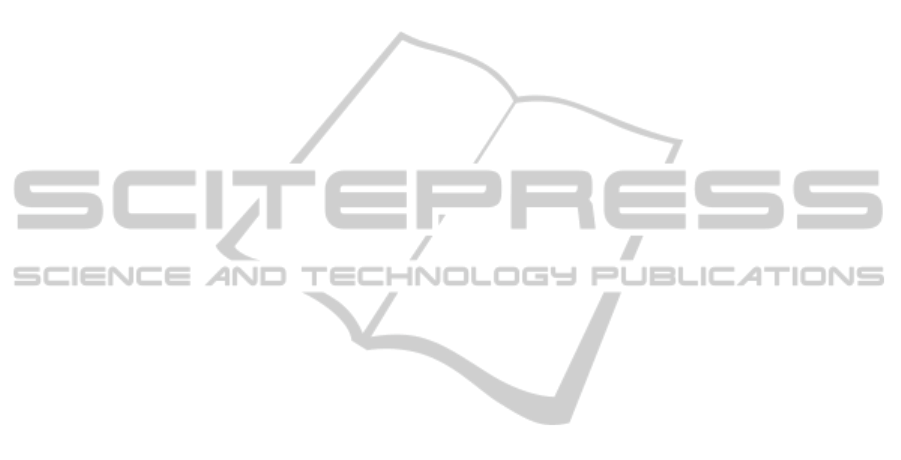
cation method and the bounding ellipse method, it
seems that the fusion parameter worked exactly as
intended; indicating when the Kinect skeleton is re-
liable. If the skeleton match score is low, then the
bounding ellipse has more influence on the classifica-
tion result, and fewer misclassification are made. Our
conclusion is that classification results are indeed im-
proved by data fusion.
We were able to compare the skeleton-based clas-
sification method to an existing bounding ellipse
method. Comparing to other state-of-the-art methods
is more difficult, however, since a lot of these meth-
ods use a specialized sensor setup. Additionally, not
all papers report the full confusion matrix in their re-
sults, or they have non-binary classifiers. This makes
it hard to compare our results quantitavely to other
state-of-the-art methods.
5.1 Future Work
Before a fall detection system is used in practice, it
will have to be able to deal with the challenges of ob-
serving day-to-day life. For example, pets or TVs can
make tracking a person more difficult. The Kinect
proved quite useful for fall detection, but it needs to
be better at tracking targets that sit down or lie on a
couch for the Kinect to be truly reliable in a stand-
alone setup. The limited range and field of view of
the Kinect also make it difficult to apply in Kinect in
some situations. Testing the method on real-life data
instead of simulated falls should also be done; young
people do not fall in the same way as elderly peo-
ple. Unfortunately, not many real-life falls have been
recorded.
5.2 Conclusions
In this research, we have evaluated four fall detection
methods. The first two methods had a high number of
either false positives or false negatives. To combine
data from both methods, we implemented two data
fusion methods. The results show that our data fusion
method B outperforms the other methods, by using
a match score that estimates the reliability of tracked
skeleton. With this we have shown that data fusion
between sensors of different modalities is beneficial
for fall detection.
ACKNOWLEDGEMENTS
This work has been funded by the Foundation Inno-
vation Alliance (SIA - Stichting Innovatie Alliantie),
in the framework of the Balance-IT project.
REFERENCES
Alemdar, H., Yavuz, G.,
¨
Ozen, M., Kara, Y., Incel,
¨
O.,
Akarun, L., and Ersoy, C. (2010). Multi-modal fall
detection within the WeCare framework. In Proceed-
ings of the 9th ACM/IEEE International Conference
on Information Processing in Sensor Networks, pages
436–437. ACM.
Anderson, D., Luke, R., Keller, J., Skubic, M., Rantz, M.,
and Aud, M. (2009). Linguistic summarization of
video for fall detection using voxel person and fuzzy
logic. Computer Vision and Image Understanding,
113(1):80–89.
Brown, D. (1971). Lens distortion for close-range pho-
togrammetry. Photometric Engineering, 37(8):855–
866.
Foroughi, H., Rezvanian, A., and Paziraee, A. (2009). Ro-
bust Fall Detection Using Human Shape and Multi-
class Support Vector Machine. In Computer Vision,
Graphics & Image Processing, 2008. ICVGIP’08.
Sixth Indian Conference on, pages 413–420. IEEE.
Gallagher, B., Corbett, E., Freeman, L., Riddoch-Kennedy,
A., Miller, S., Smith, C., Radensky, L., and Zarrow,
A. (2001). A fall prevention program for the home
environment. Home care provider, 6(5):157–163.
Gillespie, L. (2004). Preventing falls in elderly people. Bmj,
328(7441):653–654.
Kannus, P., Parkkari, J., Koskinen, S., Niemi, S., Palvanen,
M., J
¨
arvinen, M., and Vuori, I. (1999). Fall-induced
injuries and deaths among older adults. JAMA:
the journal of the American Medical Association,
281(20):1895–1899.
Liu, C., Lee, C., and Lin, P. (2010). A fall detection sys-
tem using k-nearest neighbor classifier. Expert Sys-
tems with Applications.
Mastorakis, G. and Makris, D. (2012). Fall detection system
using kinects infrared sensor. Journal of Real-Time
Image Processing, pages 1–12.
Oliver, N., Rosario, B., and Pentland, A. (2000). A bayesian
computer vision system for modeling human inter-
actions. Pattern Analysis and Machine Intelligence,
IEEE Transactions on, 22(8):831–843.
Rougier, C., Auvinet, E., Rousseau, J., Mignotte, M., and
Meunier, J. (2011). Fall detection from depth map
video sequences. Toward Useful Services for Elderly
and People with Disabilities, pages 121–128.
Tao, J., Turjo, M., Wong, M., Wang, M., and Tan, Y. (2005).
Fall incidents detection for intelligent video surveil-
lance. In Information, Communications and Signal
Processing, 2005 Fifth International Conference on,
pages 1590–1594. IEEE.
T
¨
oreyin, B., Dedeo
˘
glu, Y., and C¸ etin, A. (2005). HMM
based falling person detection using both audio and
video. Computer Vision in Human-Computer Interac-
tion, pages 211–220.
Yu, X., Wang, X., Kittipanya-Ngam, P., Eng, H., and
Cheong, L. (2009). Fall Detection and Alert for
Ageing-at-home of Elderly. Ambient Assistive Health
and Wellness Management in the Heart of the City,
pages 209–216.
FusionofColorandDepthCameraDataforRobustFallDetection
613