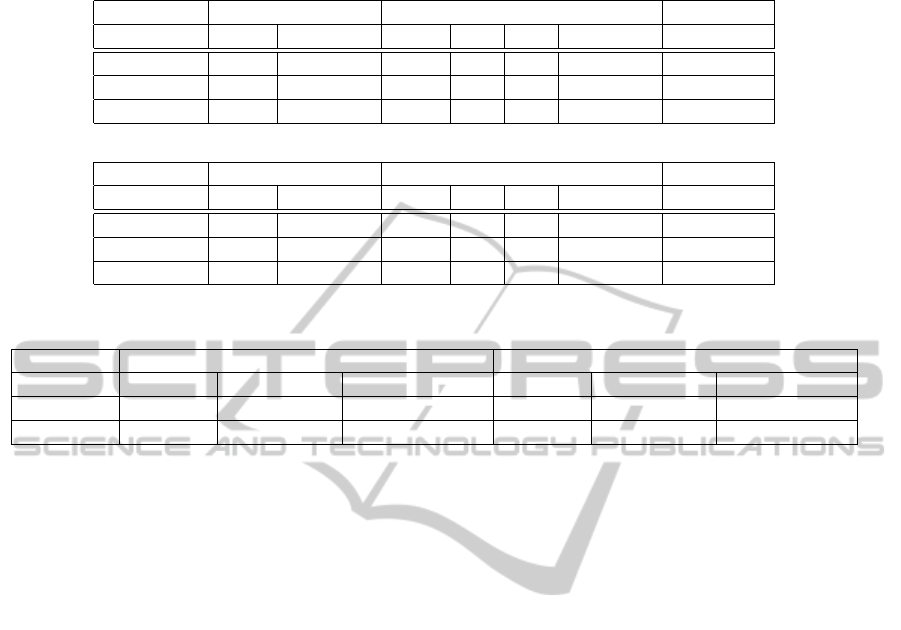
Table 1: Recognition results (%) with the two BoW schemes explored.
(a) Weizmann dataset
k-means Random Projections
Descriptor k Accuracy b L P Accuracy Difference
HOG 2,000 86.02 512 100 200 82.47 3.55
HOF 3,000 91.40 256 200 250 90.25 1.15
HOGHOF 2,000 92.47 2,048 100 250 90.00 2.47
(b) KTH dataset
k-means Random Projections
Descriptor k Accuracy b L P Accuracy Difference
HOG 1,000 83.33 1,024 200 250 70.00 13.33
HOF 1,000 95.37 2,048 100 50 93.51 1.86
HOGHOF 3,000 94.44 2,048 200 200 92.12 2.32
Table 2: Stability results obtained by repeating 20 times each experiment with the same parameters configuration (b, L, P).
Best result Worst result
Mean (µ) Std. dev. (σ) b L P Mean (µ) Std. dev. (σ) b L P
Weizmann 88.50 1.78 8 200 250 80.33 2.22 11 5 50
KTH 91.79 1.73 11 100 50 14.76 4.03 11 25 250
CSD2007-00018, Fundaci Caixa-Castell Bancaixa
(P11A2010-11 and P11B2010-27) and Generalitat
Valenciana (PROMETEO/2010/028).
REFERENCES
Aggarwal, J. and Ryoo, M. (2011). Human activity analy-
sis: A review. ACM CS.
Aloise, D., Deshpande, A., Hansen, P., and Popat, P. (2009).
NP-hardness of euclidean sum-of-squares clustering.
ML.
Bilinski, P. and Bremond, F. (2011). Evaluation of local
descriptors for action recognition in videos. In ICCVS.
Bingham, E. and Mannila, H. (2001). Random projection in
dimensionality reduction: applications to image and
text data. In KDD.
Boutsidis, C., Zouzias, A., and Drineas, P. (2010). Random
Projections for k-means Clustering. NIPS.
Bregonzio, M., Xiang, T., and Gong, S. (2012). Fus-
ing appearance and distribution information of interest
points for action recognition. PM.
Chakraborty, B., Holte, M. B., Moeslund, T. B., and Gon-
zlez, J. (2012). Selective spatio-temporal interest
points. CVIU.
Fern, X. Z. and Brodley, C. E. (2003). Random Projection
for High Dimensional Data Clustering: A Cluster En-
semble Approach. In ICML.
Gorelick, L., Blank, M., Shechtman, E., Irani, M., and
Basri, R. (2007). Actions as Space-Time Shapes.
tPAMI.
Jain, A. K. (2010). Data clustering: 50 years beyond k-
means. PRL.
Johnson, W. and Lindenstrauss, J. (1984). Extensions of
Lipschitz mappings into a Hilbert space. In CMAP.
Kl
¨
aser, A., Marszałek, M., and Schmid, C. (2008). A spatio-
temporal descriptor based on 3d-gradients. In BMVC.
Laptev, I. (2003). On space-time interest points. IJCV.
Li, R. and Zickler, T. (2012). Discriminative virtual views
for cross-view action recognition. In CVPR.
Moosmann, F., Nowak, E., and Jurie, F. (2008). Ran-
domized Clustering Forests for Image Classification.
tPAMI.
Mu, Y., Sun, J., Han, T. X., Cheong, L.-F., and Yan, S.
(2010). Randomized locality sensitive vocabularies
for bag-of-features model. In ECCV.
Natarajan, P., Singh, V. K., and Nevatia, R. (2010). Learn-
ing 3D action models from a few 2D videos for view
invariant action recognition. In CVPR.
Sadanand, S. and Corso, J. J. (2012). Action bank: A high-
level representation of activity in video. In CVPR.
Sch
¨
uldt, C., Laptev, I., and Caputo, B. (2004). Recognizing
human actions: A local SVM approach. In ICPR.
Wang, H., Ullah, M. M., Kl
¨
aser, A., Laptev, I., and Schmid,
C. (2009). Evaluation of local spatio-temporal fea-
tures for action recognition. In BMVC.
Wu, X., Kumar, V., Ross Quinlan, J., Ghosh, J., Yang, Q.,
Motoda, H., McLachlan, G. J., Ng, A., Liu, B., Yu,
P. S., Zhou, Z.-H., Steinbach, M., Hand, D. J., and
Steinberg, D. (2007). Top 10 algorithms in data min-
ing. KAIS.
Bag-of-WordsforActionRecognitionusingRandomProjections-AnExploratoryStudy
619