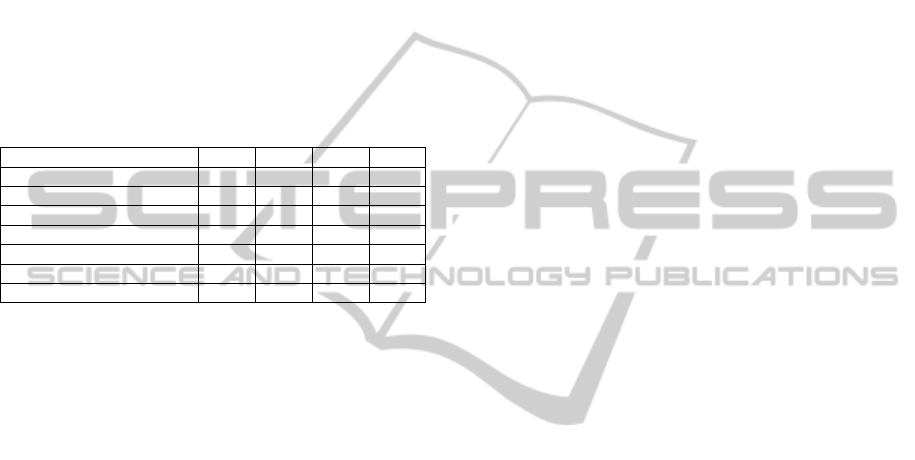
This time is lower than 0.5 second (Sharabaty et al.,
2008) for a normal blink and it is greater than two
seconds (Dinges et al., 1998) for a blink of an
individual in a state of drowsiness. In brief, the three
conducted experiments show the difference between
an alert individual and a sleepy one by analyzing the
variation of the features D
andD
. Generally, the
detection of states of drowsiness is processed by the
location of blinking first, then by studying the speed
of blinking and finally by calculating the duration of
eye closure. The driver is considered in a state of
drowsiness if the speed of eye closure exceeds 1
second or the duration of eye closure is greater than
2 second.
Table 1: Result of drowsiness detection.
Videos 1 2 3 4
Real drowsiness 9 7 4 8
Generated alarm 8 7 4 8
False negative 1 0 0 0
False positive 0 0 0 0
Correct alarm 8 7 4 7
Correct rate of fatigue 88% 100% 100% 87%
Accuracy rate of fatigue 100% 100% 100% 100%
Table 1 shows an example of drowsiness
detection result conducted on the four test videos.
The opinion of an expert in this step is essential to
determine the actual driver drowsiness. Our
approach does not generate false alarms for the
detection of fatigue in all videos. On the other hand,
there are alarm errors of states of fatigue due to false
detections of the iris that influences the detection of
both eyelids and subsequently the values of features.
3 CONCLUSIONS
This paper presents an approach to the detection of
reduced alertness, based on video analysis. Our
system uses a study of the eyes by analyzing the
video of several topics. The steps of detecting
drowsiness consist firstly of locating a driver face
and eyes by applying Haar features. The circular
Hough transform allows the detection of the center
of the iris and the intersection points of both eyelids
(Figure 2) in order to capture two geometric
features. The blink detection, the frequency and the
period of eye closure are major factors in
determining the fatigue of an individual. The
guidance, other facial feature as yawning, the
monitoring and the 3D pose estimation of the face
are also indicators of the state of vigilance of an
individual. These data are the subject of our future
work in order to improve the obtained results.
REFERENCES
Cauchie, J. Fiolet, V. Villers, D., (2008). Optimization of
an Hough transform algorithm for the search of a
center. Pattern Recognition. USA.
Dinges, D. Mallis, M. Maislin, G. Powell, J., (1998)
Evaluation of techniques for ocular measurement as
an index of fatigue and the basis for alertness
management. Departement of Transportation Highway
Safety. USA.
Hongbiao, M. Zehong, Y. Yixu, S. Peifa, J., (2008). A
Fast Method for Monitoring Driver Fatigue Using
Monocular Camera, Proceedings of the 11th Joint
Conference on Information Sciences. China.
Horng,W. Chen, C. Chang, Y., (2004). Driver fatigue
detection based on eye tracking and dynamic template
matching. IEEE International Conference on
Networking, Sensing & Control. USA.
Masayuki, K. Hideo, O. Tsutomu, N., (1999). Adaptability
to ambient light changes for drowsy driving detection
using image processing. UC Berkeley Transportation
Library
Murray, J. Andrew, T. Robert, C., (2005). A new method
for monitoring the drowsiness of drivers. International
Conference on Fatigue Management In
Transportation Operations. USA.
Picot, A. Caplier, A. Charbonnier, S., (2009). Comparison
between EOG and high frame rate camera for
drowsiness detection. IEEE Workshop on Applications
of Computer Vision. USA.
Rajinda, S. Budi, J. Sara, L. Arthur, H. Saman, H. Peter,
F., (2011). Comparing two video-based techniques for
driver fatigue detection: classification versus optical
flow approach, Machine Vision and Applications.
USA.
Tchernonog, G. Pelle, B. Larbi, M., (2008) Expertise
CHSCT Unité de production de Brétigny conduite.
France.
Viola, P.Jones, M. (2001). Rapid object detection using a
boosted cascade of simple features. CVPR. Kauai.
USA.
Wenhui, D. Xiuojuan, W., (2005). Driver Fatigue
Detection Based On The Distance Of Eyelid. IEEE
Workshop Vlsi Design & Video Tech. China.
Yong, D. Peijun, M. Xiaohong, S. Yingjun, Z., (2008).
Driver Fatigue Detection based on Eye State Analysis.
Proceedings of the 11th Joint Conference on
Information Sciences. China.
VISAPP2013-InternationalConferenceonComputerVisionTheoryandApplications
416