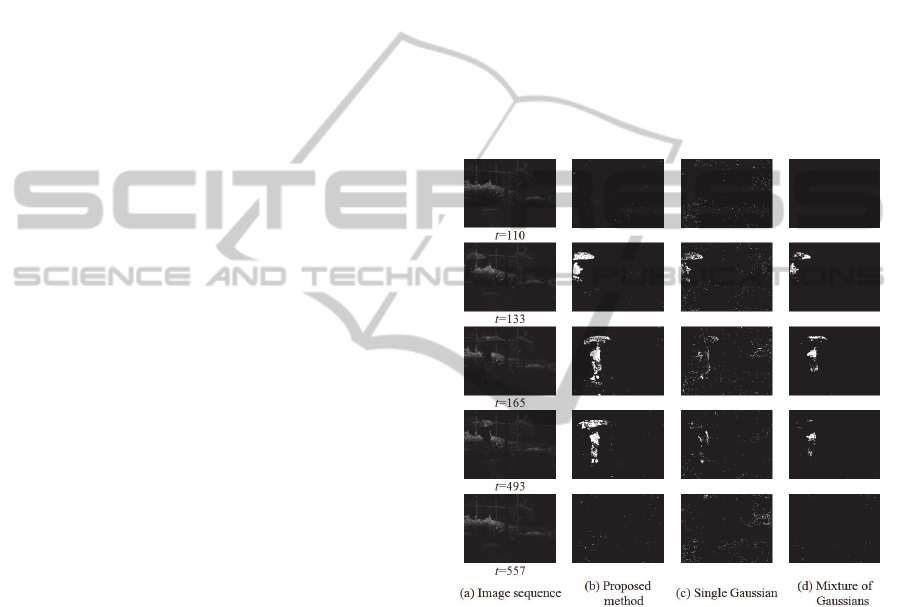
benchmark for comparison. Each sequence shows a
different type of challenge to foreground
segmentation applications. The Wallflower dataset
also provides a hand-segmented ground truth for
evaluation.
Figure 1 shows the comparative results on the
Microsoft benchmark dataset.
The experiment results show that the proposed
method has overall the best performance on the
Microsoft’s Wallflower dataset, as seen in Figure 1.
In terms of the capability for detecting low-contrast
objects, the experimental results of the “Time-of-
Day” video sequence in the Wallflower dataset show
that the proposed dual-mode method significantly
outperforms the GAP method as seen in column (b)
in Figure 1. In terms of computational efficiency, the
GAP method is computationally very expensive. It
takes 25 seconds to process just an image frame of
size 160×120. It can only be used for off-line
applications, such as video retrieval.
The outdoor scenario entails monitoring of a
parking lot, in which a person with an umbrella
passes a clump of bushes and a light pole on a dark,
rainy night. The path is poorly lit and the man is
hardly observable. Rain and wind produce dynamic
changes in the background. The image sequence is
also influenced by the rain in the environment. The
video sequence was filmed at 15 fps. The first
column (a) of Figure 2 shows the original video
sequence at varying time frames. The proposed
foreground segmentation scheme can effectively
extract the person under the very low-contrast
background, as seen in the second column (b) of
Figure 2. The detection results from the single
Gaussian model are presented in the third column (c)
of Figure 2. That model failed to detect the low-
contrast person against the noisy background. The
last column (d) of Figure 5 presents the detection
results from the Gaussian mixture model. This
column shows that the mixture of Gaussians model
was robust to the noise of moving foliage and rain in
the background. However, the foot of the person and
the umbrella were mis-detected, and the shape is not
complete.
4 CONCLUSIONS
In this paper, we have presented a dual-Mode model
for foreground detection from a static camera. It
detects the most frequently occurring gray level of
each pixel, instead of the mean, in the image
sequence. It can quickly respond to changes in
illumination and accurately extract foreground
objects against a low-contrast background. The
variance of gray-level distance between pixel value
and mode indicates the degree of scene changes. The
dual-mode model can handle noise and repetitive
movements such as an opening and closing door and
flashes on a monitor. It can accurately extract the
silhouette of a foreground object in a low-contrast
scene, and it is very responsive to both gradual and
radical changes in the environment. A process frame
rate of 153 fps can be achieved for images of size
160120
on an INTEL Core2 2.53GHz 2046 MB
personal computer. Experimental results have
revealed that the proposed method can be applied to
monitoring of both indoor and outdoor scenarios
with under-exposed environments and low-contrast
foreground objects.
Figure 2: Experimental results of an outdoor parking lot
on a rainy night: (a) discrete scene images in a video
sequence filmed at 15 fps; (b) detected foreground objects
from the proposed method; (c) detection results from the
single Gaussian model; (d) detection results from the
Gaussian mixture model. (The symbol t indicates the
frame number in the sequence).
REFERENCES
Toyama K, Krumm J, Brumitt B, Meyers B. 1999,
Wallflower: Principles and practice of background
maintenance. Inter Conf on Computer Vision 1999:
255-261, Corfu, Greece, September.
Wren, C. R., Azarbayejani, A., Darrell, T., Pentland, A. P.,
1997, Pfinder: real-time tracking of the human body,
IEEE Trans. Pattern Analysis and Machine
VISAPP2013-InternationalConferenceonComputerVisionTheoryandApplications
434