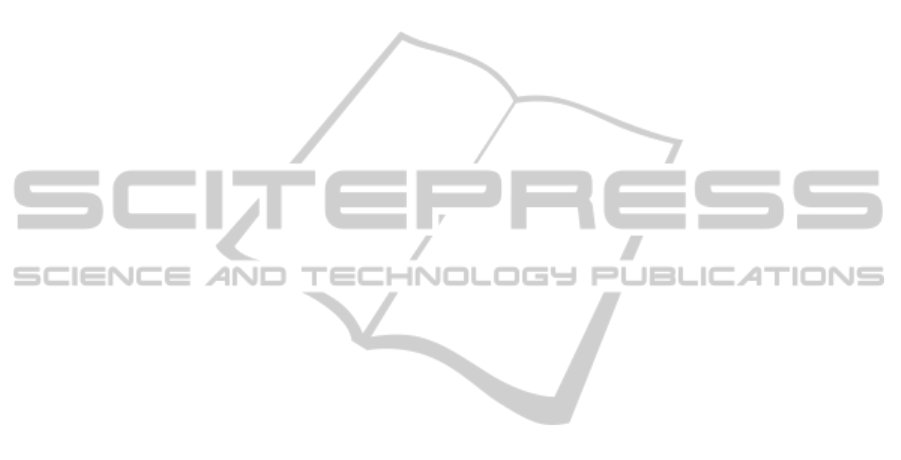
on two public mammogram databases. The
algorithm, inspired by binary search, reached a
sensitivity of 91% with false positive rate per image
of 4.77 in mini-MIAS database and a sensitivity of
87% with a false positive rate per image of 3.67 in
INBreast.
REFERENCES
Eltonsy, N. H., Tourassi, G. D., and Elmaghraby, A. S.
(2007). A concentric morphology model for detection
of masses in mammography. IEEE Transactions
on Medical Imaging, 26(6):880–889.
Gao, X., Wang, Y., Li, X., and Tao, D. (2010).On
combining morphological component analysis and
concentric morphology model for mammographic
mass detection. IEEE transactions on information
technology in biomedicine, 14(2):266–273.
Kom, G., Tiedeu, A., and Kom, M. (2007). Automated
detection of masses in mammograms by local adaptive
thresholding. Computers in Biology and Medicine,
37(1):37–48.
Malagelada, A. O. (2007). Automatic mass segmentation
in mammographic images. Ph.D. dessertation,
Department of Electronics-Computer Science and
Automatic Control, University of Girona.
Martins, L. D. O., Paiva, A. C. D., and Gattass, M. (2009).
Detection of breast masses in mammogram images
using growing neural gas algorithm and ripleys k
function. Journal of Signal Processing Systems,
55:7790.
Mencattini, A. and Salmeri, M. (2011). Breast masses
detection using phase portrait analysis and fuzzy
inference systems. International Journal of Computer
Assisted Radiology and Surgery, page 111.
Moreira, I. C., Amaral, I., Domingues, I., Cardoso, A.,
Cardoso, M. J., and Cardoso, J. S. (2012). INbreast:
toward a full-field digital mammographic database.
Academic radiology, 19(2):236–248.
Nguyen, V. D., Nguyen, D. T., Nguyen, T. D., Thi, N.,
and Tran, D. H. (2010). A program for locating
possible breast masses on mammograms. In
Proceeding of the 3rd International Conference on the
Development of BME in Vietnam, pages 11–14.
Oliveira, M. L. D., Braz, J. G., Cardoso, P., and Gattass,
M. (2009). Detection of masses in digital
mammograms using k-means and support vector
machine. Electronic Letters on Computer Vision and
Image Analysis, 8(2):39-50.
Oliver, A., Freixenet, J., Marti, J., Prez, E., Pont, J.,
Denton, E. R. E., and Zwiggelaar, R. (2010). A review
of automatic mass detection and segmentation in
mammographic images. Medical Image Analysis,
14(2):87–110.
Oliviera, J. d., Albuquerque, A. d., and Deserno, T. M.
(2011). Content-based image retrieval applied to
BIRADS tissue classification in screening
mammography. World Journal of Radiology, 3(1):24-
31.
Petrick, N., Chan, H., Sahiner, B., and Wei, D. (1996). An
adaptive Density-Weighted contrast enhancement
filter for mammographic breast mass detection. IEEE
Transactions on Medical Imaging, 15(1):59–67.
Sampaio, W. B., Diniz, E. M., Silva, A. C., de Paiva, A.
C., and Gattass, M. (2011). Detection of masses in
mammogram images using CNN, geostatistic
functions and SVM. Computers in Biology and
Medicine, 41(8):653-664.
Suckling, J., Parker, J., Dance, D. R., Astley, S., Hutt, I.,
Boggis, C., Ricketts, I., Stamatakis, E., Cerneaz, N.,
Kok, S. L., Taylor, P., Betal, D., and Savage, J.
(1994). The mammographic image analysis society
digital mammogram database. In Exerpta Medica.
International Congress Series 1069, volume 1069,
pages 375–378.
ANewLocalAdaptiveMassDetectionAlgorithminMammograms
137