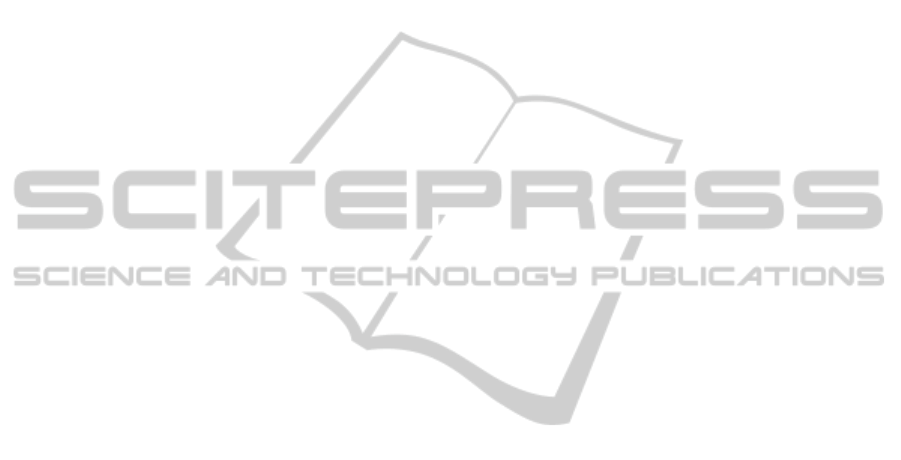
timation. In IEEF Proceedings Radar and Signal Pro-
cessing. IET,Vol. 1, pp. 107-113.
Isard, M. and Blake, A. (1998). Condensation conditional
density propagation for visual tracking. In Interna-
tional Journal of Computer Vision. Kluwer Academic
Publishers Hingham,MA,USA, Vol. 29, No. 1, pp. 5-
28.
Julier, S. (2002). The scaled unscented transformation. In
Proceedings of the 2002 American Control Confer-
ence2. IEEE Conference Publications,Vol 6,PP.4555-
4559.
Julier, S., Jeffrey, J., and Uhlmann, K. (2002). Reduced
sigma point filters for the propagation of means and
covariances through nonlinear transformations. In
In Proceedings of the American Control Conference.
IEEE Conference Publications Vol 2, pp. 887-892.
Julier, S. J. and Uhlmann, J. K. (2004). Unscented filtering
and nonlinear estimation. In Proceedings of the IEEE.
IEEE,Vol 92, pp. 401-422.
kyung-min Cho, jeong-hun Jang, and ki-sang Hong (2001).
Adaptive skin-color filter. In Pattern Recognition. El-
sevier, Vol 34,pp. 1067-1073.
Lefebvre, T. and Bruyninckx, H. (2004). Kalman filters for
nonlinear systems: A comparison of performance. In
International Journal of Control. Vol 77,pp.639-653.
Liang-qun, L., Hong-bing, J., and Jun-hui, L. (2005).
The iterated extended kalman particle filter. In Pro-
ceedings International Symposium on Communication
and Information Technologies 2005. IEEE Conference
Publications,Vol 2,pp.1213-1216.
Lipton, A., Fujiyoshi, H., and Patil, R. (1998). Moving tar-
get classification and tracking from real-time video. In
Proceeding of IEEE Workshop Applications of Com-
puter Vision. IEEE Conference Publicationsn,pp. 8-
14.
LIU, Y., Haizho, A., and Guangyou, X. (2001). Moving ob-
ject detection and tracking based on background sub-
traction. In Proceeding of Society of Photo-Optical
Instrument Engineers. Vol. 4554, pp. 62-66.
McIvor, A. M. (2000). Background subtraction tech-
niques. In Proceeding of Image and Vision Comput-
ing. IVCNZ00, Hamilton, New Zealand.
Meyer, D., Denzler, J., and Niemann, H. (1998). Model
based extraction of articulated objects in image se-
quences for gait analysis. In Proceeding of IEEE Int.
Conf. Image Proccessing. IEEE Conference Publica-
tions,Vol 3,pp.78-81.
Phung, S., Chai, D., and Bouzerdoum, A. (2003). Adap-
tive skin segmentation in color images. In Proceed-
ing of IEEE International Conference on Acoustics,
Speech and Signal Processing. IEEE Conference Pub-
lications,Vol. 3, pp. 353-356.
R Van der Merwe, A. D. (2000). The unscented particle
filter. In Advances in Neural Information Processing
Systems.
Rawlings, J. and Bakshi, B. (2006). Particle filtering and
moving horizon estimation. In Computers and Chem-
ical Engineering. Elsevier,Vol 30,pp. 1529-1541.
Ristic, B., Arulampalam, S., and Gordon, N. (2004). Be-
yond the Kalman filter: Particle filters for tracking
applications. Artech House.
Soderstorm, T. (2002). Discrete-time stochastic systems,
in: Advanced Textbooks in Control and Signal Pro-
cessing. Springer.
Sugandi, B., Kim, H., Tan, J. K., and Ishikawa, S. (2009).
A moving object tracking based on color information
employing a particle filter algorithm. In Artificial Life
and Robotics. Springer Japan,Vol 14,pp. 39-42.
Wan, E. and van der Merwe, R. (2001). Chapter 7: The un-
scented kalman filter,. In Kalman Filteringand Neural
Networks, S. Haykin, Ed.,. Wiley Publishing.
Zhiqiang, W., Peng, Z., Deng, X., and Li.Shifeng (2011).
Particle filter object tracking based on multiple cues
fusion. In Advanced in Control Engineering and In-
formation Science. Procedia Engineering,Vol 15, pp.
1461-1465.
Zhonga, Q., Qingqinga, Z., and Tengfeia, G. (2012). Mov-
ing object tracking based on codebook and parti-
cle filter. In International Workshop on Informa-
tion and Electronics Engineering. Procedia Engineer-
ing,Vol 29,pp. 174-178.
HybridIteratedKalmanParticleFilterforObjectTrackingProblems
381