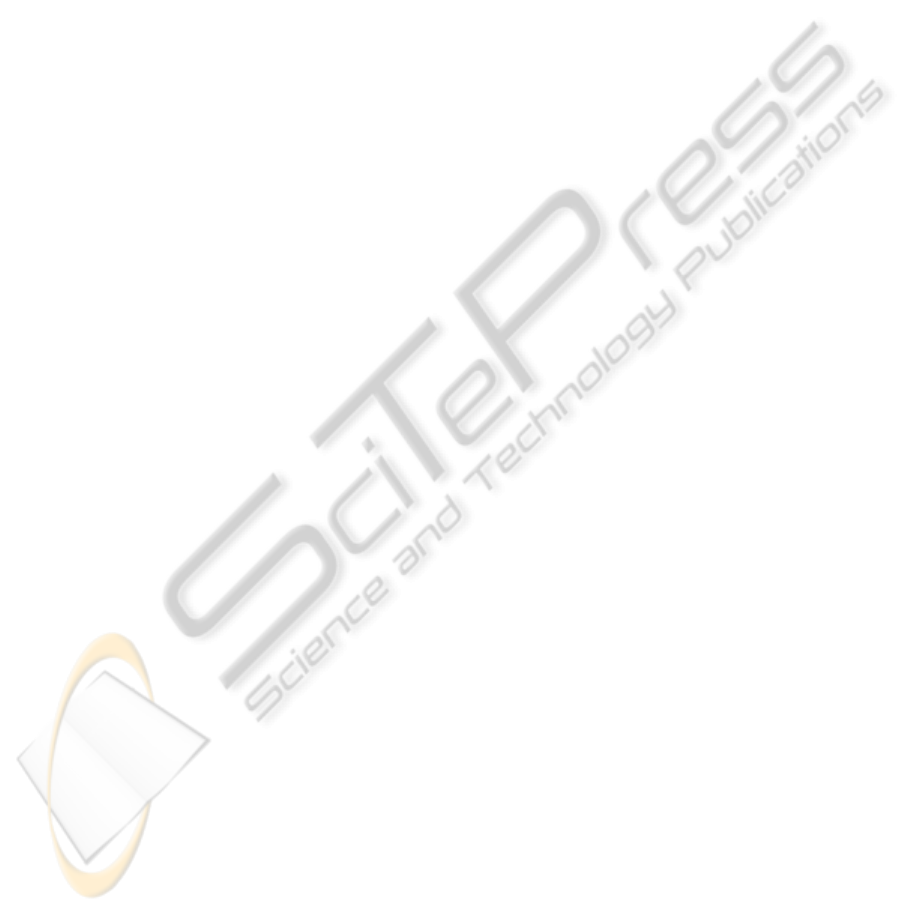
the regression method aims at modeling multiple re-
sponses simultaneously, but it is possible to suggest
the estimates of a factor with different signs, which
causes confusions when one attempts to set up the fac-
tor levels of the experiment. Desirability function is
a compromise method that aggregates responses into
one single quantity, and all factors are set optimally on
this aggregated quantity. Thus, only one set of com-
promise factor setting will be returned and it reduces
the confusion when one attempts to set up the experi-
ment.
Bernoulli-distributed responses, which consists of
only two possible outcomes, is the simplest case for
categorical type variables. One promising direction to
the next step is to develop a framework of desirabil-
ity function approach for categorical responses. It is
interesting to investigate in how to couple the varia-
tion information with the categorical responses when
repeated experiments are done. Since these responses
are not continuous, transformations on the responses
and their variations are required for proper analysis.
Furthermore, the example in this paper has been an-
alyzed and thus comparable. It is desired to perform
more simulations on some new real-life applications
in order to check the efficiency of the generalized
method for categorical responses.
ACKNOWLEDGEMENTS
This work was supported by National Science Coun-
cil of Taiwan ROC grant numbers 100-2118-M-001-
002-MY2 and 101-2811-M-001-001. The authors
would like to thank two referees for their valuable
suggestions and comments to this paper.
REFERENCES
Candes, E. and Tao, T. (2006). The dantzig selector: Statis-
tical estimation when p is much larger than n. Annals
of Statistics, 35:2313–2351.
Derringer, G. (1994). A balancing act: Optimizing a prod-
uct’s properties. Quality Progress, 6:51–58.
Derringer, G. and Suich, R. (1980). Simultaneous optimiza-
tion of several response variables. Journal of Quality
Technology, 12:214–219.
Harrington, E. (1965). The desirability function. Industrial
Quality Control, 4:494–498.
Khuri, A. (1996). Multiresponse surface methodology.
Handbook of Statistics: Design and Anlysis of Exper-
iments (Ghosh, A., Rao C.R. (Eds.)), 13:377–406.
Khuri, A. and Conlon, M. (1981). Simultaneous optimiza-
tion of multiple responses represented by polynomial
regression functions. Technometrics, 23:363–375.
Kim, K. and Lin, D. (2000). Simultaneous optimization
of multiple responses by maximining exponential de-
sirability functions. Journal of the Royal Statistical
Society C, 49:311–325.
Kim, K. and Lin, D. (2006). Optimization of multiple re-
sponses considering both location and dispersion ef-
fects. European Journal of Operational Research,
169:133–145.
Phoa, F. (2012a). The stepwise response refinement
screener (srrs). (in review).
Phoa, F. (2012b). The stepwise response refinement
screener (srrs) and its applications to analysis of
factorial experiments. Proceedings of International
Conference on Pattern Recognition Applications and
Methods (ICPRAM), 1:157–161.
Phoa, F., Pan, Y., and Xu, H. (2009). Analysis of supersat-
urated designs via dantzig selector. Journal of Statis-
tical Planning and Inference, 139:2362–2372.
Pignatiello, J. (1993). Strategies for robust multiresponse
quality engineering. IIE Transactions, 25:5–15.
Tibshirani, R. (1996). Regression shrinkage and selection
via the lasso. Journal of the Royal Statistical Society
B, 58:267–288.
Vande Heyden, Y., Jimidar, M., Hund, E., Niemeijer, N.,
Peeters, R., Smeyers-Verbeke, J., Massart, D., and
Hoogmartens, J. (1999). Determination of system
suitability limits with a robustness test. Journal of
Chromatography A, 845:145–154.
Vining, G. G. (1998). Compromise approach to multire-
sponse optimization. Journal of Quality Technology,
30:309–313.
Xu, K., Lin, D., Tang, L., and Xie, M. (2004). Multi-
response system optimization using a goal attainment
approach. IIE Transactions, 36:433–445.
DesirabilityFunctionApproachontheOptimizationofMultipleBernoulli-distributedResponse
131