Face Databases
The ORL database includes 10 different images of
40 persons. The GTF database includes 15 differ-
ent face images of 50 different persons. As we want
to compare our proposal with the methods presented
in (Zhong and Def´ee, 2005) and (Bai et al., 2012), we
implemented similar experiments as them, that means
use first 6 images as image database and remaining
4 images as query images for recognition on ORL
database and first 11 images of each person as image
database and remaining 4 images as query images on
GTF database.
As ORL and GTF are relatively small databases,
to further illustrate the contribution of the proposal,
we also implement it on FERET database which con-
tains more than 10000 images from more than 1000
persons. The two sets of frontal view faces fa and fb
were selected to evaluate the proposed method: fb is
used as query images for retrieval from the fa.
For evaluating the performance, we use Equal Er-
ror Rate (EER) (Bolle et al., 2000). Images are con-
sidered as similar if the distance between their fea-
tures descriptors is under a given threshold. So con-
sidering a query image belonging to class A, two
things could occur: on one hand, it could be recog-
nized rightly; on the other hand, it could be falsely
rejected from class A, then the ratio of how many im-
ages of class A are in this situation is called False Re-
jected Rate (FRR). In contrast, considering a query
image out of class A, when it is compared with the
images of class A, it could be rejected rightly or it
could be falsely accepted as class A, then the ratio
of how many images of other classes are in this situa-
tion is defined as False Accept Rate (FAR). These two
rates will change when the threshold changes. When
FRR and FAR take equal values, an equal error rate
(EER) is got. The lower the EER is, the better is the
performance.
As mentioned before, the concatenation of the
AC-Pattern histogram and the DC-Pattern histogram
is used to do image retrieval. To verify the effec-
tiveness of the proposed new method on constructing
AC-Pattern, the DC-Patterns histogram is the same
through all the experiments, but the construction of
AC-Pattern are different. We name the method pre-
sented in (Zhong and Def´ee, 2005) as “Zhong”, the
method presented in (Bai et al., 2012) as “Bai”. For
these two methods and our proposal, we tested differ-
ent sets of parameters to find the one that can assure
the best performance, and only the best performances
are compared that are shown in Table 1. From this
table, we can see that the proposal outperforms the
referred methods.
Table 1: Comparison of EER (%) on face databases.
Database/Method Zhong Bai Proposal
ORL 6.07 3.75 3.25
GTF 11.57 11.19 9.84
FERET 3.75 3.57 3.35
4.2 Experiments on Texture Database
In order to evaluate extensibility of the proposal in a
wider application field, we work on a selection of im-
ages from the Vistex Texture Database (Media Lab-
oratory, 1995), consisting of 40 textures which have
already been extensively used in texture image re-
trieval literature (Do and Vetterli, 2002) (Kokare M.
and Chatterji, 2005) (Kwitt and Uhl, 2010).
The 512 × 512 pixels color version of the tex-
tures are divided into 16 non-overlapping subimages
(128×128 pixels) and convertedto gray scale images,
thus creating a database of 640 images belonging to
40 texture-classes, each class includes 16 different
samples. In this retrieval experiments, each image is
used as query image. The relevant images for each
query consists of all the subimages from the same
original texture. Like in other literatures (Do and Vet-
terli, 2002) (Kokare M. and Chatterji, 2005) (Kwitt
and Uhl, 2010), we use the average retrieval rate
VISAPP2013-InternationalConferenceonComputerVisionTheoryandApplications
744